AI-Powered Patent Review and Analysis - Streamline Your Patent Process with patentreviewpro.com (Get started for free)
7 Legal Safeguards for Protecting Your AI-Related Intellectual Property Through Non-Disclosure Agreements
7 Legal Safeguards for Protecting Your AI-Related Intellectual Property Through Non-Disclosure Agreements - Defining Core Trade Secrets in AI Model Training Data and Outputs
Protecting the core secrets underpinning your AI models—both the data used to train them and the outputs they generate—is becoming more vital as the field grows more competitive. The Defend Trade Secrets Act offers a helpful, broad definition of what constitutes a trade secret, which is beneficial for companies aiming to safeguard unique datasets and the specialized processes used to build their AI systems. This broad definition allows companies to protect valuable information that gives them a competitive edge.
However, in this evolving world of AI, particularly with the rise of generative AI systems that create truly novel outputs, deciding exactly what qualifies as a trade secret can be difficult. The legal landscape around AI's intellectual property is still being defined, so there's a degree of uncertainty surrounding what protections are available. This makes it crucial for businesses to create strong safeguards, such as non-disclosure agreements, to protect their sensitive information and proprietary innovations. Striking the right balance between these protections and other legal frameworks is key to maintaining a leading position in the rapidly changing field of AI.
The notion of what constitutes a crucial trade secret within the training and output of AI models can vary greatly depending on the specific industry. For instance, a core secret in the healthcare sector might be vastly different from one in finance, emphasizing the importance of tailoring protection strategies to the unique circumstances. Unlike patents, which necessitate public disclosure, trade secrets empower organizations to keep their foundational algorithms and datasets private. This secrecy offers a more flexible avenue for shielding competitive advantages, making them a compelling tool for maintaining a leading edge.
However, safeguarding trade secrets comes with certain stipulations. To secure legal protection, companies must actively strive to maintain confidentiality. Falling short on these measures can result in the loss of trade secret status, regardless of the information's inherent worth. It's not just the training data that can be a trade secret. Outputs from AI models, like unique patterns or insights gleaned during the learning process, can be equally critical. These could provide insights that aren't directly obvious in the training data itself, yet are crucial for holding a competitive position.
The issue of "reverse engineering" within the context of trade secrets requires careful consideration. While analyzing the outputs of an AI model might be permissible, attempting to dissect the model's inner workings could potentially breach trade secret protection and lead to legal complications. This area is becoming increasingly relevant as we increasingly rely on third-party services, especially cloud providers, for AI development. Companies must be mindful of how these vendors handle potentially sensitive data, emphasizing the need for strict provisions within non-disclosure agreements to protect against unauthorized access.
Trade secret protection offers a potentially lasting advantage—it persists as long as the information remains confidential, allowing businesses to rely on this approach for as long as it delivers a competitive edge. Unlike patents, there's no need for periodic renewal or re-filing. But establishing a successful trade secret claim typically necessitates proving that reasonable measures were taken to ensure secrecy. This underscores the vital role of internal policies and comprehensive employee training programs in establishing robust data protection strategies.
Courts often consider the economic value of a trade secret in light of how its disclosure would affect the holder's competitive standing. This adds another layer of complexity to the legal landscape surrounding AI-generated outputs. This ongoing interplay between artificial intelligence and trade secrets is creating a dynamic situation where jurisdictions are grappling with how to best foster innovation while concurrently safeguarding proprietary information. This balancing act leaves the future of related legal structures uncertain and ripe for further exploration and evolution.
7 Legal Safeguards for Protecting Your AI-Related Intellectual Property Through Non-Disclosure Agreements - Protecting Machine Learning Algorithms Through Specific Confidentiality Terms
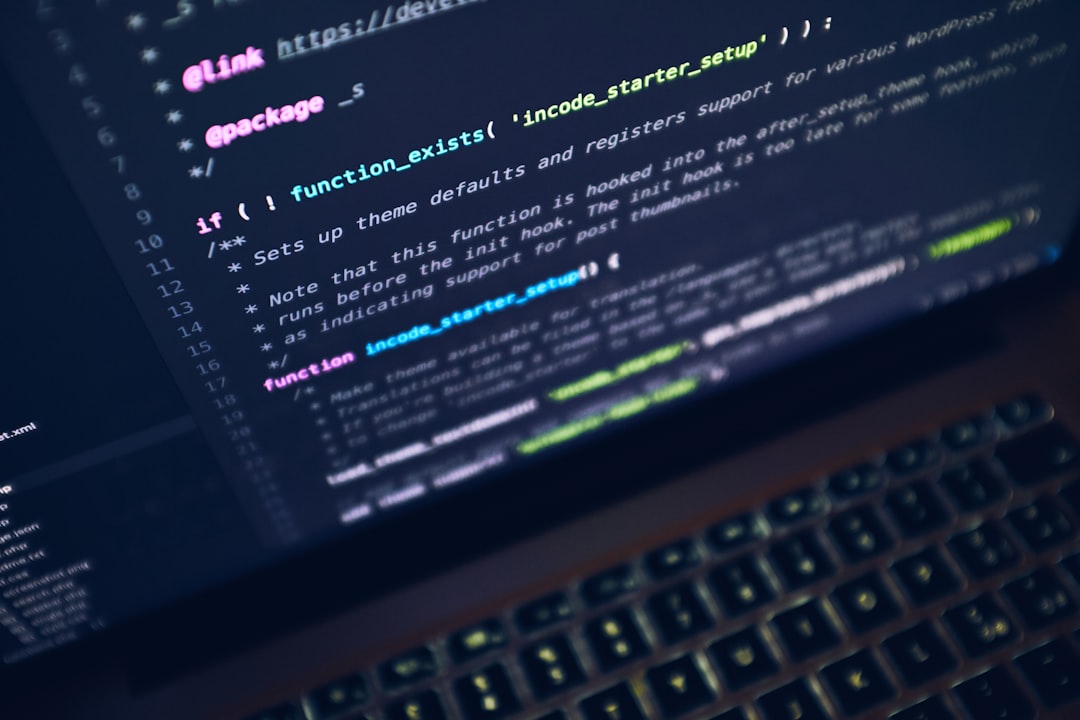
Within the field of artificial intelligence, the safeguarding of machine learning algorithms is paramount, especially in a competitive landscape. Confidentiality terms specifically crafted within non-disclosure agreements (NDAs) are crucial for preventing the unauthorized dissemination of these valuable intellectual property assets. Since algorithms are often classified as trade secrets, it's vital to precisely define what information warrants protection and to establish and uphold measures to maintain that confidentiality. This is because the proprietary nature of these algorithms and the unique approaches they represent can provide a substantial competitive edge.
It's not just the core code of the algorithms that needs protection; the output they generate also can hold substantial value. Companies must ensure that the results of their algorithms – often containing unique patterns or insights – remain confidential and protected from unwanted access by competitors or third-party service providers. The legal landscape around AI and specifically algorithm protection is constantly evolving, filled with legal ambiguity. It's wise to consult with legal experts to craft specific, tailored NDAs to help manage this legal landscape and to understand the scope of protection available to you. By carefully implementing these measures, businesses can navigate the intricacies of intellectual property in the ever-changing world of AI and proactively protect their competitive position.
1. Protecting the specific details of machine learning algorithms through confidentiality agreements can go beyond just the code itself. It can also cover the underlying processes and the data used to train them—things that often set a company's AI apart. This is a crucial aspect of establishing a competitive edge, especially as AI becomes more mainstream.
2. One big risk with confidentiality clauses is that they might not be comprehensive enough to cover every part of how machine learning works. If they're poorly written, there could be gaps that competitors could exploit, potentially letting them access valuable knowledge without any repercussions. It's essential to get them right.
3. When we're talking about AI, specific confidentiality agreements help clarify what counts as a trade secret violation. But they also provide a more nuanced understanding of what can be shared with partners or other collaborators without giving away proprietary information. This careful line-walking is vital for successful collaboration in the field.
4. The legal environment for AI is changing fast. Companies need to be ready to update their confidentiality agreements regularly to make sure they are compliant with new regulations or data protection standards. This constant adaptation is important for avoiding future legal issues.
5. Crafting specific confidentiality terms helps protect trade secrets, but it also gives companies a strategic advantage when negotiating with others. They can highlight the unique value of their AI approaches in a way that sets them apart from competitors. This strategic advantage is a crucial aspect of business negotiations.
6. Having confidentiality agreements with clear terms can deter employees or contractors from taking proprietary information with them. The agreements can reinforce the importance of keeping things secret and outline the consequences of violating the agreement. This creates a greater awareness and risk-aversion surrounding intellectual property.
7. The responsibilities that come with confidentiality agreements are not set in stone. They can place ongoing obligations on employees and external partners to maintain the integrity of the information. This in turn can affect how companies train their teams to handle sensitive data and manage it throughout the lifecycle of a project.
8. There are differences in trade secret laws across countries. Companies must be aware of these variations, both at home and abroad, when drafting their confidentiality agreements. What's protected in one jurisdiction might not be in another, which can make international collaborations complex and legally risky.
9. When trade secret disputes happen in the machine learning world, the financial repercussions can be serious. Cases we've seen demonstrate that stolen proprietary information can lead to millions of dollars in lost revenue. This underscores the importance of safeguarding trade secrets and having a robust legal strategy.
10. It's not enough to just have strict confidentiality agreements in place. Companies also need to invest in training programs to ensure that everyone understands the nature and importance of the trade secrets they are working with. This creates a company culture of information security around sensitive data.
7 Legal Safeguards for Protecting Your AI-Related Intellectual Property Through Non-Disclosure Agreements - Contractual Requirements for Third Party Access to AI Development Tools
When allowing outside entities to utilize your AI development tools, carefully crafted contracts are crucial for protecting your intellectual property. These agreements should clearly outline who owns what – the AI models, the development tools themselves, and any results produced by them. Defining how outside parties can use your data and including provisions for indemnification, which protect you from potential intellectual property infringement claims, are vital aspects. Navigating the complex legal terrain of AI, especially with constantly evolving legal definitions and interpretations, necessitates carefully negotiating these contract terms. Vetting any third-party vendor thoroughly helps ensure they pose minimal legal risk and adhere to applicable regulations. Doing so can help minimize the possibility of future liabilities and strengthen your compliance posture.
When letting others use your AI development tools, it's really important to be aware of the different intellectual property laws around the world. These laws can be very different, so it's tricky to make sure you're not accidentally breaking any rules.
Non-disclosure agreements (NDAs) aren't always perfect. If they're not carefully worded, they can be open to interpretation, and that can lead to problems with information getting out. It's like trying to build a wall with loose bricks—it might look good on the outside, but there are gaps where problems can slip through.
Letting someone else access your tools is not a one-time thing. It can involve ongoing cooperation with certain security measures. If those aren't followed, it can put your confidential info at risk. It's like letting someone borrow your car—you expect them to follow the rules, and if they don't, it could cause harm.
Contracts can have parts that check how third parties use your tools, making sure they stick to the NDA. Without these rules, you could be giving access to competitors or even people who might want to cause trouble. It's like having a security camera in your house—you're able to watch what happens and make sure things are going according to plan.
If someone gets unauthorized access to your tools, it's not just about losing money. Your company's reputation can be harmed and people might not trust you anymore. This makes strong contracts and keeping an eye on how others use them really important. It's like a business's social credit—you don't want your credit rating to go down.
Companies sometimes don't really think about what's considered "okay" to do when giving access to third parties. Broad descriptions can lead to misuse of your tech and secrets. It's like giving someone a blank check—you have no idea how much they'll spend and it could be detrimental.
Having clear rules for data handling and processing in contracts is crucial for both following the law and reducing the chance of data leaks. This includes specific guidelines for storing, sending, and getting rid of data. It's like having a cleanroom—you have to be super careful and follow specific protocols to avoid contaminating your work.
Depending on the field you're in, government bodies might have their own regulations for third-party access. You have to understand these rules if you want to follow the law and run your operations smoothly. It's like navigating different airports with different rules for security and luggage—it's a necessity to understand the protocols of each place you are working.
Using AI tools in the cloud is a growing trend. This means that agreements need to be carefully worded about who owns the data, especially when the collaboration ends. Having a plan for what happens when the relationship is over is very important to avoid arguments. It's like a prenuptial agreement—it outlines who gets what in case things don't work out.
AI tech and the best ways to use it are always changing. This means that contracts for third-party access should be updated over time. Regularly reviewing and revising contracts to stay up-to-date with the latest advances in technology and legal changes is a must. Otherwise, contracts could become outdated and no longer be relevant. It's like a living document—constantly evolving to meet the changing needs of the moment.
7 Legal Safeguards for Protecting Your AI-Related Intellectual Property Through Non-Disclosure Agreements - Breach Detection and Response Mechanisms for AI Asset Protection
Protecting AI assets from breaches is becoming more vital as the field grows more complex and adversarial. Integrating AI itself within security systems can help detect unusual network behavior, aiding in the early identification of breaches. This proactive approach enables swift responses and minimizes damage compared to reactive approaches. Further, the ability to automate incident response procedures allows organizations to respond more efficiently, saving both time and resources during a security crisis.
However, the reliability of AI-driven security measures is still being tested. Simply relying on AI-powered tools isn't sufficient. A well-rounded approach must be taken, incorporating robust policies, employee training, and the creation of detailed security protocols. Human oversight remains critical to successfully interpreting complex AI-generated alerts and ensuring appropriate action is taken.
A holistic approach that blends sophisticated technical tools with comprehensive governance practices is essential. Failing to address both aspects can leave organizations vulnerable to increasingly advanced cyber threats. By properly balancing AI-driven detection capabilities with comprehensive security practices, organizations can enhance their capacity to effectively safeguard their valuable AI-related intellectual property.
Within the realm of AI, safeguarding its intellectual property is becoming more vital as the field becomes more complex and competitive. While contracts like NDAs help establish boundaries, proactive measures for detecting and responding to breaches are crucial. We're seeing a shift toward using behavioral analytics to spot unusual activity, which can help catch breaches early on before they cause serious damage. This is particularly important given that a large portion of breaches are often caused by human error. This emphasizes the need for constant reminders and training, ensuring employees understand security protocols and how to recognize potential phishing or other attack vectors.
The rapid evolution of AI's capabilities, including the application of machine learning, has enabled more sophisticated real-time anomaly detection. By training algorithms to recognize typical behavior and flag deviations, we can potentially greatly reduce the time needed to address threats. This is especially important in the face of a rising trend like ransomware attacks. These attacks require companies to have robust response plans that are well-rehearsed and include things like regular backups and alternative operational strategies. It's interesting that security strategies using multiple layers of defense have shown to be more effective in reducing the likelihood of data breaches than simpler strategies, underscoring the importance of redundancy.
Exploring new technologies like blockchain to improve data integrity in collaborative AI projects is an exciting possibility. Decentralized structures may offer a way to audit data access and usage across partners in a way that's tamper-proof, which is an important advancement. However, the legal ramifications of security breaches can be very serious, with organizations facing potentially immense financial costs per incident. This makes it even more crucial to invest in detection and response systems to mitigate future damage.
Despite the promise of AI in enhancing breach detection, it's not without its limitations. AI-powered detection systems can sometimes generate false alarms, leading to wasted time and effort by IT teams trying to sort through what's real and what's not. Additionally, current regulations like the GDPR and others have strict requirements for notifying individuals when a breach occurs. This necessitates creating fast response protocols that can efficiently communicate with those affected within required timeframes.
It's surprising to find that many companies don't have established, documented procedures for dealing with breaches. The absence of comprehensive incident response plans creates a vulnerability. All companies, regardless of size or field, should have such plans in place and should regularly test them to make sure they're effective. Developing and routinely rehearsing those plans can lessen the damage caused by a security breach and can help the organization adapt better to future incidents.
7 Legal Safeguards for Protecting Your AI-Related Intellectual Property Through Non-Disclosure Agreements - Time Limitations and Geographic Restrictions on AI Knowledge Sharing
When it comes to protecting the intellectual property tied to AI, the ability to control how and when knowledge is shared is crucial. This is further complicated by varying legal frameworks around the world. Some places have specific rules about how long companies can keep certain AI-related information private before it needs to be made public. Other areas may restrict the flow of this information across international borders, impacting cross-border partnerships and the potential for global innovation.
These limitations aren't just bureaucratic hurdles; they can also hinder a company's ability to maintain a competitive edge, especially if valuable insights are not shared with the right people or within the correct timeframe. The challenge for organizations is to develop comprehensive non-disclosure agreements (NDAs) that consider these geographic and temporal limitations. This delicate balance – between protecting valuable AI assets and fostering ethical knowledge sharing – requires careful planning and adaptation. Organizations need to be proactive in understanding and adjusting to the changing landscape of international and local laws concerning AI knowledge sharing to ensure their intellectual property is shielded in a responsible and effective manner.
The pace of AI development introduces a unique challenge when it comes to sharing knowledge, particularly with the rapid obsolescence of certain algorithms and insights. For example, a breakthrough in machine learning today might be outdated in a few months, making the urgency of maintaining confidentiality through agreements all the more crucial.
When collaborating across international boundaries, ensuring compliance with data protection laws becomes much more complex. Regions like the European Union have strict regulations, like the GDPR, regarding the transfer of data, potentially making it challenging to freely share AI tools and data across borders. This creates a hurdle for international collaborations.
Interestingly, the legal status of AI-generated data isn't universally defined. Depending on the location, the outputs of an AI model might not be classified as traditional intellectual property, creating confusion about what exactly can be shared and how it should be protected. This jurisdictional ambiguity adds another layer of uncertainty for researchers and companies operating globally.
Most non-disclosure agreements are designed for a limited time frame, often a few years, which might leave previously classified information exposed when that term expires. This can be a problem as AI development rapidly accelerates, leading to potentially unintentional knowledge leaks.
It's startling to discover that a large portion of businesses don't consistently update their contracts to reflect changes in laws or new regulations related to geographic restrictions. This means they might inadvertently break the law when regulations evolve. This highlights a need for consistent vigilance in legal matters regarding AI.
The inconsistent ways in which different countries regulate the sharing of AI-related data creates a lot of uncertainty. Companies are often working in a grey area, which can lead to penalties or difficulties if they're found in violation of obscure regulations. It seems to be a situation where companies face unnecessary risks without a more uniform global approach.
Companies often don't fully anticipate the amount of time and resources it takes to stay in compliance with changing geographic restrictions related to AI. This can lead to disruptions in their projects and delays in development timelines, particularly if the research or development process is time-sensitive. It seems to be an area where planning and foresight are critically needed.
If partners involved in AI development don't have a solid understanding of the legal implications of time constraints and geographic boundaries, it can hinder the flow of information. This might lead to the creation of information silos and could stifle innovation that might benefit from a broader knowledge base. It is important to understand these parameters from the outset of a collaboration.
It's not uncommon for companies to find themselves in legal disagreements regarding ownership and usage rights when they haven't clearly established protocols for knowledge sharing. This problem can become amplified as regulations and the legal framework evolve in response to the rapid advances of AI. There's a clear need to solidify such protocols.
Many companies haven't fully embraced blockchain technology to support data transactions and track compliance across different jurisdictions. Using blockchain to record data sharing and transactions could create a secure, transparent, and auditable pathway for knowledge sharing that adheres to geographic restrictions. It presents an opportunity for innovation that addresses some of the inherent complexities of AI knowledge sharing.
7 Legal Safeguards for Protecting Your AI-Related Intellectual Property Through Non-Disclosure Agreements - Ownership Rights and Attribution Clauses for AI Generated Content
The question of who owns and how to attribute AI-generated content is a complex and shifting legal matter. It's not clear-cut whether the person using the AI, the AI software maker, or the person who initially prompted the AI has the primary ownership rights over the final product. Courts are starting to address these issues, and some governments are proposing laws to try and bring clarity to the situation. This uncertainty surrounding intellectual property rights is a key concern for companies and individuals who are making use of AI to create new things. As the use of AI for creative purposes expands, it becomes ever more important to have transparent rules about who gets credit and who owns the work produced. This evolving legal landscape calls for a close watch and a readiness to adjust legal frameworks to keep pace with AI innovation and its far-reaching effects. It's still early days in terms of resolving these matters, and there's a risk of misinterpretations or unexpected consequences.
The legal landscape surrounding who owns AI-generated content remains blurry, especially when considering the source materials used to train the AI. If an AI model learns from copyrighted works, even if the final output appears new and unique, questions about ownership can arise. This can impact who gets credit for the content.
Many legal systems still lack clear rules on who's considered the creator of AI-generated work. This lack of clarity makes attribution a tricky business, especially when multiple individuals or groups contribute training data to a project. It can quickly become a dispute about ownership.
Adding clauses in agreements that deal with attribution can help establish who deserves recognition for the work, but also protects everyone involved from copyright infringement accusations. They provide a clear roadmap for responsibility in AI-generated outputs.
When AI-generated content is misused, it can lead to hefty legal penalties. We're talking about lawsuits with damages reaching hundreds of thousands, even millions, depending on the harm to the creator of the original content. This potential risk underscores the importance of clear attribution.
Despite the rapid advancement of AI technology, a lot of contracts haven't caught up in adapting to those changes. This can create situations where organizations are bound by older agreements that don't reflect the current understanding of AI ownership rights.
In certain legal systems, the person operating an AI tool might be viewed as the author of what it creates. This raises the question of who truly owns the output – the AI creator or the user? It's a question that highlights the changing nature of creation and authorship.
Attribution of AI-generated content isn't always guaranteed, especially in environments like open-source projects. In these contexts, broader sharing practices can make it difficult to identify individual contributions, leading to uncertainty about who holds the rights.
Generative AI tools are presenting a big challenge to established intellectual property systems. Legal scholars are calling for changes in the law that account for how digital content is being created in new ways.
Companies should be extra cautious when drafting NDAs that relate to AI output. Vague or poorly written language can easily undermine the ability to claim ownership or attribute authorship should a dispute arise. It's crucial to be precise in the wording of such agreements.
Solutions like blockchain are being explored to improve how we track the origin and ownership of AI-generated content. By creating permanent records of data usage and sources, blockchain could offer a more transparent and immutable approach to establishing attribution. This potential for improved transparency is intriguing and could address some of the existing issues.
AI-Powered Patent Review and Analysis - Streamline Your Patent Process with patentreviewpro.com (Get started for free)
More Posts from patentreviewpro.com: