AI-Powered Patent Review and Analysis - Streamline Your Patent Process with patentreviewpro.com (Get started for free)
7 Practical Applications for a Personal Humanoid Robot in Patent Development and Testing
7 Practical Applications for a Personal Humanoid Robot in Patent Development and Testing - Physical Testing of Patent Prototypes Through Repetitive Motion Analysis
Evaluating the physical performance of a patented humanoid robot design through repetitive motion analysis is fundamental for optimizing its functionality. This process involves subjecting the robot to a series of repeated movements, carefully assessing the efficiency and effectiveness of each action. The gathered data reveals crucial insights into the robot's interaction with its environment and, importantly, with humans. Understanding how the robot's physical structure and control systems respond to these repetitive motions is essential for identifying areas requiring improvement.
Refining the robot's design, be it the mechanics or control algorithms, is a direct outcome of this analysis. The ultimate goal is to ensure the robot fulfills its intended purpose, reliably meeting specified performance standards. Especially as the design of humanoid robots pushes towards more human-like capabilities, bridging the gap between simulated and real-world performance becomes paramount. This is achieved through an ongoing cycle of physical testing and design revisions, allowing for a continuous improvement of the robot's overall utility and applicability across a range of potential uses. In essence, this iterative approach to testing underpins the progress and evolution of the humanoid robot field, gradually enhancing its practical impact.
When developing a physical prototype for a patent, especially in the field of robotics, simply building a working model isn't enough. We need to push the design to its limits, and that often involves subjecting it to repetitive motions. While standard testing might give us a baseline, repetitive motion analysis offers a unique lens into the prototype's behavior.
It can unearth unexpected flaws or failure modes that might not be obvious during standard evaluations. This is because we can precisely quantify the stresses and strains on various materials. We might even anticipate fatigue failures long before they happen, avoiding a surprise breakdown later on.
The magic of using robots for this is the sheer volume of data we can gather. A robot equipped with the right sensors can cycle through millions of motions, giving us a mountain of data in a remarkably short period. We can, in essence, fast-forward through time, watching how the prototype degrades under the onslaught of repeated movements.
Furthermore, we can delve into the prototype's frequency response. This helps us understand how it reacts to vibrations and potentially identify frequencies that could lead to a damaging resonance or unwanted noise. This information is vital for crafting a robot that's stable and operates as intended.
Beyond stress testing, these methods help refine the accuracy of computer simulations we use in design. By comparing the simulation's predictions to the physical robot's responses, we get a better understanding of how our chosen materials truly behave under different conditions. It helps validate our initial assumptions.
It also doesn't stop at just mechanical performance. Environmental factors matter, too. We can investigate how a prototype handles temperature changes, humidity swings, and other aspects that influence real-world operation.
Interestingly, we can use this data to evaluate how comfortable and intuitive a human-robot interaction would be. It's a chance to refine the design from an ergonomic perspective, improving user experience. We can also assess how efficiently a prototype uses power during these repeated tasks, guiding us towards better energy management.
The data itself holds potential for machine learning algorithms. The goal would be to predict maintenance needs, anticipate wear and tear, and even manage the robot's overall lifecycle. Ultimately, having access to this comprehensive dataset is crucial for meeting regulatory requirements, streamlining the patent application process, and preventing costly redesigns at a later stage. It's an example of how robots are not just tools for prototyping, but also agents for advanced testing that makes a difference in creating safer, more efficient future technologies.
7 Practical Applications for a Personal Humanoid Robot in Patent Development and Testing - Collaborative Human Robot Testing Teams for Complex Patent Mechanisms
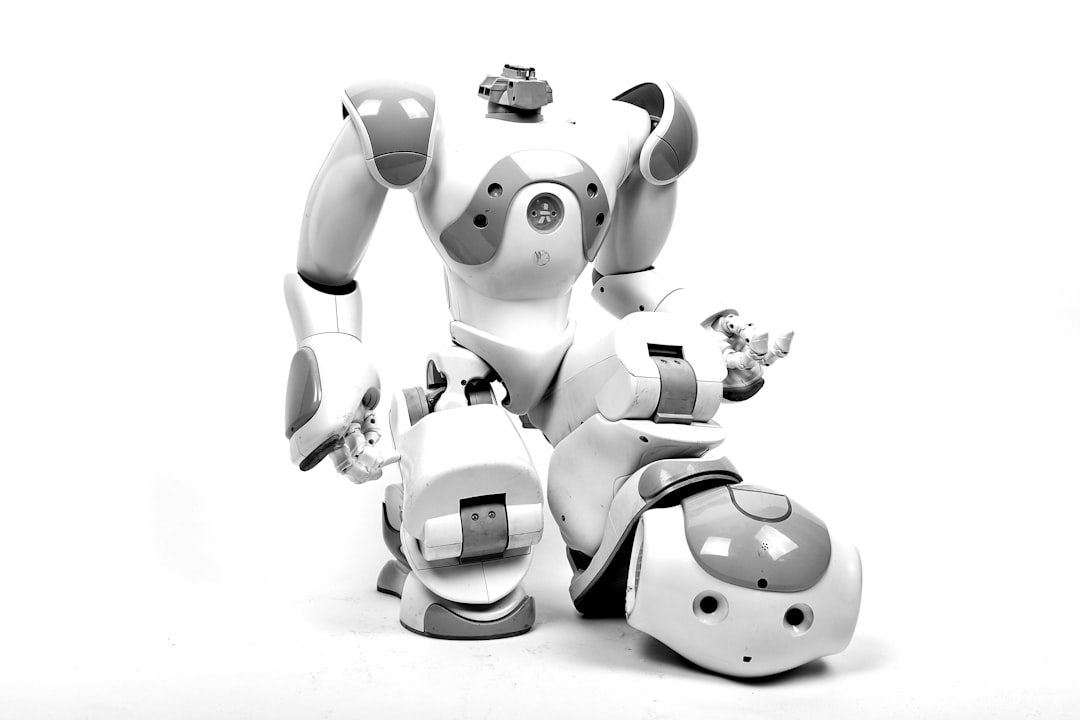
Introducing collaborative human-robot teams for testing complex patent mechanisms represents a significant shift in the approach to patent development and evaluation, especially for robotic systems. Traditionally, testing has often relied on either purely human efforts or automated systems working in isolation. However, the increasing complexity of modern patent mechanisms, particularly in robotics, calls for a more integrated solution.
The concept of using humans and robots in collaborative testing teams leverages the strengths of both. Humans excel at tasks demanding intuition, adaptability, and complex decision-making, while robots offer unmatched precision, stamina, and data collection capabilities in repetitive or high-risk testing scenarios. This type of collaboration could be especially useful when evaluating mechanisms that involve human-robot interactions, like user interfaces or safety protocols.
The development of cobots – collaborative robots designed to interact with humans safely – has paved the way for this new approach. In essence, they can share a workspace without the safety barriers typically needed for traditional industrial robots. Further enhancing the process, digital twins of the patent mechanism can be used to simulate testing scenarios virtually, streamlining the physical testing process and allowing for rapid adjustments to designs. This kind of virtual prototyping coupled with the physical testing enabled by cobots helps in improving both the efficiency and the reliability of the patent testing process.
While still a nascent area, collaborative human-robot testing holds immense potential for enhancing the quality and effectiveness of patent mechanisms, particularly in areas like robotics and automation. It's a promising direction for streamlining and refining the patent development cycle while also potentially revealing new insights into human-machine collaboration that could drive innovation in other areas as well. However, careful attention needs to be paid to addressing potential challenges like effective human-robot communication and data management to ensure the success of this new approach.
Current human-robot interaction is still somewhat limited, but focusing on specific challenges can lead to smoother collaboration. Human-robot teams offer unique benefits from both sides, but achieving effective collaboration can be tricky. Collaborative robots, often called "cobots," are designed to interact physically with humans in shared spaces without the usual protective barriers seen with traditional industrial robots. The idea of using "digital twins" in human-robot teams is being researched, hoping to improve task performance and efficiency.
Allocating tasks in mixed human-robot groups can be improved by using an artificial trust-based approach which learns about each team member's capabilities. The FourByThree project, running since 2014, has been focused on building modular industrial robot systems that allow safe human-robot collaboration in factories. Human-robot collaboration (HRC) is a field of research that pulls together lots of different areas of study. It has a broad range of possible uses and could have a major impact on the economy. A comprehensive study of human-robot collaboration and machine learning showed how important it's becoming in many industrial jobs.
The way collaborative robotics are designed has shifted toward building robots that can easily adapt to working with humans without needing complex programming. A single framework for dynamically changing roles in human-robot collaborative processes has been built to improve efficiency and results in industrial settings.
It's interesting to consider how this applies to patent development and testing, especially for intricate patent mechanisms. A team made up of humans and robots can potentially decrease the time it takes to find flaws in a design, often speeding up the design cycle. Having this fast feedback loop is essential because engineers can adjust their designs faster, based on real data from testing. Advanced sensors in humanoid robots make it possible to gather very detailed data during testing, which can capture even very small changes in motion and stress that might be hard for humans to notice on their own. This precise data is key to refining both the mechanical parts and the control systems.
It's surprising that human-robot collaboration in testing has shown that robots can actually improve human performance. When working alongside a robot, engineers frequently say they are more accurate in jobs that need fine motor skills. This shows robots can be good co-workers rather than just tools. By using machine learning, the data from testing teams can predict not only immediate failures but also long-term maintenance needs, which will change how people think about managing the lifecycle of robots. This means testing can change from fixing things after they break to preventing issues before they happen, which could save a lot of money over time.
It's also interesting to see that using robots to test complex patent mechanisms can help with complying with regulations, by providing concrete proof of safety and performance. Having clear standards from robotic tests strengthens patent applications and could make the approval process smoother. Human-robot teams are especially helpful for figuring out how good the design is for people who will be using it. Robots can simulate different human interactions and point out things about how comfortable and easy to use something is that traditional testing methods might miss. This is important for designs intended for public use or in situations where safety is really important.
Collaborative testing can also reveal how materials react to repeated stress, like changes in stiffness or flexibility over time. Understanding how materials change is vital to making designs that are strong and work properly. The ability of robots to do the same action over and over again without getting tired is attractive to engineers. This lets them test for longer periods than would be practical for humans. With longer testing comes more data points, making the results more reliable and statistically valid. Robots equipped with adaptive systems can change their testing based on what's happening around them, resulting in a testing environment that changes and reacts quickly. This adaptability lets teams look at more situations and stress conditions in an effective way.
It's also worth noting that when engineers and roboticists work together, it's helped lead to successful patent development. They have a more creative approach to problem-solving. This collaboration doesn't just improve testing, it sparks new design ideas, which helps make stronger patent applications. This field of human-robot collaboration holds a lot of promise for making the patent development process more efficient, reliable, and innovative. The continued exploration of how humans and robots can collaborate is important for advancing robotics, patent testing, and many other fields.
(Oct 24, 2024)
7 Practical Applications for a Personal Humanoid Robot in Patent Development and Testing - Patent Safety Feature Validation Using Humanoid Stress Tests
Validating the safety features of a humanoid robot through stress testing is a critical step in ensuring its reliable operation, particularly when working alongside humans. As humanoid robots become more sophisticated and integrated into our lives, these stress tests become increasingly important. They involve pushing the robot's limits by simulating a range of extreme conditions it might experience during its intended use. This process not only reveals potential weaknesses and failure modes but also helps engineers understand how the robot handles those stressors.
The ability to run complex and repetitive tests, often facilitated by automated testing procedures, is vital to refine a robot's safety features and ensure they meet the highest standards. These tests are key for ensuring the robot's safety, particularly in situations where human interaction is paramount. By understanding how a robot responds to stress, we can further enhance its design, refine its safety protocols, and ultimately ensure it functions reliably in real-world applications. The insights gathered from these stress tests are crucial for strengthening the robustness of patent applications in robotics, as they provide concrete evidence of a robot's performance under challenging conditions. In turn, this strengthens the overall patent development process and fuels innovation in the field.
Humanoid robots, with their ability to perform repetitive tasks tirelessly, offer a unique approach to patent safety feature validation. They can accelerate the identification of design flaws that might take years to surface through traditional mechanical testing methods. This accelerated testing is crucial in ensuring the robustness of designs before they reach the market.
The embedded sensors in these robots allow for a level of precision in data capture that often surpasses human capabilities. They can detect subtle variations in material behavior under stress, providing insights into potential mechanical failures. This level of detailed data improves the reliability of predictions about the design's long-term performance, which strengthens patent applications.
Beyond the mechanical aspects, data from these stress tests can offer valuable insights into the ergonomics of human-robot interaction. It's fascinating how we can derive insights about user experience from observing the robot's performance. This opens up the possibility of designs that prioritize user comfort and safety simultaneously.
These tests can often uncover failure modes that are not readily apparent in typical lab environments. A robot might encounter an end-user in a way that was not anticipated, thereby illuminating previously unobserved design vulnerabilities. These unexpected insights are invaluable in enhancing a design's safety profile.
Utilizing machine learning on the large datasets generated from these stress tests allows us to move towards more proactive maintenance strategies. By analyzing the data, we can potentially predict future maintenance needs and potential downtimes. This paradigm shift from reactive to preventative maintenance has the potential to significantly reduce downtime and associated costs.
Another valuable aspect is vibration analysis during these stress tests. Identifying potential resonant frequencies that could compromise the integrity of a design allows engineers to proactively address these issues before they lead to failures. The ability to anticipate and mitigate these vibration-related problems further enhances the safety and reliability of the design.
We can even envision humanoid robots participating in design review sessions alongside engineers. They could be used to demonstrate the safety features within the context of real-world operational scenarios. This could help ensure that the safety measures integrated into the design effectively address the intended use case.
The wealth of data from these stress tests provides engineers with the capability to refine their simulation and modeling techniques. The cyclical feedback between physical testing and simulation allows for continual improvement in the accuracy of the models and optimization of the design process.
It is interesting how this method allows us to validate both the mechanical robustness and user interaction aspects of safety features. This is especially crucial for products intended for public use, where user experience must be intrinsically linked to safety protocols.
Ultimately, the integration of humanoid robots into the patent validation process streamlines the testing phase and encourages a more innovative approach among engineers. This data-driven method can potentially influence the way we think about design and development, leading to safer, more efficient products in the future. It's a testament to how robotics is not only impacting manufacturing but also pushing the boundaries of how we design and evaluate new technologies.
(Oct 24, 2024)
7 Practical Applications for a Personal Humanoid Robot in Patent Development and Testing - Movement Pattern Recording for Patent Documentation Requirements
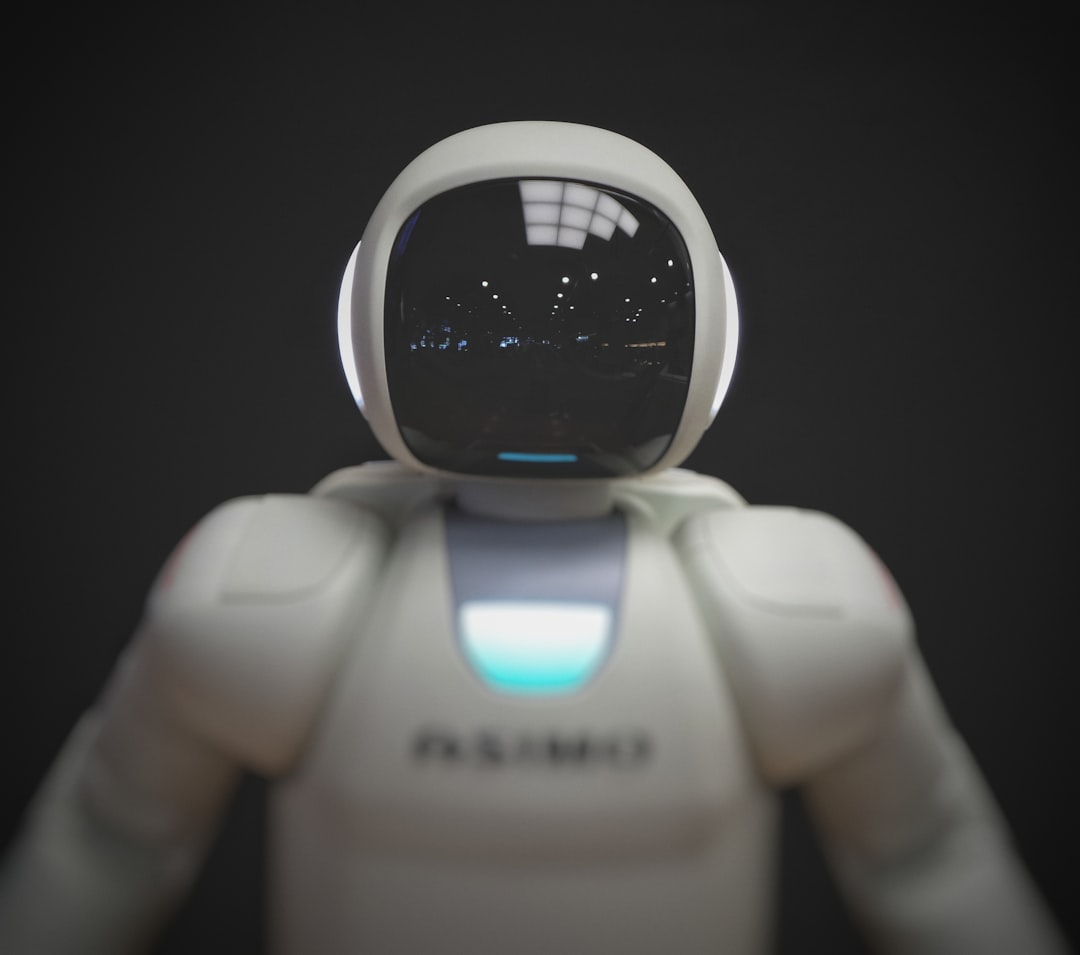
Capturing and documenting a humanoid robot's movement patterns is essential for meeting patent documentation needs. This involves recording how a robot moves and interacts with its environment, which is vital information to include in patent applications, particularly in robotics. By recording and analyzing this movement data, developers can provide robust evidence of how their robot functions and how it might interact with users or objects. This helps substantiate claims in a patent application, as well as reveal any weaknesses in the design before they appear in the real world. It's becoming more important as these robots become more advanced and capable. By rigorously documenting these movement patterns, developers can meet industry standards and enhance the overall quality of their robotic inventions. This thorough approach can streamline the patent process and encourage future advancements in humanoid robot designs.
When it comes to documenting a patent for a humanoid robot, simply describing the intended function isn't enough. We need hard evidence, and that's where capturing and analyzing the robot's movement patterns becomes crucial. This process offers a level of detail often surpassing human observation. Subtle changes in how a robot moves, tiny variations that might escape a human's eye, can be precisely captured with the right sensors. This is particularly important when we consider things like identifying potential points of failure in a robot's joints or mechanisms due to repeated stress over time.
Think of it like this: By examining these movement patterns, we can predict when certain components might fail. Imagine analyzing the way a robot's arm moves during a repetitive task – we might see subtle changes in its motion that signal an impending mechanical failure. This kind of foresight is invaluable for preventative maintenance, a concept that's becoming more important as robots become more prevalent in our lives.
Additionally, we can study the robot's response to vibrations. Some frequencies can cause parts to resonate and potentially lead to damage, just like the way a wine glass can shatter if you hit it with the right tone. Identifying these potential problem areas in advance is critical for ensuring that a robot's design is stable and durable.
Beyond mechanical issues, these movement patterns also provide a new way to think about how a robot interacts with a human. We can use this information to objectively evaluate the comfort and ease of use from a user's perspective, essentially performing a quantitative ergonomic study. It's a subtle but important shift, from just considering how a robot moves to how it moves in relation to humans. This is increasingly crucial for robots intended for use by or around humans.
The collected data also feeds back into our computer models. The insights we gain from observing a robot's physical movements help refine our simulations, ensuring they represent real-world conditions more accurately. This enhanced realism in the simulations is essential for strengthening the claims made in a patent application.
Of course, one of the biggest benefits of capturing movement patterns is speeding up the development cycle. We can use robots to run repetitive tests in a fraction of the time it would take to do the same thing manually. This allows us to quickly identify design flaws and fix them before they become major problems. Think of it as accelerating time – instead of waiting years to discover a flaw through traditional mechanical testing, we can potentially uncover it in a matter of days or weeks.
Moreover, these motion recordings give us a powerful tool for understanding exactly why things fail. It's like having a detailed record of the events leading up to a mechanical failure, making it much easier to identify the root cause. This is crucial for guiding design revisions and ensuring future reliability.
And it doesn't stop there. As the robot moves and collects data, it can learn and adapt its actions in response to observed conditions. This dynamic capability is increasingly important for applications where robots need to work in unpredictable environments, a testament to how robotics is moving beyond predefined tasks.
In essence, having a detailed record of a robot's movements helps us strengthen the claims we make in patent applications. It transforms theoretical arguments into tangible, measurable evidence. It enables us to demonstrate, rather than simply state, that a particular robot design offers advantages in terms of safety, efficiency, or ergonomic design. As humanoid robots become more integrated into our lives, capturing their movement patterns will undoubtedly become an essential part of ensuring that they're both safe and beneficial.
(Oct 24, 2024)
7 Practical Applications for a Personal Humanoid Robot in Patent Development and Testing - Machine Learning Training Data Collection During Patent Development
In the realm of patent development, machine learning is emerging as a powerful tool to enhance data collection and analysis. The increasing complexity of patents, particularly in robotics and AI, requires robust training data for efficient processing. This data allows for the automation of tasks like patent document classification, streamlining the workflow for patent offices and accelerating the filing process.
Building and testing effective machine learning models hinges on the availability of relevant training datasets. Gathering data from specific patent domains becomes crucial for creating reliable training and testing sets, enabling the rigorous evaluation of machine learning model performance. The emergence of dedicated datasets, like those focused on identifying AI elements in vast patent collections, showcases the potential for machine learning to drive innovation within the patent development process.
While machine learning offers significant advantages, it's essential to establish well-defined protocols for data collection and meticulously manage the entire process. The potential benefits are substantial, but the challenges associated with handling intricate data in this growing field must be carefully considered. Achieving informed decisions and ensuring research integrity necessitates a critical approach to data quality and validation throughout the process.
Machine learning is increasingly relevant in patent development, particularly for tasks like classifying patent documents. This reliance on machine learning emphasizes the importance of collecting high-quality training data. A robust dataset is essential for building and refining machine learning models used for patent analysis.
Publicly accessible datasets can accelerate research in patent analysis, but there's a need to develop specialized datasets for particular technology domains, like humanoid robots. Focusing on specific topics helps create targeted training and test datasets to evaluate the performance of machine learning models in that domain.
The process of gathering data for training machine learning models involves clearly defining the problem you want to solve. This includes identifying the kinds of data needed and where to find it. Furthermore, you need to figure out the most effective methods for collecting this data. Data quality is fundamental. Without it, you'll have trouble making smart business decisions, maintaining research quality, and staying on track.
Once the data is collected, it's used to train and tune machine learning models. This development phase involves evaluating the model's accuracy and overall performance. There are interesting examples of this in practice, like the Artificial Intelligence Patent Dataset (AIPD). This dataset, built using machine learning, was used to spot AI mentions across a massive collection of patents and patent applications.
However, we need to be careful when implementing these machine learning models in commercial situations. Failing to think this through might lead to issues with patent filing deadlines, a point we must address.
Understanding how a patent uses data is becoming critical during invention disclosure interviews, especially with the rise of AI and machine learning technologies. It's vital to document how data collection and processing influence the patent's core function. This type of meticulous consideration is likely to become a key aspect of patent development and analysis going forward.
7 Practical Applications for a Personal Humanoid Robot in Patent Development and Testing - Environmental Adaptability Testing for Multi Surface Patents
Within the broader field of personal humanoid robot development, evaluating their ability to function in different environments is increasingly important. This is particularly true when considering patents related to robots that need to operate on various surfaces. Testing how a robot adapts to different terrains, such as rough surfaces, stairs, or even water, is crucial to ensure they function as intended. Using design features like shape-shifting or flexible materials can give them better movement abilities, allowing them to adapt to a greater variety of environments. While this is promising, it raises new concerns. Maintaining these flexible components and ensuring their continued functionality over time poses a challenge that needs further study. Control systems also play a key role. Advanced methods for walking control that can dynamically adjust to environmental feedback are being developed and tested. This mimics how animals and humans are able to adapt to unexpected obstacles or changes in terrain. There is a clear focus on making humanoid robots more versatile, allowing them to easily move from one environment to another. But, this ambition needs to be tempered by ensuring safety and long-term reliability, particularly if these robots are going to interact with humans in various settings.
Environmental adaptability testing is crucial for personal humanoid robots to work well across different surfaces and terrains. This type of testing reveals a lot about how robots might actually behave in the real world, and it's becoming increasingly important as these robots become more sophisticated and more common.
One interesting aspect is that robots can experience quite different friction when moving from one surface to another, such as from wood to tile. This change in friction can make it harder for the robot to maintain balance and move smoothly, especially if the robot isn't designed to anticipate these changes. So, for patents related to multi-surface movement, adaptive control systems are vital, and it's a good idea to include those in the design process.
Then, there's the influence of temperature. The way different materials react to changes in temperature can have a significant impact on the way a robot's components behave. This is particularly relevant when you are thinking about the robot being used in many different environments. A robot designed to be used inside might not work as well outdoors when temperatures change rapidly. This temperature sensitivity needs to be accounted for in the design, and that's where extensive environmental testing can help.
It's also vital to consider the impact of moisture. A robot that moves from a dry surface to a wet surface can experience a large change in performance, potentially leading to slipping and errors. Understanding how moisture affects the material properties of the robot is crucial for designing robots that will operate effectively in different environments, including some that may be wet, like a kitchen or bathroom.
When designing sensors for robots, it's important to understand that the calibration might be different for various materials. A sensor that works flawlessly on one surface might not work the same on another. This shows why it's crucial to perform extensive tests across multiple surfaces during the design phase.
Furthermore, the types of wear and tear a robot will experience can vary based on the surfaces it traverses. Continuous testing can help us predict how long different parts of a robot will last before they break down. This information is crucial for making reliable claims in patent applications.
The way humans interact with robots can also be influenced by the type of surface they're both on. Multi-surface testing is a useful way to see how the ergonomics of the robot might change in different environments, potentially impacting the design in ways that improve the human-robot interaction.
Also, it's not always obvious how robots will react to vibrations. Different surfaces create a unique vibration environment, which can potentially create problems like resonance, if not taken into consideration. Through testing across multiple surfaces, engineers can try to anticipate these problems and address them.
It's also crucial to understand that the weight and speed at which a robot is moving can impact its stability, especially as the environment changes. These dynamic load responses play a significant role in a robot's performance and can guide design choices, particularly in the context of environmental adaptability.
Additionally, using the real-time data from environmental adaptability tests can provide valuable data to train machine learning algorithms. This can help to make future robots more adaptive by teaching them to anticipate and react appropriately to changes in their environment.
Finally, it's important to remember that environmental adaptability testing isn't just an engineering practice but is also becoming a requirement for many regulators. This is particularly important for robots designed for public use. Having patents that specifically cover adaptability and performance across multiple surfaces can make it easier to meet safety standards and get a patent approved, improving the whole process of creating and deploying new robotics technologies.
(Oct 24, 2024)
7 Practical Applications for a Personal Humanoid Robot in Patent Development and Testing - Automated User Experience Testing for Consumer Product Patents
Automated user experience (UX) testing is becoming increasingly important in the development of consumer products, particularly for patent applications. It's designed to augment conventional UX evaluation methods that often rely on time-consuming user studies. Through the use of automated systems, patent developers can quickly identify usability issues and improve the efficiency of product testing, speeding up the overall development process. However, the shift toward automated UX testing also brings with it the need to critically evaluate its effectiveness compared to traditional, human-centered testing approaches. Are the results just as comprehensive? Are the findings equally accurate? These are questions that are at the forefront of patent research and development as this technology progresses. The broader implications of this trend for how people will ultimately accept and use the product remain a crucial element in the patent process and in innovation more generally.
Automated UX testing is emerging as a valuable complement to traditional methods in patent development, especially for consumer products. Traditional methods, like user surveys and workshops, can be time-consuming and hinder the quick design iterations we need in today's fast-paced development cycles. Automated UX testing aims to speed this up.
For instance, we can imagine using a humanoid robot as a test subject, outfitted with sensors that can capture incredibly detailed data. Instead of relying on human observers, the robot can detect very small variations in pressure or other factors, giving us far more precise data on user comfort and interaction. This could lead to a deeper understanding of how users might instinctively interact with a product, sometimes even in ways we hadn't anticipated initially.
One of the benefits is that it can create a quantitative basis for ergonomics, meaning we can translate "feel" into actual, measurable data. We can get precise numbers on things like how far a user has to reach, their posture, and how long they interact with a specific part of a design. This objective data can then be used to refine the design, making it more user-friendly and improving the likelihood of user acceptance.
Further, this automated data collection can feed into machine learning algorithms to potentially predict when a product might need maintenance. This shift towards predictive maintenance could be huge, allowing us to proactively fix issues before they lead to a user problem. For example, it could identify trends in user interactions that show a component may be nearing the end of its life, allowing for preventative repairs. This type of predictive capability could not only enhance the longevity of a product but also give us a unique perspective on its usability over time.
However, we can also utilize the humanoid robot to perform complex tasks. It can simulate how a user might interact with the product in more realistic ways than what we might achieve in a lab setting. It helps document the product's functionality and limitations under practical conditions. That helps make the patent application stronger.
This approach to testing can also be very versatile. For example, it can allow us to expose products to a range of different environments to better understand how they respond under changing conditions. This allows us to quickly spot flaws in design and durability that may be missed in regular testing and significantly benefit product safety and usability in the real world.
This automated testing approach enables us to collect data over long periods of time, unlike typical user tests. That enables a better understanding of how a product performs over time with repeated use. We can start to see a better picture of user experience evolution and how it changes based on continued interaction. And because we are using machines, there is less chance of human bias interfering with the data. That means we have a more unbiased understanding of a design's strengths and weaknesses.
Finally, there's the possibility of a sort of cross-pollination of ideas. The data and insights collected for one patent application might reveal principles that can be applied to completely different product domains. This could help inspire innovative solutions in areas that might not seem related at first glance.
The field of UX testing is definitely evolving, and automated testing is rapidly becoming a significant tool in that evolution. While there are still challenges and limitations to address, the potential of automated UX testing, particularly when we incorporate the use of robots, has the potential to enhance patent development and lead to new insights on product usability and design. It's a fascinating area ripe for ongoing research and development.
(Oct 24, 2024)
AI-Powered Patent Review and Analysis - Streamline Your Patent Process with patentreviewpro.com (Get started for free)
More Posts from patentreviewpro.com: