Beyond Speed: Key Considerations Before AI Patent Review
Beyond Speed: Key Considerations Before AI Patent Review - Evaluating Compliance with Current Regulatory Guidance
The dynamic regulatory climate surrounding artificial intelligence is making the evaluation of adherence to prevailing guidance increasingly vital. Introducing AI systems brings distinct challenges, requiring robust governance frameworks equipped to handle both long-standing rules and the array of new regulations emerging specifically for AI applications. Companies face the task of not only meeting traditional compliance benchmarks but also actively adjusting to developing AI-specific mandates. This complex reality underscores the critical need for proactive risk management. While the potential for AI to assist in streamlining compliance processes is discussed, promising greater efficiency and accuracy, the speed at which the regulatory landscape shifts necessitates ongoing careful monitoring and flexible adaptation. It's crucial to maintain a critical perspective, recognizing that deploying AI for compliance doesn't remove the fundamental requirement for thorough oversight and a deep grasp of the regulations themselves.
Considering artificial intelligence systems during patent review adds layers of complexity, not least when trying to evaluate their alignment with current regulatory guidance. It’s less about checking off a static list and more about grappling with dynamic, sometimes elusive, challenges.
First, assessing how a claimed AI system adheres to regulations, particularly concerning data usage or fairness, is difficult due to the inherent complexity and opacity of many models. Proving definitively within a patent disclosure that an AI *never* operated in a way that violates privacy rules or fairness standards can feel like trying to prove a negative, raising tricky questions about enablement and the scope of the invention as described.
Second, the regulatory landscape for AI is still actively forming and shifting globally. Guidance deemed sufficient today could be obsolete tomorrow as governments establish new rules around bias, accountability, or specific applications. This means an AI system that appears compliant when a patent is filed might later face legal challenges under evolving standards, complicating the long-term value and utility of the claimed invention.
Third, many proposed AI systems include or rely upon tools designed to monitor or mitigate issues like bias or non-compliance. A critical eye is needed because these evaluation tools themselves might carry their own biases or limitations, meaning assessing the compliance of the *claimed* system requires scrutinizing the validity and potential flaws of the *methods used to evaluate* it.
Fourth, artificial intelligence inventions are often intended for use across international borders, yet regulatory approaches to data, ethics, and acceptable AI behavior vary significantly by jurisdiction. Evaluating whether a claimed system or method conflicts with established (or emerging) principles in major markets presents a puzzle for patentability, as differences in privacy law or algorithmic fairness requirements can impact public policy considerations relevant to examination.
Finally, for AI systems designed to learn and adapt over time, ensuring continuous regulatory compliance is a significant challenge. The behavior of a self-improving AI might drift away from its initial parameters in ways not anticipated or validated, potentially leading to non-compliant operations. For patent review, this raises questions about whether the claims adequately capture and enable a system whose compliant state might not be static but requires ongoing monitoring and correction.
Beyond Speed: Key Considerations Before AI Patent Review - Assessing Data Quality and Confidentiality Risks
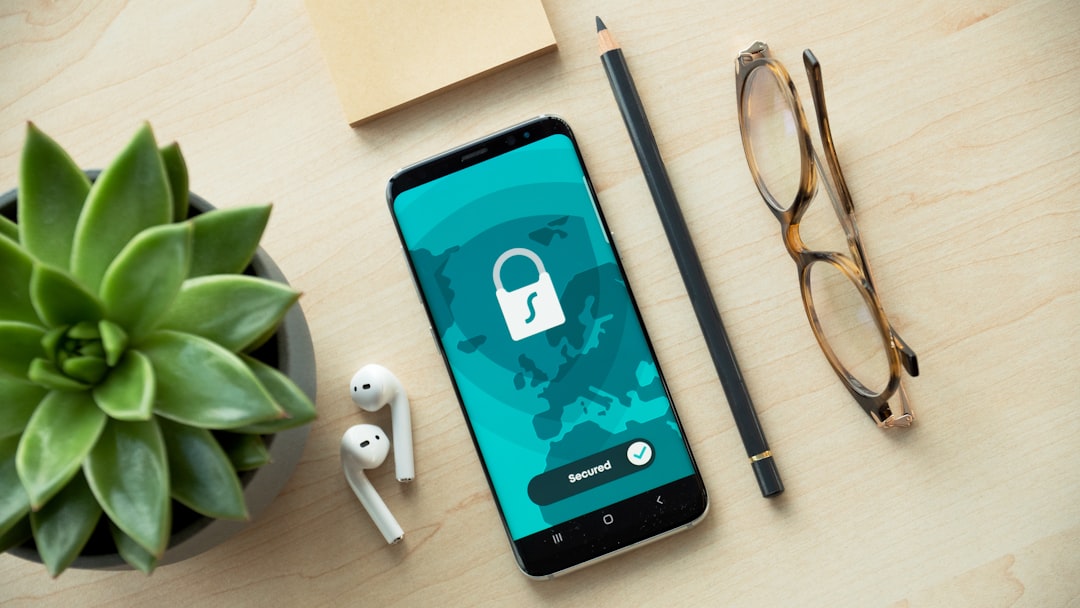
Before employing artificial intelligence in tasks like patent review, it's essential to rigorously examine the data being used, focusing particularly on its quality and potential confidentiality issues. The utility and trustworthiness of any AI system fundamentally depend on the accuracy, completeness, and relevance of its input data. Using poor, outdated, or incomplete data can easily lead to unreliable, biased, or even misleading outcomes from the AI, undermining its purpose. Furthermore, handling sensitive information is inherent in processes like patent review. Deploying AI introduces new vectors for confidentiality risks, demanding robust measures and continuous vigilance to protect proprietary or personal data processed by the system. Organisations must identify and actively manage these data-centric risks from the outset, understanding that the integrity of the process and the reliability of the AI's contribution relies heavily on addressing these data fundamentals effectively and responsibly.
When considering AI systems during patent review, examining data quality and potential confidentiality pitfalls is paramount, and frankly, often more intricate than it first appears.
For one, AI models, particularly complex ones, seem to possess an unsettling ability to amplify any existing flaws in their training data. Trivial inconsistencies, missing values, or outdated entries in the input can propagate through intricate model architectures and dramatically distort the final outputs or predictions. Ensuring data quality isn't just about having data; it's about rigorously validating its integrity before ever feeding it to a model, as rectifying errors post-training is far more difficult.
While techniques like differential privacy are promoted as safeguards for data confidentiality, providing mathematical guarantees against re-identification, the reality is less absolute. Ongoing research continues to demonstrate sophisticated adversarial attacks that can, in certain circumstances, circumvent these protections and potentially link seemingly anonymized records back to individuals. Relying solely on one privacy-preserving technique without continuous evaluation against evolving threats feels risky.
There's also an inherent, often uncomfortable, tension when trying to balance data quality, confidentiality goals, and the ultimate utility of the resulting AI model. Methods designed to aggressively protect privacy, such as heavy data anonymization or aggregation, can strip away crucial granularity or patterns needed to train a robust and accurate model for its intended task. Achieving strong privacy often comes at the cost of diminished data richness, forcing difficult compromises.
Furthermore, regulatory calls for a 'right to explanation' regarding AI decisions clash significantly with the operational reality of many powerful AI systems, particularly deep neural networks. Their sheer complexity makes interpreting *why* a specific decision was reached incredibly challenging. There's a clear tension between deploying high-performing, opaque models and providing the transparency needed to satisfy these explanation requirements, leaving a gap in understanding and potentially accountability.
Even seemingly straightforward anonymization methods, like geographically masking location data by generalizing specific points to broader areas, are vulnerable. Researchers have shown that combining such masked data with other readily available public datasets can often allow for the re-identification of individuals or infer sensitive locations. This highlights how challenging it is to achieve truly effective data anonymity in a world of linked information.
Beyond Speed: Key Considerations Before AI Patent Review - Defining the Essential Human Oversight Role
Establishing the necessary human oversight when integrating artificial intelligence into processes like patent review is fundamental. As AI systems become more deeply embedded, ensuring their effective use necessitates a role for humans that goes beyond simply monitoring output or troubleshooting technical glitches. It requires individuals with a critical understanding of the underlying principles and context, capable of applying judgment that AI currently cannot replicate. This involves navigating complex legal interpretations, ethical considerations, and the potential societal implications of AI-driven decisions. While AI can process vast amounts of information rapidly, discerning nuances, assessing intent, and making value-laden judgments remains squarely within the human domain. The integration should focus on how AI can augment human expertise, rather than aiming for full automation, acknowledging the inherent limitations of current AI in areas demanding abstract reasoning, ethical awareness, and contextual understanding. Defining clear boundaries for AI's capabilities and ensuring meaningful human review points are crucial to maintain the integrity and trustworthiness of the patent review process in an era of increasing automation.
Examining the necessary human element in AI-assisted tasks like patent review uncovers some perhaps unexpected dynamics. It turns out that simply inserting a human into the loop isn't a guaranteed solution for mitigating AI's potential pitfalls; the *nature* of that human involvement matters considerably.
One significant observation is the susceptibility of human reviewers to what's sometimes termed 'automation bias.' When presented with an AI's output, humans can have a strong inclination to accept it uncritically, even if incorrect. This isn't necessarily a failing of the human, but rather a complex cognitive interaction where perceived AI efficiency or authority overrides independent scrutiny. Effective oversight, therefore, isn't just about having a human look at the result, but structuring the workflow and potentially providing counter-check mechanisms to actively encourage skepticism and verification against specific criteria or audit trails.
Furthermore, while deep domain expertise remains fundamental, insights suggest that continuous training focused specifically on the AI system itself – understanding its capabilities, limitations, and how its outputs should be interpreted or challenged – is arguably more impactful for effective oversight than solely relying on a human's static, pre-existing knowledge base. The human reviewer needs to evolve alongside the AI's evolving capabilities and failure modes.
Thinking about the structure of oversight, evidence points towards the value of collaborative approaches. Relying on a single individual to catch every nuance or error in complex AI outputs can be less effective than leveraging a team with diverse perspectives – perhaps combining technical AI understanding with legal expertise and specific subject matter knowledge – and establishing clear roles and processes for layered review. A lone human checker, no matter how skilled, faces inherent limits when reviewing complex AI-generated results.
There's also an intriguing psychological dynamic observed: higher perceived reliability or trustworthiness of the AI system by the human overseer doesn't necessarily correlate with *increased* vigilance. In fact, the opposite can occur – humans may become *less* diligent in their checks when they have high confidence in the AI. This points to a counter-intuitive need for deliberately embedding friction or mandatory verification steps to prevent complacency, regardless of the human's subjective trust level in the machine.
Finally, focusing solely on efficiency metrics like "time saved per review" when evaluating AI-assisted processes can be misleading. While AI undoubtedly accelerates certain steps, this speed doesn't automatically translate into fewer errors identified or corrected. There's a risk that the push for throughput might lead human reviewers to overlook more subtle or complex issues they might have spotted at a slower, manual pace. True effectiveness needs to be measured not just by speed, but by the quality and accuracy of the final outcome after human intervention.
Beyond Speed: Key Considerations Before AI Patent Review - Understanding AI Tool Analytical Capabilities and Limitations
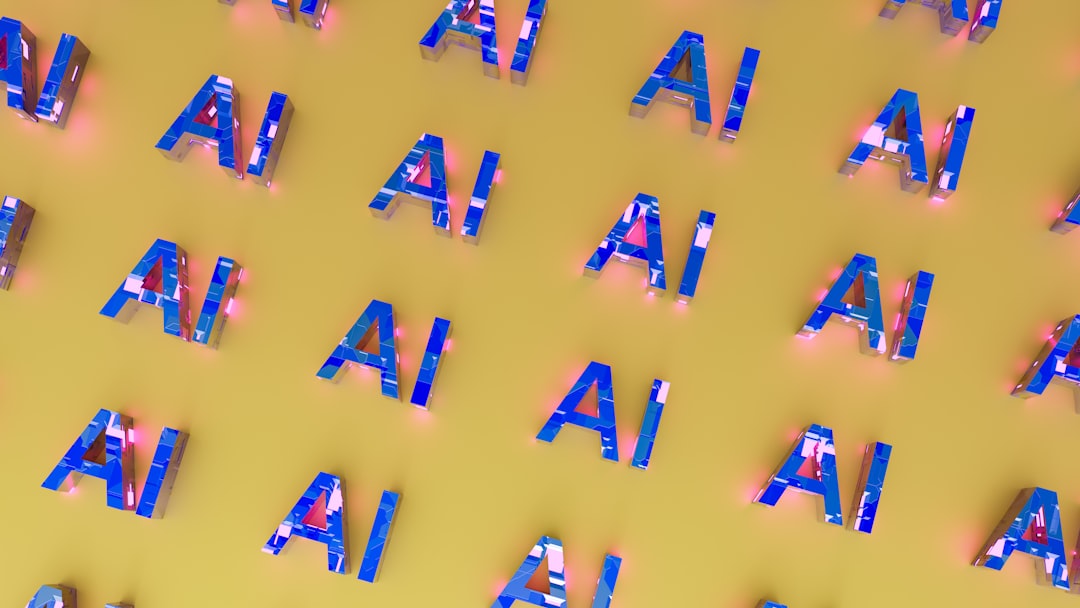
Stepping past the headline speed of artificial intelligence, a deeper dive into its analytical core is necessary. This involves scrutinizing precisely what kinds of insights AI tools are genuinely equipped to produce and, crucially, where their current methods inherently fall short when faced with the specific demands of tasks like patent review. It's about understanding the nature of the 'intelligence' at play, not just its output volume.
Delving into the analytical functionalities offered by artificial intelligence tools for fields like patent review reveals a blend of impressive capabilities and fundamental constraints that warrant close examination. While these systems can process information volumes and identify patterns far beyond human capacity, relying on them blindly without appreciating where their current analytical power falters would be ill-advised. As of mid-2025, here are a few points that highlight where the rubber meets the road regarding AI's analytical limits in this complex domain:
1. One persistent hurdle is the AI's difficulty in grasping the often subtle, context-dependent, and sometimes implied meaning within patent claims. Although statistically adept at finding keyword matches or structural similarities in text, the deep conceptual understanding needed to truly distinguish novel subject matter or pinpoint precise scope, akin to human interpretation informed by years of legal and technical background, remains largely elusive, leading to potentially misleading analytical outputs regarding relevance or novelty.
2. The inherent biases present in the vast datasets used to train these analytical models inevitably color their assessments. If historical patent data over-represents certain technologies, inventors, or even linguistic styles, the AI's analytical framework can implicitly favor or penalize newer or differently structured inventions, potentially skewing automated evaluations of validity or value based on embedded historical inequities rather than objective technical merit.
3. Sophisticated analytical techniques, particularly those involving complex feature extraction or correlation analysis, can inadvertently reveal sensitive or proprietary information through their outputs, even when operating on ostensibly anonymized data. The very process of identifying nuanced patterns can sometimes act as a pathway for inferring details about specific documents or inventors, a subtle data security risk tied directly to the analytical horsepower being applied.
4. Despite the development of "explainable AI" methods, the insights they provide into complex model decisions often fall short of true, human-intelligible reasoning. The explanations might highlight correlative factors or input features, but they frequently fail to illuminate the underlying, often opaque, decision-making logic within deep neural networks, leaving users with an incomplete or potentially misleading understanding of *why* a specific analytical conclusion was reached.
5. Current AI models, while proficient with general language, demonstrably struggle when tasked with analyzing the highly specific, technical jargon and unique syntactical structures prevalent in certain niche technological areas or within legal texts like patent claims. Their analytical precision can degrade significantly when encountering vocabulary or phrasing not adequately represented in their general training corpus, limiting their reliability when diving deep into specialized domains.
Beyond Speed: Key Considerations Before AI Patent Review - Identifying Necessary Practitioner Training Requirements
As AI systems become integral to complex tasks like patent review, the training required for the practitioners who will interact with them takes on new, urgent dimensions. It's not simply about familiarizing staff with new software interfaces; the critical need now is to cultivate sophisticated understanding and specific practical skills that acknowledge the unique characteristics and inherent limitations of current AI technology. Preparing practitioners effectively means addressing how to interpret potentially opaque AI outputs, how to navigate situations where AI might exhibit bias or handle nuanced legal language imperfectly, and how to exercise robust, independent judgment that complements, rather than defers to, machine analysis. The focus must be on developing a deeper, critical technical literacy married with the unchanging demands of rigorous patent practice.
Examining the skills and knowledge required for practitioners working alongside artificial intelligence in areas like patent review uncovers some perhaps unexpected priorities regarding training. It turns out the focus isn't necessarily on turning legal or technical experts into AI developers, but rather equipping them to be critical evaluators and astute collaborators with the machine.
1. It appears that deep coding or data science skills aren't the primary bottleneck for effective AI-assisted patent review. The more critical training emphasis seems to be on sharpening the practitioner's foundational domain expertise (legal and technical subject matter) and refining their capacity for critical thought and judgment to accurately interpret, validate, and challenge the insights generated by the AI system.
2. Insights suggest that robust training programs for this field are increasingly incorporating 'adversarial' exercises. These involve presenting practitioners with scenarios deliberately designed to test the limits of AI tools – perhaps complex prior art drafted to be hard for algorithms to find, or claims worded to expose weaknesses in automated analysis – to build resilience against potential AI failure modes.
3. Observations highlight the necessity for training that delves into the human side of the human-AI partnership. This includes modules specifically addressing cognitive biases like automation bias (the tendency to over-trust automated output) or algorithm aversion (undue skepticism). Understanding these psychological dynamics helps practitioners calibrate their trust and interaction with the AI appropriately.
4. Given the constant evolution of technology, patent law, and the AI models themselves, the evidence points strongly away from viewing practitioner training as a single event. Instead, it functions more effectively as a continuous process, with ongoing education, updates, and practical refreshers needed to ensure skills remain sharp and relevant alongside the rapidly changing tools and landscape.
5. A notable shift in training philosophy appears to be prioritizing an understanding of the AI system's context and limitations – things like data provenance, potential embedded biases, and the specific failure modes of different model types – over detailed knowledge of the underlying algorithms themselves. This approach empowers practitioners to evaluate the trustworthiness of an AI output even if the internal 'why' remains opaque within a black box system.
More Posts from patentreviewpro.com: