New Algorithm Enhances Spotify's Music Discovery Patent Pending for AI-Driven Recommendation Engine
New Algorithm Enhances Spotify's Music Discovery Patent Pending for AI-Driven Recommendation Engine - Spotify's New Algorithm Leverages Decision Trees for Refined Music Recommendations
Spotify's new approach to music recommendations centers on using decision trees. This refined algorithm aims to improve the accuracy of recommendations, ultimately leading to a better experience for listeners. The algorithm processes data like a user's listening history and favorite musical styles to create more relevant playlists. This increased personalization is designed to better capture individual tastes and musical preferences. The platform has filed for a patent on this AI-powered recommendation system, indicating a belief in its innovative nature and potential. This development shows Spotify's ongoing commitment to enhancing its ability to suggest music that resonates with users. However, relying solely on algorithms can risk pigeonholing listeners into narrow musical categories. It remains to be seen if this new algorithm can overcome this potential challenge and truly broaden musical horizons while maintaining relevance. The goal is clear: Spotify seeks to ensure recommendations are constantly evolving to match users' ever-changing tastes and broaden its offerings across both music and podcasts.
Spotify's new algorithm employs decision trees, a supervised learning method that breaks down intricate recommendation tasks into a series of simpler, yes/no questions. This allows the system to categorize and recommend music with greater precision based on individual user preferences. Unlike simpler linear models, decision trees can account for more intricate, non-linear connections between user data points. This capability enables Spotify to provide more personalized recommendations, moving beyond broad, generic trends and instead reflecting the subtleties of individual tastes.
The algorithm is designed to analyze various data types, including numerical and categorical, which allows it to take a holistic view of user behavior. This includes aspects like listening history, song characteristics, and potentially even social media activity. It's interesting to see how they are integrating diverse data sources into their music recommendation process. Decision trees also provide a degree of transparency that some other machine learning approaches lack. Visualizing the decision trees helps engineers and researchers grasp how different data features impact recommendations, making the overall process more interpretable.
The algorithm continually learns from user feedback, adjusting as users' music preferences change over time. This means the listening experience is constantly refined, potentially leading to surprising and delightful musical discoveries. An interesting facet of this decision-tree approach is its ability to guard against overfitting. Through techniques such as pruning, the model is less likely to simply memorize past user behavior and instead develops a more robust ability to generalize to new, unobserved data.
It's conceivable that Spotify could further enhance the recommendation accuracy by using an ensemble method like Random Forests. This strategy leverages multiple decision trees to produce a consolidated and hopefully more robust outcome. Spotify's algorithm doesn't just focus on musical similarities but also integrates contextual factors into its recommendations. These factors include time of day and user activity, which makes suggestions more relevant and timely. It's intriguing how contextual awareness is shaping the recommendations.
The system aims to become more perceptive of subtle shifts in user mood or taste through their listening patterns. Ideally, this will allow Spotify to offer tracks that anticipate and align with a user's emotional state. A major advantage of the decision tree approach is its computational efficiency. This efficiency enables the system to process massive datasets in real time, guaranteeing users receive nearly instantaneous updates to their recommendations as their tastes and preferences evolve. This rapid response is a crucial element for keeping users engaged in the platform.
New Algorithm Enhances Spotify's Music Discovery Patent Pending for AI-Driven Recommendation Engine - AI-Driven System Adapts to User's Evolving Music Tastes Over Time
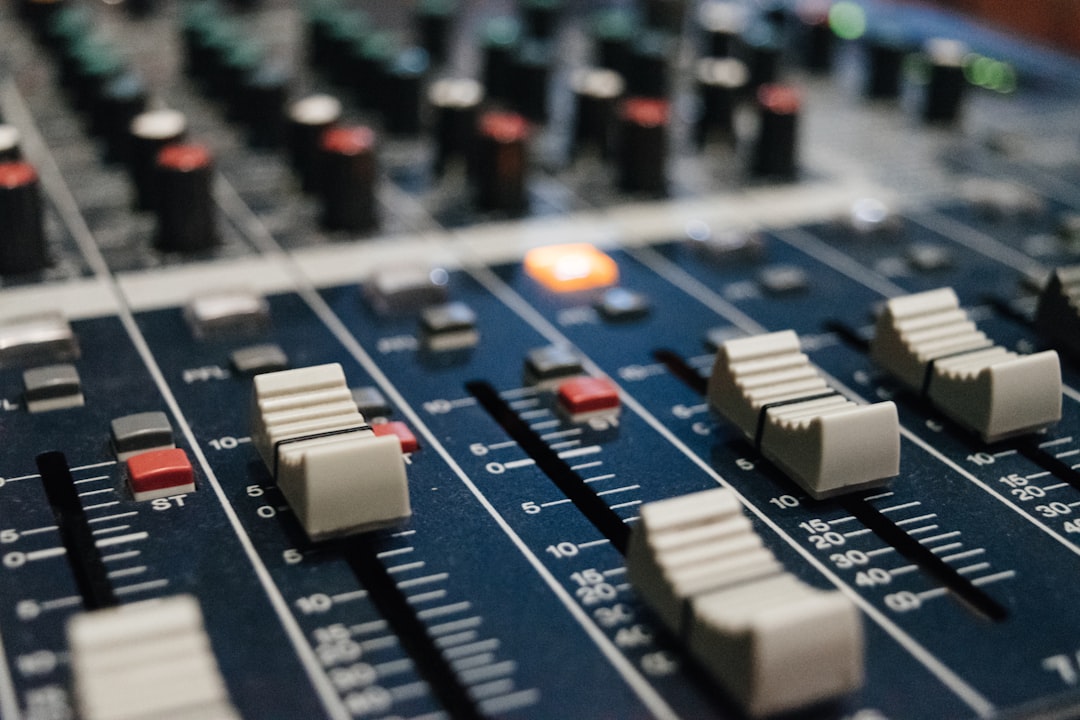
Spotify's patent-pending AI system aims to revolutionize music discovery by adapting to each listener's evolving tastes. This system utilizes a sophisticated algorithm, built around decision trees, to provide more accurate and personalized music recommendations. The algorithm analyzes a wide range of user data, including listening history, preferred genres, and even potentially social media activity, to create a deeper understanding of individual preferences. The algorithm's continuous learning allows it to adjust recommendations as users' tastes shift, ideally leading to a more dynamic and relevant listening experience.
However, relying solely on algorithmic predictions raises concerns about the potential for limiting musical exploration. While personalized playlists can offer convenience and delightful discoveries, there's a risk of users getting stuck in a musical echo chamber. Finding a balance between fostering personalized experiences and encouraging users to venture beyond their usual listening habits will be a crucial aspect of the system's success. The ultimate goal is to provide recommendations that reflect the nuances of individual preferences while also creating opportunities for discovering new genres and artists, keeping the listener's musical journey vibrant and engaging.
It's fascinating how Spotify's algorithm, based on decision trees, can potentially adapt to the dynamic nature of a user's musical journey. For instance, life events like a relationship ending or a new career can significantly alter a person's emotional state and, consequently, their musical preferences. The system could potentially learn to anticipate these shifts, offering more relevant and emotionally resonant recommendations.
Beyond explicit user input, analyzing passive signals like skipped or replayed songs could provide valuable insights into evolving tastes, enhancing the dataset and leading to a richer, more personalized experience. Interestingly, studies show that social circles often influence musical tastes, suggesting that integrating social media data could yield recommendations that align more closely with a user's social environment.
This decision-tree approach not only streamlines complex decision-making processes but also offers the benefit of transparency. Engineers can identify which factors are most crucial in influencing recommendations, allowing for more precise fine-tuning of the system. Furthermore, the algorithm can analyze temporal patterns, generating playlists that adapt to the various phases of a user's day—think upbeat tunes in the morning, mellow vibes in the evening. This temporal adaptation could optimize the overall listening experience, tailoring the soundtrack to the specific moments of a user's daily life.
There's a compelling possibility that the algorithm could steer users towards completely different genres than they typically gravitate towards, essentially challenging the "recommendation bubble" that often arises in streaming platforms. This has the potential to broaden musical horizons and introduce users to new sonic landscapes. Additionally, the model can potentially detect emerging musical trends within the broader user base, spotting new genres or artists that haven't yet gained widespread recognition.
Human music preferences tend to evolve through exposure and context, and the algorithm could potentially replicate this natural process. This is especially important in today's rapidly evolving musical landscape. As the system's accuracy increases, it's plausible that users might find themselves rediscovering older favorites they'd forgotten about, suggesting that nostalgia plays a substantial role in our relationship with music.
Finally, introducing location-specific factors could lead to location-based playlists. Imagine receiving music recommendations tailored to the specific place you're visiting, transforming everyday settings into curated musical experiences. This intriguing possibility suggests a future where music recommendations not only enhance personal listening experiences but also become intertwined with our physical surroundings.
New Algorithm Enhances Spotify's Music Discovery Patent Pending for AI-Driven Recommendation Engine - Patent Reveals Integration of Algorithmic and Editorial Inputs in 'Algotorial' Approach
Spotify has filed a patent outlining a novel approach to music discovery called "Algotorial." This method blends algorithmic suggestions with human editorial input. The aim is to create a music recommendation system that provides more relevant and personalized results compared to purely algorithm-driven suggestions. This "Algotorial" approach attempts to marry the strength of machine learning algorithms in processing user data with the nuanced understanding of music and preferences that human editors possess. It's a response to potential issues with algorithms leading to narrow musical tastes, as they might just cater to past preferences. Spotify believes this hybrid model will lead to an improved user experience and hopefully widen musical horizons for its listeners. This innovation is part of Spotify's ongoing pursuit of making its platform the primary source for discovering and experiencing music and podcasts, and the integration of AI and human curation in this patent could have far-reaching implications for the future of music discovery and artificial intelligence's role in artistic creation.
A recently filed patent reveals Spotify's exploration of a new music discovery approach dubbed "Algotorial." This approach intriguingly blends the strengths of algorithms with the nuanced understanding of human music curators. Essentially, it suggests a way to improve music recommendations by using not just algorithms but also human knowledge about music and how people listen to it.
This novel approach uses a sophisticated algorithm, based on decision trees, that can unravel intricate patterns hidden within user data. This ability surpasses simpler algorithms, leading to more precise and individualized music suggestions. Decision trees are also quite transparent, allowing developers to visually understand how different factors influence the final music recommendations. This transparency helps with refining and debugging the system.
One of the core benefits of this "Algotorial" idea might be to combat a potential drawback of relying solely on algorithms: bias. By including human curation, the system could potentially offer a broader range of genres and lesser-known artists alongside the more popular ones. Instead of just repeating what's already popular, Algotorial hopes to prevent a "musical echo chamber" effect.
Further enhancing the algorithm's adaptability, it can analyze passive listening actions, like skipping songs or replaying them. This helps the system adapt to subtle changes in user tastes without needing constant explicit feedback. The patent also alludes to integrating social aspects of music preferences. This hints at the algorithm possibly using information from social media or other online interactions to better tailor recommendations, since musical tastes are often shaped by the people around us.
This new approach is designed to respond to the context in which a person is listening, suggesting different music depending on the time of day or activity. This means you might get energetic tunes in the morning, and more relaxed choices in the evening. This "contextual music" aspect could make the music experience more enjoyable and relevant. It seems the patent also hints at the algorithm's potential to spot emerging trends in music, exposing users to new artists and genres before they become mainstream. This could lead to a much more vibrant musical discovery journey for users.
Furthermore, the algorithm might use older, potentially forgotten tracks to evoke a sense of nostalgia. This could be a clever way to reintroduce listeners to music they enjoyed in the past, particularly if their musical taste or emotional state has shifted. Lastly, the patent explores the possibility of location-based recommendations, potentially creating musical experiences linked to specific places. For instance, imagine getting a playlist tailored to the city you're visiting, blending music with travel. This suggests future possibilities where music is even more integrated into our lives and experiences.
The Algotorial approach definitely raises intriguing questions about how AI and human expertise can best collaborate in creative fields like music. The ability to adapt to evolving tastes and provide a broader range of recommendations could be a big step forward in how music discovery works, but of course, much depends on how well this approach is developed and implemented in the future.
New Algorithm Enhances Spotify's Music Discovery Patent Pending for AI-Driven Recommendation Engine - Song Categorization Based on Attributes Like Danceability and Energy
Spotify's new algorithm uses a more sophisticated way to categorize songs based on features like how danceable they are and how energetic they sound. By understanding these qualities of songs, the system can get a better grasp of what makes each track unique, ultimately improving personalized music recommendations. Danceability, for instance, examines elements that make a track suitable for dancing, while energy reflects how intense and active a song feels. This detailed analysis allows Spotify to better tailor recommendations to individual musical tastes and potentially expose listeners to a wider variety of music. However, a concern with this approach is that excessive focus on these attributes could inadvertently limit a user's musical journey, leading to a somewhat narrow listening experience. The challenge for Spotify will be to find the right balance between personalization and encouraging exploration of new genres and artists.
Spotify's approach to music discovery involves categorizing songs based on factors like danceability and energy. These classifications are essentially numerical representations of aspects like tempo, rhythm, and intensity. It's quite intriguing how a song's consistent beat, a key factor in danceability, can trigger a psychological inclination towards movement.
The energy of a track can significantly affect a listener's emotional state and drive. Research suggests that energetic music can boost motivation and performance in physical activities. It's not surprising that athletes and fitness enthusiasts find such music valuable during their training sessions.
Interestingly, cultural perspectives on what constitutes "danceable" music differ considerably. For instance, musical rhythms from different cultures elicit distinct responses. This observation highlights the need to consider cultural context when building global music recommendation systems.
While the algorithms are getting increasingly sophisticated, there's always a potential for bias in music categorization. Popular genres can inadvertently dominate the categorization process, possibly hindering the visibility of niche genres and less mainstream artists. This could negatively impact music diversity within the platform.
It's clear that our preference for danceable and energetic music varies not only based on our mood but also on the time of day. Research reveals that energy levels naturally fluctuate throughout the day, typically peaking during periods of heightened activity. This makes creating context-aware recommendations a complex challenge.
Beyond relying on explicit user preferences, Spotify has smartly incorporated para-data such as how frequently a user skips a track. These passive behaviors provide a richer understanding of preferences, offering insights that might be missed with only direct inputs.
The intricate process of categorizing songs extends beyond basic surface-level features. Techniques like MFCCs are used to extract more in-depth musical characteristics. This detailed analysis allows for a deeper understanding of how songs are structurally connected.
The influence of social circles on musical taste is well documented. The recommendation engine could be further improved by analyzing users' social media interactions. This approach, by acknowledging the impact of social environments on musical preferences, could refine the categorization process.
Our musical tastes are constantly evolving, sometimes even revisiting older favorites. This nostalgic element shows the powerful connection we have with music. The algorithms could potentially recognize these patterns, potentially reintroducing forgotten songs to listeners, potentially adding a layer of emotional connection to the recommendations.
Taking cues from the broader context of listening can make the recommendations more relevant. By considering factors like the user's activity (like working out versus studying) or the environment (weather or location), the algorithm can generate playlists that better align with these situations. This enhanced contextual awareness can improve user engagement with the platform.
New Algorithm Enhances Spotify's Music Discovery Patent Pending for AI-Driven Recommendation Engine - Speech Analysis Technology Incorporated to Enhance Music Suggestions
Spotify's latest effort to enhance music recommendations involves incorporating speech analysis technology. This new approach aims to go beyond just analyzing listening history and instead tries to understand a user's emotional state through their voice. The idea is to tailor music suggestions to match the user's current mood, potentially creating a more dynamic and relevant listening experience.
This integration of speech analysis builds upon Spotify's existing AI-driven recommendation engine, but it introduces new challenges and potential concerns. While the goal is to create a more personalized experience, there is a risk that this focus on emotional cues could inadvertently limit musical diversity and lead to users being confined to a more narrow range of music.
The success of this new approach will depend on its ability to strike a balance between delivering personalized recommendations that resonate with a user's emotional state and fostering a sense of musical exploration. By carefully navigating these challenges, Spotify has an opportunity to reshape how we discover and enjoy music in the future, creating a more emotionally connected experience with our listening choices.
Spotify's patent suggests a fascinating direction for music recommendations: incorporating speech analysis. This technology could go beyond simply analyzing listening history and delve into a user's vocal cues, potentially uncovering more nuanced aspects of their musical preferences. For instance, the system could attempt to understand a user's mood or emotional state by analyzing their voice. This could lead to more contextually relevant recommendations, like offering upbeat tunes when a user sounds energetic or calming music when their voice suggests a more relaxed state.
However, there's a potential downside to this approach. Relying on speech for personalization could potentially worsen the "filter bubble" effect where users only hear music similar to what they already like, potentially limiting musical exploration. Striking a balance between providing personalized recommendations and encouraging discovery of new sounds will be key for this technology.
One intriguing area is the ability to detect cultural nuances in how people talk about music. Speech patterns and linguistic cues could provide valuable data for tailoring recommendations to different cultural contexts. For instance, a user from a culture that values rhythmic music might receive more recommendations within that genre based on the way they express their preferences.
Beyond the content of what's said, the way a person speaks—their tone, rhythm, pauses—could also offer insights into their preferences. Maybe a user with a more excited vocal pattern would get energetic tracks, whereas a more contemplative tone could lead to soothing melodies.
It's also promising that this type of technology could offer a more interactive way to refine recommendations. Perhaps if a user vocalizes dissatisfaction with a current track, the algorithm could immediately make a change, leading to a more dynamic music discovery experience.
But a key challenge is how to ensure this new approach doesn't simply reinforce existing biases. For example, if certain music genres are discussed in ways that consistently trigger particular emotional responses, the algorithm might inadvertently overemphasize those genres, hindering the exploration of other musical styles. It'll be critical to develop these algorithms responsibly to ensure they aren't reinforcing pre-existing biases.
Moreover, the ability of algorithms to learn from both text and spoken inputs introduces exciting possibilities. This dual approach could provide a much richer dataset, allowing the system to create even more tailored and relevant music suggestions over time.
Furthermore, voice-driven interactions could enhance the feedback loop between the user and the system. When users discuss their music preferences, the system could analyze those conversations, along with their listening patterns, to understand their evolving tastes more comprehensively. This might lead to more accurate suggestions, bridging the gap between a user's stated preferences and their actual listening habits.
One hope is that this technology could make music discovery more accessible for diverse audiences. By capturing the unique ways different cultures, demographics, and age groups talk about their music, the system might help users discover musical genres and artists they might have otherwise missed.
While still early in its development, the integration of speech analysis into music recommendations presents a compelling opportunity to improve music discovery in several ways. It has the potential to make music more tailored to our individual needs, but it's important to ensure these systems are developed thoughtfully to maximize their benefits while mitigating potential biases and unintended consequences.
New Algorithm Enhances Spotify's Music Discovery Patent Pending for AI-Driven Recommendation Engine - Focus on Personalization and Interactivity for Seamless Audio Content Access
Spotify's latest developments prioritize a more personalized and interactive experience for accessing audio content. This shift is central to improving user engagement and satisfaction. The integration of an AI-powered voice interface intends to create a more customized interaction, impacting how people connect with both music and podcasts. Sophisticated machine learning methods allow the platform to refine recommendations over time, adapting to shifts in user tastes and incorporating factors such as the time of day or even the perceived emotional state of the listener. This attempt to tailor content can be beneficial, but it also carries the risk of creating "echo chambers," where listeners are only presented with music similar to what they've previously liked. This creates a need for a careful balancing act – encouraging exploration of new sounds while still providing relevant recommendations. As Spotify continues to advance its AI-powered recommendations, a key hurdle will be finding a way to stay relevant and anticipate user preferences while also challenging them to move beyond their established listening habits.
Spotify's pursuit of enhancing music discovery extends beyond just analyzing listening history. The platform is now exploring ways to personalize the experience even further by incorporating a user's emotional state and broader context into the recommendation process. This involves analyzing not just what a user listens to but how they express themselves.
One key development is the integration of speech analysis into the AI-driven recommendation engine. This allows the algorithm to potentially grasp emotional cues within a user's voice, like their tone and energy levels. This opens up possibilities for generating music recommendations that align with their perceived mood. Imagine the algorithm sensing a user's tired voice and suggesting a calming playlist, or recognizing an energized voice and offering something more upbeat. However, there's a danger of this approach reinforcing existing musical preferences and potentially limiting exploration.
Beyond mood, this system aims to better account for cultural differences. The way someone speaks about music can carry valuable cues about their cultural background and musical preferences. Someone from a culture that appreciates rhythmic music might receive more suggestions in that genre based on how they talk about music. This highlights the need for careful development to avoid biases in how musical genres are categorized.
Furthermore, the algorithm can adapt to the user's daily rhythms. It can learn that a user might prefer energetic tunes during their morning commute but prefer more soothing music for evening relaxation. This temporal aspect of recommendations seeks to create a more personalized soundtrack for a user's daily life. The system also allows for real-time feedback, so if a user vocally expresses dissatisfaction with a song, the algorithm can respond promptly and generate a new suggestion.
There are still concerns about the potential for personalization to limit musical discovery. It's crucial to find a balance between delivering exactly what a user anticipates and opening them up to a wider array of musical genres. Spotify is attempting to address this by using social media signals as well to provide insight into social circles and musical preferences. This kind of approach might help predict how a user's musical tastes might evolve over time, further enhancing the discovery experience.
Interestingly, the system can leverage nostalgia to reintroduce users to music they haven't heard in a while, potentially creating an emotional connection to their listening experience. It's remarkable how the system can combine multiple data sources to build a richer and more complete picture of user preferences. This holistic understanding of users could pave the way for a future where music isn't just entertainment but becomes a more intricately woven part of our everyday lives and emotional states. This new approach to music discovery presents both incredible opportunities and potential pitfalls, as the technology needs to be carefully designed to avoid fostering limited perspectives.
More Posts from patentreviewpro.com: