AI-Powered Patent Review and Analysis - Streamline Your Patent Process with patentreviewpro.com (Get started for free)
Patent Eligibility Criteria for Artificial Intelligence Innovations A 2024 Analysis of USPTO Standards
Patent Eligibility Criteria for Artificial Intelligence Innovations A 2024 Analysis of USPTO Standards - USPTO July 2024 Framework Updates Integrate AI Patent Analysis Methods
The USPTO's July 2024 framework update focuses on refining the process of evaluating patent eligibility for artificial intelligence inventions. While reiterating the continued use of the existing PEG framework, the update underscores the crucial role of discerning whether AI-related claims are tied to abstract ideas. It then emphasizes the need to analyze how these claims integrate the abstract idea into a concrete and practical application. This adaptation by the USPTO can be interpreted as a direct response to the explosive growth of AI technologies. The revised guidelines provide a structured approach to analyze AI-related patent claims, offering a path forward for understanding how these innovations can be evaluated within the framework of current patent law. Notably, the extension of the comment period allows for further input from the community, highlighting a commitment to create an environment that encourages innovation while prioritizing safety and trust in AI development. The updated guidance, therefore, demonstrates a conscious effort to keep patent law current with the pace of technological progress, navigating the challenges presented by this evolving landscape.
In July 2024, the USPTO rolled out revisions to their framework for evaluating AI patent applications. These changes, aimed at enhancing the speed and accuracy of patent examination, leverage AI algorithms to sift through prior art more quickly than traditional methods. This includes a refined classification system built to better categorize AI inventions, potentially simplifying the examination procedure and mitigating ambiguity in patent claims.
However, some legal scholars express worry about potential biases within these AI-driven systems, wondering if they could inadvertently overlook important aspects of AI innovations. As part of these updated practices, the USPTO has established a mechanism where applicants can submit the AI-generated content of their inventions. This allows examiners to more readily grasp the technology underlying the patent application.
The USPTO's revised guidelines emphasize clear and complete disclosure about the training process and datasets used in AI inventions. This is crucial because such details heavily influence a patent's validity and enforceability. The increased use of AI in patent analysis has also sparked more discussion between engineers and patent attorneys, leading to refined terminology for describing AI inventions within patent applications.
Furthermore, the USPTO is now encouraging applicants to back up their claims of novelty with empirical data. This marks a subtle shift towards a more evidence-based approach for assessing the eligibility of AI patents. To further their understanding of AI, USPTO staff are receiving specialized training on various AI-related technologies. This training aims to equip them to tackle patent applications within this ever-changing field.
The question of who owns the intellectual property when an AI system independently creates an innovation remains a topic of discussion. This question raises broader implications concerning the future of AI in intellectual property law. These USPTO changes could influence other global patent offices as they grapple with integrating AI into their own intellectual property frameworks.
Patent Eligibility Criteria for Artificial Intelligence Innovations A 2024 Analysis of USPTO Standards - Machine Learning Patent Applications Must Now Detail Technical Implementation Steps
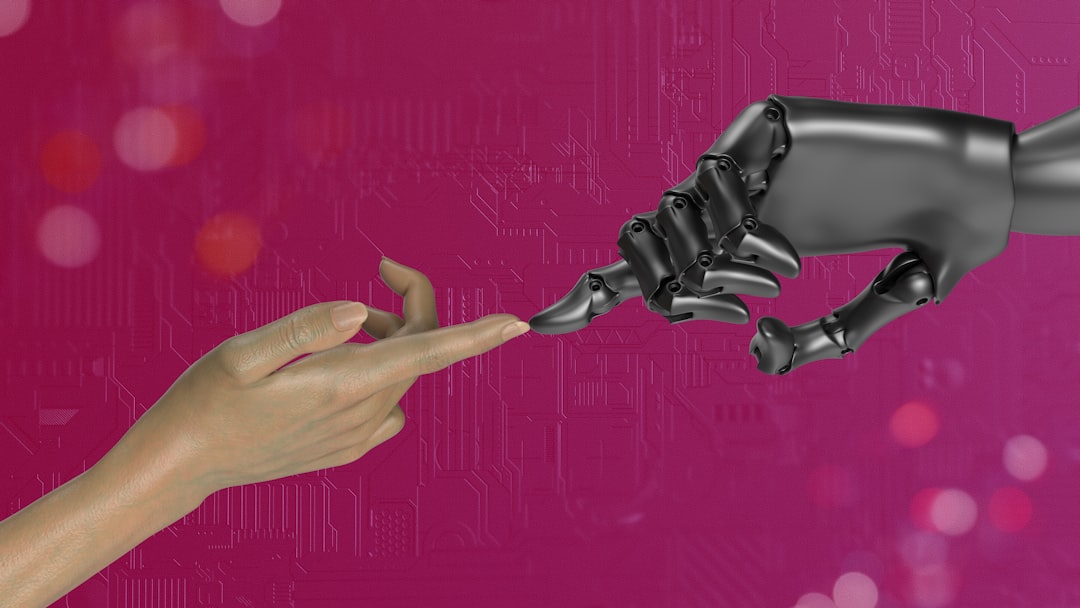
The USPTO has recently mandated that machine learning patent applications must now include detailed explanations of the technical steps involved in their implementation. This new requirement is part of a wider effort to clarify patent eligibility criteria for AI innovations. The goal is to ensure that patent claims are tied to real-world applications rather than just stating desired outcomes. By demanding a comprehensive description of how AI inventions work, the USPTO hopes to provide a clearer path towards obtaining a patent. This shift could influence the way innovation happens in the AI field. It may also make it harder to get a patent, as applicants might need to present stronger proof that their inventions actually work and are useful. These changes ultimately show how patent law is evolving to keep up with the rapid pace of technological progress.
The USPTO's recent adjustments to patent eligibility criteria for AI inventions, specifically requiring detailed technical implementation steps, signify a push for more clarity and precision in patent applications. It's a move towards ensuring AI innovations are not shrouded in ambiguity but rather are described with a degree of specificity that could result in more robust and defensible patent claims. However, it seems that the increasing complexity of AI and machine learning methods might create challenges for patent examiners, particularly those without a strong technical background. There's a need for continuous training and education for patent professionals to keep pace with this quickly developing field.
Another significant change is the emphasis on using empirical data to support claims of novelty. This new approach encourages patent applicants to move beyond mere theoretical assertions and present actual evidence for their claims, leading to a potential improvement in the quality of patent applications. Though, it's worth noting that the new guidelines necessitate the disclosure of training data, a practice that hasn't always been strictly enforced in the past. This requirement raises concerns about trade secrets, potentially limiting a company's ability to protect proprietary datasets.
Furthermore, the use of AI in patent examinations has sparked conversations around the possibility of bias within these systems, leading to a heightened focus on fairness and equitable evaluation practices. This heightened scrutiny is crucial for maintaining the integrity of the patent system. Yet, the updated framework may inadvertently favor larger companies, who possess the resources to undertake comprehensive documentation and empirical research. This situation could potentially create a disadvantage for smaller entities and independent inventors who may not have the same capabilities, potentially tilting the playing field in favor of those with deeper pockets.
The USPTO is also promoting collaboration between engineers and patent attorneys, suggesting a shift towards an interdisciplinary approach to patent applications. This can lead to innovations in the legal and technical language used to describe AI-related innovations. This shift is also intertwined with the increasing use of AI-generated content in applications, which, although intending to streamline the examination process, raises important questions about ownership and authorship of inventions created by autonomous systems.
Given the rapidly evolving role of AI in generating patentable ideas, it's likely that the new requirements will challenge long-held notions of invention and creativity. This situation might call for a reevaluation of intellectual property laws. The influence of the USPTO's approach could extend beyond its borders as other patent offices contemplate similar frameworks for AI inventions. This development may lead to inconsistent practices across nations, making navigating global innovation and intellectual property protection more complex for companies working with AI. It seems that the path ahead for AI patents and related intellectual property is rife with interesting challenges and evolving landscapes.
Patent Eligibility Criteria for Artificial Intelligence Innovations A 2024 Analysis of USPTO Standards - Natural Language Processing Patents Face New Subject Matter Requirements Under 35 USC 101
The USPTO's updated guidelines under 35 USC 101 have introduced stricter requirements for natural language processing (NLP) patent applications, reflecting a broader effort to refine patent eligibility standards for AI technologies. This revised approach involves a two-part assessment: first, determining if a patent claim aligns with the four established categories of patentable subject matter, and second, examining whether it falls within any judicial exceptions, such as abstract ideas. This heightened scrutiny necessitates a detailed description of how NLP inventions are technically implemented, a shift towards a more evidence-based approach for determining novelty and utility.
While the changes aim to clarify patent eligibility and encourage innovation, there are potential concerns. These new requirements could introduce biases within the patent review process, especially if they favor larger companies with ample resources to fulfill the detailed documentation and research needs. There is a risk of unintentionally hindering smaller entities or individual inventors who may struggle to meet the stricter standards. Ultimately, these developments underscore the evolving landscape of AI patent law, where the push for innovation must be balanced with maintaining a fair and rigorous standard for patent eligibility.
The USPTO's updated guidance on patent eligibility, particularly for natural language processing (NLP), marks a significant shift in how AI-related inventions are evaluated under 35 USC 101. It's attempting to delineate more clearly between abstract ideas and tangible applications in AI-driven innovations, which could shape how NLP patents are pursued and granted in the future.
The current requirement to include detailed technical implementation steps for AI applications, especially within NLP, might fundamentally alter the patent landscape. It could deter applications that lack a clear practical implementation, potentially leading to patents being more closely tied to real-world outcomes. It's a change that might reshape the way innovations are conceived and protected.
Furthermore, the USPTO's increasing emphasis on empirical data to prove novelty presents both opportunities and challenges. While this could lead to more rigorous and potentially higher-quality patent evaluations, it could disadvantage smaller organizations or startups that may not possess the resources to collect and analyze such data.
The growing inclusion of AI-generated content in patent applications has sparked debate about ownership and authorship. This raises important questions about the fundamental nature of intellectual property and how invention and creativity are viewed within the legal framework. It's a topic that might necessitate a rethinking of established intellectual property norms.
In addition, the new emphasis on revealing training datasets in applications poses a dilemma for businesses. It creates tension between the requirement for transparency and the need to safeguard sensitive, proprietary data. Companies must carefully navigate these new constraints to protect trade secrets while complying with the updated guidelines.
It's not without its concerns. The inherent complexity of many NLP and AI models may outstrip the technical capabilities of some patent examiners. This could lead to misunderstandings or oversights within the evaluation process, potentially impacting the quality of patent decisions.
These changes underscore the necessity for greater collaboration between engineers and patent attorneys. The future of AI-related patent applications likely hinges on a more thorough understanding of both the technical nuances and legal requirements. This will undoubtedly influence the composition of patent teams and the skillsets needed to navigate this complex field.
There's a growing concern that the new standards, while promoting stronger patent applications, might inadvertently favor larger corporations that have the resources for comprehensive documentation and extensive empirical testing. This could potentially create a more uneven playing field for smaller startups and independent inventors, making it harder for them to compete for intellectual property protection.
Moreover, the approach taken by the USPTO for NLP patent eligibility could influence how other patent offices across the globe handle AI-related innovations. This might lead to a heterogeneous set of standards globally, which could complicate matters for organizations aiming for international intellectual property protection.
These tighter patent requirements could fundamentally shift how innovations are approached within the AI field. Companies may need to adopt different practices to document and communicate their inventions, impacting the pace of development and overall innovation within the NLP and AI sectors. The landscape of AI patent law continues to evolve, posing exciting, yet challenging, questions for both researchers and policymakers.
Patent Eligibility Criteria for Artificial Intelligence Innovations A 2024 Analysis of USPTO Standards - Computer Vision Patent Claims Need Hardware Integration Documentation After 2024 Update
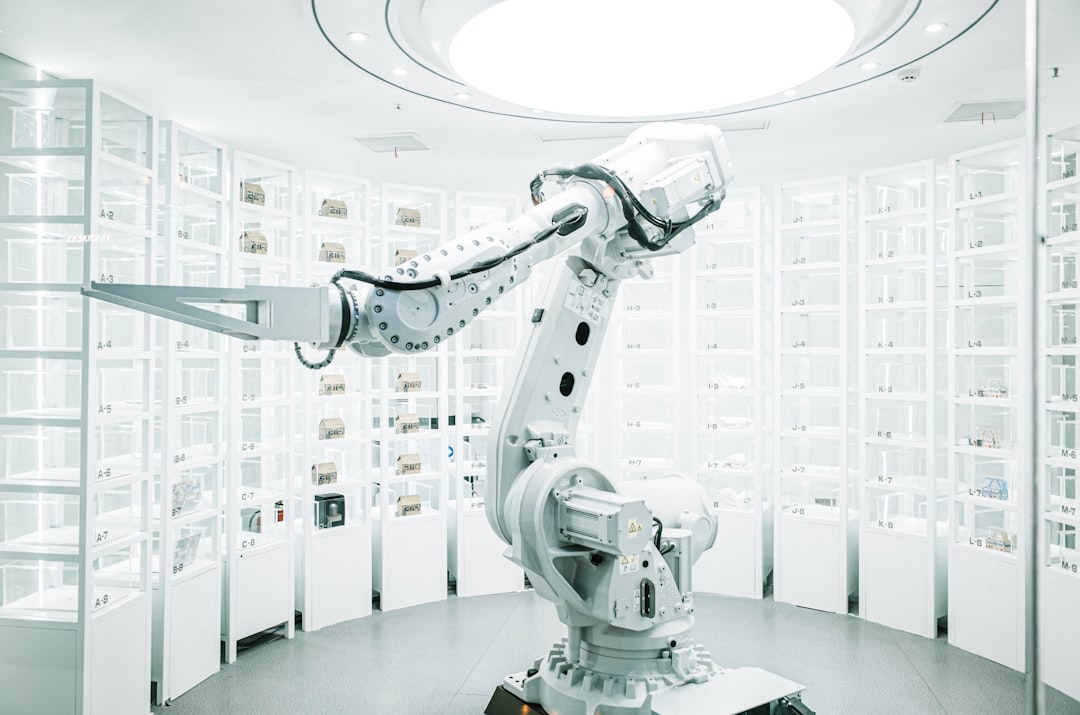
The USPTO's July 2024 update to patent eligibility standards has introduced a notable change for computer vision patent applications: they must now include detailed documentation of how the invention interacts with physical hardware. This requirement, effective July 17th, 2024, signifies a move towards a more concrete assessment of AI inventions. Instead of focusing solely on the abstract concepts of computer vision algorithms, the USPTO now necessitates a demonstration of how these algorithms are integrated into working systems. This new emphasis reflects a broader effort to ensure that AI-related patents are grounded in practical applications and not merely theoretical constructs.
While the goal is to promote clarity and encourage responsible innovation, some might view this shift as potentially more stringent. The need to demonstrate tangible hardware implementation may pose a challenge for some applicants, especially smaller inventors or those whose focus is on the development of the core AI algorithms themselves. The USPTO aims to balance the protection of innovative ideas with the assurance that patented inventions have genuine utility and are more than just abstract concepts. This update is yet another adjustment within patent law as it seeks to accommodate the unique and rapid changes occurring within the AI field.
The USPTO's July 2024 update to patent eligibility criteria, particularly impacting artificial intelligence (AI) inventions, now emphasizes a stronger connection between software and hardware for computer vision patent applications. Specifically, they've made it clear that, moving forward, patent claims need to demonstrate how computer vision algorithms are integrated with tangible hardware components. This shift from theoretical concepts towards practical application is part of a broader effort to ensure that patents aren't granted for abstract ideas alone.
This new requirement adds a layer of complexity to the patent application process. It isn't enough to just describe an algorithm; engineers and inventors must now meticulously outline how it interacts with various hardware configurations. This need for detailed documentation can present a significant hurdle, particularly for smaller startups or independent researchers who may not have the resources to fully detail hardware integration across different scenarios.
The increased focus on hardware integration is likely to change how companies approach patenting their AI innovations. We'll likely see more collaboration between software and hardware engineers during the patent application process to ensure the necessary information is captured. However, it's concerning that this added complexity might inadvertently create a bias towards larger companies with extensive resources for detailed documentation. There's a legitimate worry about smaller entities being unfairly disadvantaged in the patent landscape.
Furthermore, as the USPTO strengthens its focus on hardware integration, we can expect a potential rise in patent rejections due to insufficient documentation. While this approach will hopefully lead to a higher quality of granted patents, it may also slow the pace of innovation if some innovators are unprepared for the added scrutiny. Existing patents may also be subject to closer review to determine if they meet the new criteria, potentially leading to challenges for companies seeking to protect their older innovations in this evolving environment.
The new rules also contribute to the growing trend of demanding empirical evidence to back up patent claims. This approach, while aiming to improve patent quality, could potentially discourage more speculative or foundational AI research, preferring to see immediate practical application. It's a fascinating dynamic—a push for validation, but perhaps at the expense of exploring the potential of innovative AI concepts.
The changes will require the USPTO to revise their examination practices, necessitating more specialized training for patent examiners to assess the relationship between AI models and hardware. This is a critical step to ensure the quality of patent evaluations in a complex and rapidly evolving field. However, this updated approach may lead to inconsistencies internationally, as different patent offices navigate these new standards. It's entirely possible that global harmonization will be a challenge, potentially creating difficulties for companies seeking international patent protection for AI innovations.
In essence, the 2024 update represents a subtle shift in the way computer vision innovations are evaluated, emphasizing a more practical and evidence-based approach to patent eligibility. While this could lead to more robust patents, it will also introduce new complexities for innovators, particularly those without extensive resources. It will be fascinating to see how these changes reshape the computer vision patent landscape in the coming years.
Patent Eligibility Criteria for Artificial Intelligence Innovations A 2024 Analysis of USPTO Standards - Automated Decision Systems Patent Applications Require Clear Human Oversight Protocols
The USPTO's updated guidance for AI patent applications places significant emphasis on the inclusion of well-defined human oversight protocols, particularly within automated decision systems. This focus highlights the ethical dimension of AI development, acknowledging the importance of human intervention in ensuring the reliability and trustworthiness of these systems. The idea of a "human-in-the-loop" approach to patent examinations is promoted, suggesting that integrating human judgment into the automated patent review process is crucial for responsible innovation. The ongoing conversation surrounding AI necessitates the creation of strong governance structures, aiming to strike a balance between promoting innovation and upholding ethical standards in this quickly evolving field. While the desire to streamline patent evaluation through AI is understandable, this emphasis on human oversight suggests a recognition of the potential pitfalls of relying solely on automated systems in such a sensitive area.
The USPTO's updated guidance emphasizes the need for clearly defined human oversight protocols within any automated decision system used in patent applications, particularly those involving AI. This is a crucial step in ensuring that AI-driven processes don't operate autonomously, which, in turn, contributes to the overall reliability and accountability of AI inventions, especially when it comes to patentability.
This increased focus on human oversight is partially due to the valid concerns about potential biases embedded within AI algorithms. These biases can significantly impact how decisions are made within the patent process, potentially leading to inconsistencies in treatment of different applicants.
Furthermore, the updated guidelines promote more comprehensive disclosure in AI patent applications, not just to explain the technical workings of the invention, but also to shed light on the decision-making logic behind automated systems. This level of transparency is essential for validating patent claims and guaranteeing that the review process is fair and impartial.
The introduction of AI-driven systems for generating patent content raises complex questions regarding ownership and intellectual property rights. With the addition of mandated human oversight protocols, determining who rightfully owns AI-generated inventions could become quite convoluted, forcing a reevaluation of established concepts of inventorship.
These new standards suggest that without clearly articulated human oversight, AI-generated patent applications might be met with greater scrutiny or even rejection. This dynamic reveals a crucial tension between encouraging innovation and demanding ethical accountability in automated systems, particularly those impacting significant decisions like patent approval.
Incorporating detailed human oversight protocols into the application process can potentially improve the overall quality of patent submissions, according to the USPTO. By prioritizing human involvement in critical decisions, it lessens the reliance on exclusively automated assessments. This is believed to enhance the decision-making process by ensuring human intellect is integrated with the capabilities of AI.
The call for clearer human oversight is especially important for evaluating newer, rapidly evolving technologies where established frameworks might be insufficient. The dynamic nature of innovation, particularly in the AI space, underscores the need for adaptable and rigorous assessment standards.
This push for transparency within AI systems also serves as a countermeasure to the potential risks associated with "black box" AI, where the internal decision-making processes remain obscured. Having a clearer picture of how AI systems reach specific conclusions is crucial for understanding their impact and ensuring fairness.
One potential side effect of these new guidelines could be an increase in the costs and complexities of patent applications, especially for smaller inventors or companies with limited resources. These stakeholders now have to demonstrate not only the technical merit of their AI invention but also a comprehensive human oversight structure.
Ultimately, the USPTO's emphasis on human oversight is likely to lead to more collaboration between engineers and patent attorneys. Both parties need to ensure that the implications of AI-driven decision-making are clearly understood and adequately expressed within the patent framework. This interdisciplinary approach is essential to navigate the unique challenges posed by the integration of AI in the patent application process.
Patent Eligibility Criteria for Artificial Intelligence Innovations A 2024 Analysis of USPTO Standards - Training Data Requirements Added to Neural Network Patent Applications in Mid 2024
Starting in the middle of 2024, the USPTO made significant changes to the way they assess patent applications involving artificial intelligence. A particularly notable alteration was the implementation of new standards specifically for neural networks, demanding that applicants provide details about the training data used to develop these AI systems. This requirement, intended to improve transparency and ensure rigorous validation of AI inventions, necessitates a thorough description of the datasets used to train the neural network.
The USPTO likely hopes this will encourage the use of empirically validated results and create more accountability. While this emphasis on concrete evidence is understandable, there are concerns that this increased demand for specificity and detail might present obstacles for smaller inventors and companies. The resources and expertise required to meet these new standards might be beyond the reach of many, potentially creating a less level playing field within the patent process. This new push for transparency in AI patent applications is part of a broader evolution in patent law as it attempts to grapple with the complex and rapidly changing world of artificial intelligence.
Following the USPTO's July 2024 updates, patent applications for neural networks and AI-related inventions now necessitate the disclosure of the training data used in the system. This means applicants must detail the data's nature, scope, and any inherent limitations, adding a layer of complexity to the patent process. While promoting a degree of transparency, it simultaneously creates tension, especially for businesses seeking to safeguard valuable, proprietary datasets – potentially creating a conflict between patent eligibility and trade secret protection. This could inadvertently stifle innovation for those companies that rely heavily on their unique datasets for a competitive edge.
Interestingly, this standardization of training data requirements could unwittingly introduce or reinforce biases present in those datasets directly into the patent examination process itself. The question of whether a patent evaluation process informed by biased datasets is fair and equitable is a critical concern that raises doubts about the integrity of the patent evaluation process moving forward.
Furthermore, the USPTO is now emphasizing the importance of providing empirical evidence to support claims of novelty. While this encourages higher-quality patents overall, it could potentially disadvantage smaller startup companies who might lack the resources necessary for extensive data collection and analysis. This new reliance on empirical evidence for novelty appears to favor larger, established companies, possibly creating an unbalanced landscape where smaller innovators struggle to compete.
Naturally, these shifts necessitate closer collaboration between engineers, who understand the technical aspects of the invention, and patent attorneys, who are experts in the legal framework surrounding patents. This collaboration will be crucial to effectively navigate the complex landscape of documenting and describing these increasingly sophisticated inventions. It’s likely to result in a shift in the type of skills needed within patent teams, and likely influence patent application strategies themselves.
The push for stricter oversight of AI systems within patent applications, especially for automated decision-making, reflects a growing understanding of the ethical issues associated with AI applications. This recognition of the need for human oversight is primarily driven by a desire to prevent negative consequences from inadvertently embedded biases within algorithms.
These stricter requirements are anticipated to lead to an increase in patent rejections due to insufficient documentation, potentially creating more hurdles for smaller inventors and companies. These actors may not possess the resources to comply with the increased demands for comprehensive technical and empirical details required by the updated standards.
Given that the USPTO is establishing more stringent standards, there's a risk of increased global variation in patent standards across different jurisdictions. This creates a possibility of inconsistencies in patent protection for AI innovations across national borders. Navigating the international landscape of intellectual property rights for AI inventions is likely to become even more complex.
The USPTO's efforts to promote greater transparency in AI patent processes also address the concerns around 'black box' AI systems. A focus on transparency aims to clarify the rationale behind automated decisions, improving the ability to evaluate patents and ensuring the overall fairness of the process. However, this transparency might be challenging to achieve in practice, particularly for more complex AI models that operate in opaque ways.
The changes introduced in 2024 are likely to profoundly impact the way companies approach and document patent applications for AI. Companies might find themselves shifting their focus towards innovations with immediate and practical applications. This could result in a slower pursuit of more speculative or abstract AI-based innovations that are harder to document with empirical evidence. The evolving landscape of AI patent law is creating new challenges and potential constraints for researchers and innovators.
Ultimately, the increased scrutiny surrounding AI patents raises important questions about how we balance the need for innovation with the need for ethical consideration and transparency in the development and deployment of AI technologies. This dynamic is likely to remain a focal point for discussion among policymakers, legal professionals, and innovators within the AI field in the years to come.
AI-Powered Patent Review and Analysis - Streamline Your Patent Process with patentreviewpro.com (Get started for free)
More Posts from patentreviewpro.com: