John McCarthy's 1956 Dartmouth Proposal The Birth Certificate of Modern AI Patents
John McCarthy's 1956 Dartmouth Proposal The Birth Certificate of Modern AI Patents - The McCarthy McCarthy Proposal Original Typescript and Its Journey to Stanford Archives
John McCarthy's original 1955 proposal, a 17-page document, lays bare the initial vision for a groundbreaking summer research project focused on artificial intelligence. It's a remarkable artifact because it is, essentially, AI's "birth certificate." Not only does it detail the goals of a ten-person study slated for the summer of 1956, but also showcases the impressive minds behind the initiative—including McCarthy, Minsky, Rochester, and Shannon, each a major figure in their respective fields of computer science and information theory.
This proposal, housed in both the Dartmouth College and Stanford University archives, stands as a cornerstone for the field's history. It was in this document that the term "Artificial Intelligence" made its first official appearance, marking a decisive moment in the development of the field. It signifies that the fledgling AI community was not just a loose collection of thinkers, but a group with the ambition and the clear goals to build a recognized field of study. Its journey into the permanent collection of these institutions underscores the recognition of this document’s pivotal role in sparking a future of technological advancement. The careful preservation of this artifact shows the importance of recognizing seminal works in a growing and often fast-changing field.
The original McCarthy Proposal, penned in 1955, was crafted in a handwritten format, a stark contrast to the digital tools prevalent in today's engineering and technology development. This document, a 17-page typescript plus a title page, serves as a primary source into the early conception of artificial intelligence. Copies of it reside in both Dartmouth's and Stanford's archives, each representing a stage of its journey through time.
The core of the proposal lays out the ambitious goal of studying artificial intelligence, a term first documented in this very proposal. It proposed a summer research project, a two-month intensive study with a small team of researchers – a bold and unprecedented idea at the time. The individuals who authored the proposal, notably John McCarthy, Marvin Minsky, Nathaniel Rochester, and Claude Shannon, were key figures in their respective fields, making the proposal itself carry a certain weight of intellectual authority.
The initial reception of the proposal wasn't overwhelmingly positive; it took several years for the broader academic community to engage seriously with the concept of AI. However, the Dartmouth conference that this proposal birthed is frequently credited as the catalyst for AI becoming a field of study. Its significance, often referred to as the "birth certificate" of modern AI, underscores its central role in the history of this technology.
The proposal envisioned a path toward developing programs with the capacity for adaptive learning – what we now know as machine learning. Furthermore, it advocated for “a way of describing computed things,” a concept that has found relevance in today's computer programming and software engineering. It even broached the topic of "intelligent machinery," prefiguring the contemporary dialogues surrounding automation and robotics. The authors showed a level of foresight about the potential impacts, including ethical considerations, related to this emerging field of study.
The typescript's path to Stanford involved preservation and transfer efforts, a testament to the significance attributed to this foundational AI document. Interestingly, the proposal included specific insights into machine reasoning and formal logic, suggesting a clear understanding of the relationship between programming languages and machine intelligence. The digitization of the typescript for preservation represents the changing landscape of archival practices in academia, providing greater accessibility for researchers than previously possible with physical copies only.
Even today, almost 75 years later, this proposal acts as a touchstone for modern discussions about the scope and future of AI, attesting to its continuing influence on the field. While AI research has evolved dramatically, the original McCarthy proposal continues to resonate, a potent reminder of the visionary ideas that laid the groundwork for the advancements we see today.
John McCarthy's 1956 Dartmouth Proposal The Birth Certificate of Modern AI Patents - Birth of The Term Artificial Intelligence in Summer 1956 Dartmouth
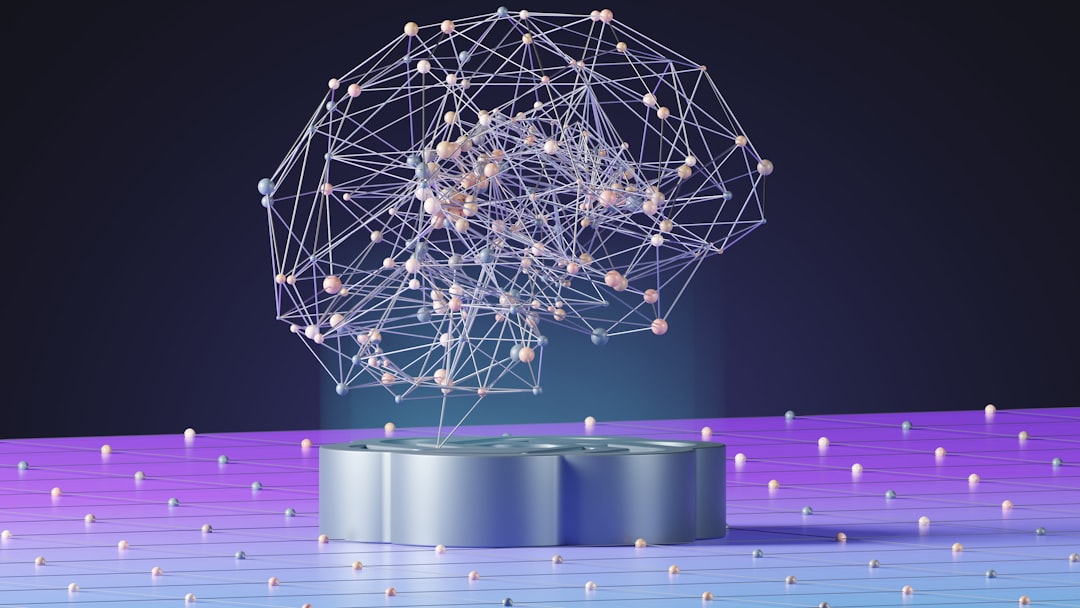
The summer of 1956 witnessed the birth of artificial intelligence as a distinct field of study with the Dartmouth Summer Research Project. Held from June 18th to August 17th, this workshop, organized by John McCarthy, Marvin Minsky, Claude Shannon, and Nathaniel Rochester, is widely considered the genesis of modern AI.
Crucially, it was at this Dartmouth gathering that the term "Artificial Intelligence" first appeared, solidifying the concept and giving it a formal identity within the scientific community. The event drew together top researchers in computing and cognitive science, all aiming to unlock the mysteries of machine intelligence. It sought to explore how computers could be programmed to perform tasks typically associated with human intelligence, such as problem-solving, learning, and language understanding.
This endeavor, often called the "Constitutional Convention of AI," underscored the ambitious goals of these pioneers. They anticipated that focused collaborative research could significantly advance the nascent field of artificial intelligence. Their efforts were ultimately successful in laying the foundation for future AI research, inspiring generations of researchers and shaping the trajectory of the field. The Dartmouth workshop's profound impact continues to be acknowledged and celebrated by the AI community even today, almost 75 years later.
The Dartmouth Summer Research Project on Artificial Intelligence, held from June to August 1956, was a pivotal moment in the history of the field. The term "Artificial Intelligence," first formally used in the Dartmouth proposal, was initially met with a degree of skepticism. Many researchers questioned if machines could truly emulate human thought, considering it almost a playful notion at the time.
Interestingly, the event had a more relaxed, summer-session feel rather than the usual rigid academic conferences. This informal atmosphere likely contributed to the collaborative and innovative spirit that permeated the gathering. Central to the Dartmouth proposal was the need to establish a firm definition of intelligence, something that continues to challenge AI researchers today. Different perspectives on what constitutes "intelligent" machine behavior persist.
McCarthy's choice of Dartmouth was deliberate; he sought to capitalize on the college's intellectual environment and the inspiring setting to fuel the researchers' creativity during the intensive two-month study. It's fascinating that the event drew researchers from a variety of backgrounds, encompassing psychology, neuroscience, and computer science. This early interdisciplinary approach proved crucial in driving the field forward.
The Dartmouth Conference produced a considerable volume of papers and proposals that laid the groundwork for the future trajectory of AI research, demonstrating a remarkable surge in ideas within a relatively short timeframe. While "machine learning" wasn't specifically used in the proposal, the core concepts that define this essential AI subfield were indeed discussed.
It's intriguing to look back and realize how optimistic those initial pioneers were. They predicted that machines would achieve human-level intelligence within a few decades, a timeframe that has significantly extended over the last 60 years. The Dartmouth proposal established a novel precedent for structuring research goals in AI, essentially acting as a blueprint for numerous future projects. This process of defining clear aims within scientific exploration has since become commonplace.
Despite its immense significance, the proposal wasn't universally embraced initially. Some critics found it overly ambitious and lacking in concrete methodologies. This reaction mirrors a larger ongoing conversation in scientific circles: the delicate balance between visionary goals and practical, achievable methods in the nascent stages of a new field.
John McCarthy's 1956 Dartmouth Proposal The Birth Certificate of Modern AI Patents - Mathematics Meets Psychology The Novel Cross Disciplinary Workshop Format
The "Mathematics Meets Psychology" workshop format embodies a shift towards interdisciplinary collaboration, mirroring the pioneering spirit of the 1956 Dartmouth AI conference. It represents a novel way to address complex cognitive issues by combining the structured tools of mathematics with the nuanced observations of psychology. This cross-disciplinary approach goes beyond simply combining fields; it seeks a deeper understanding of cognition by examining the role of cultural context in numeracy and educational practices. Examples like R. Duncan Luce's work on semiorders show how psychological concepts can actually shape mathematical theories. This dynamic interplay enriches both disciplines, fostering a more comprehensive perspective on intelligence. As various fields continue to develop, the importance of such collaborative endeavors is becoming clearer. This ultimately suggests a future where a unified approach is vital for successfully tackling intricate problems in AI and cognitive sciences. It's a powerful illustration of how combining different perspectives can lead to a more robust understanding of the human mind.
The Dartmouth workshop's inclusion of a "Mathematics Meets Psychology" session marked a fascinating intersection within the emerging AI field. It showcased the potential of applying mathematical tools to understand and model human cognitive processes. This cross-disciplinary approach was a novel idea at the time, highlighting the need to draw upon insights from psychology to build more effective AI systems.
One of the intriguing outcomes of this focus was the realization that human thought processes, including problem-solving, could be represented using mathematical algorithms. This connection between seemingly distinct fields was surprising, yet it provided a new way to bridge the gap between human and artificial intelligence. It opened up the possibility of creating AI programs that more closely mirrored human decision-making and learning styles.
By integrating psychological theories into mathematical frameworks, researchers were able to quantify abstract concepts like decision-making and learning. This approach led to the development of predictive models that, in turn, enhanced the ability of AI systems to imitate human behavior in a more sophisticated way. An interesting aspect was the exploration of how cognitive biases, which are common in human decision-making, impacted AI system performance. This realization demonstrated the importance of understanding the nuances of human psychology to create better algorithms.
Furthermore, the workshop questioned the sole reliance on strictly logical AI approaches, advocating for the incorporation of probabilistic models to account for the inherent uncertainties present in human thinking. This focus on probabilistic models is still a vital aspect of modern AI research. It underscored the need for AI to deal with the world's messiness, not just its simplified, abstract representations.
The workshop highlighted the crucial role that emotional and psychological factors could play in optimizing AI decision-making and machine learning, an area gaining increasing prominence in today's AI discussions. The idea that AI systems could be designed to better interact with humans by understanding and perhaps even simulating emotional responses was quite forward-thinking at the time. This understanding proved to be foundational to better human-AI interactions.
This convergence of fields also influenced areas like natural language processing. Researchers found that understanding how humans communicate required insights from both mathematics and psychology to develop more accurate algorithms that could recognize context and subtle variations in language. It was a precursor to the current area of interest in AI concerning explainability and interpretation.
The mathematical representation of cognitive load theory from psychology also emerged from the workshop as a powerful tool. It provided a framework for designing user interfaces that catered to human cognitive limits. Researchers started looking at how to optimize the interactions between humans and AI systems, a very practical application of this interdisciplinary perspective.
Ultimately, this early intersection of mathematics and psychology, exemplified in the "Mathematics Meets Psychology" workshop, contributed to the broader understanding of AI's potential and its limitations. It was a step towards recognizing the crucial role of this interdisciplinary connection not only in driving AI development but also in addressing the ethical considerations raised by autonomous decision-making systems. These insights are still informing our current approaches, demonstrating the lasting influence of the early pioneers in this field.
John McCarthy's 1956 Dartmouth Proposal The Birth Certificate of Modern AI Patents - 10 Researchers Who Changed Computing History During The Eight Week Workshop
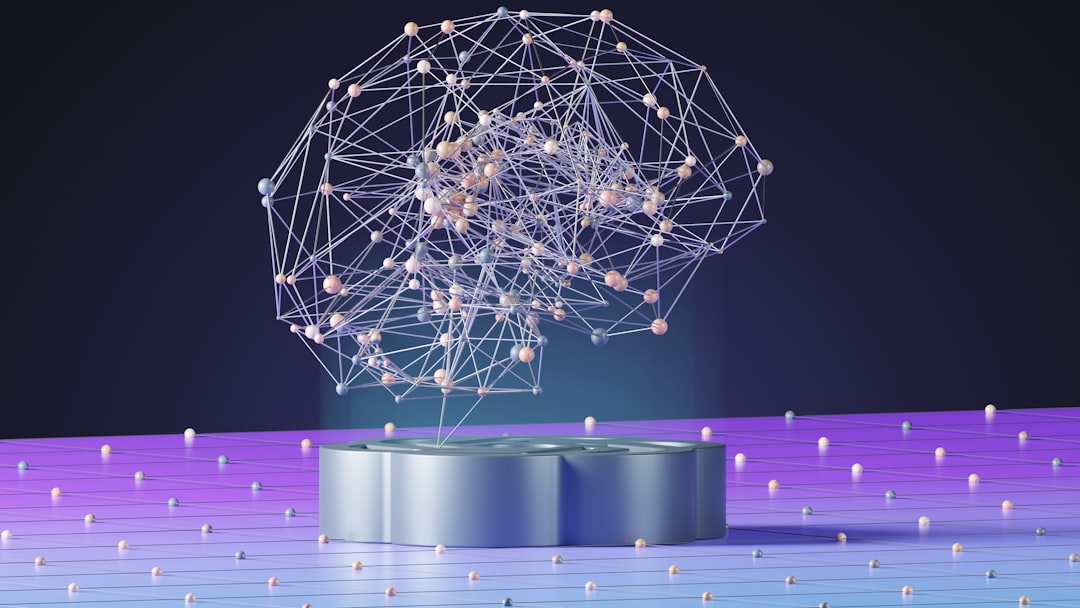
The 1956 Dartmouth Summer Research Project stands as a pivotal moment in the history of computing, serving as the birthplace of artificial intelligence as a formal field of study. This eight-week workshop brought together a remarkable group of researchers, ten of whom played particularly significant roles in shaping the future of AI. Among them were prominent figures like John McCarthy, Marvin Minsky, and Claude Shannon, whose pioneering work established core principles and theories that remain influential today.
The workshop fostered a unique environment of collaboration and open discussion, a contrast to more rigid academic settings. This informal setting proved fertile ground for groundbreaking ideas and proposals, essentially solidifying AI as its own academic discipline. This gathering, often referred to as the "Constitutional Convention of AI," not only formally introduced the term "Artificial Intelligence" but also established a template for interdisciplinary collaboration that has defined the field ever since. It was a period characterized by ambition and intellectual curiosity, and the researchers' collective efforts during those eight weeks ignited a wave of innovation that continues to shape the advancement of AI and computing as a whole. The enduring impact of the Dartmouth workshop underscores its importance in the overall timeline of AI development.
The Dartmouth Summer Research Project on Artificial Intelligence, a pivotal eight-week workshop in 1956, brought together a diverse group of researchers who significantly shaped the trajectory of computing. Their contributions were not just about programming but also about framing the very concept of artificial intelligence.
First, the gathering's interdisciplinary nature was a strength. Researchers from mathematics, psychology, and engineering found themselves at the same table, fostering unique cross-pollination of ideas. This blend helped spawn fresh ways to think about the nature of machine intelligence.
Second, the workshop was characterized by a palpable optimism. Many participants, including Marvin Minsky, boldly predicted that human-level AI would be achieved within a few decades. This projection captures both the early excitement and limitations of the nascent field's understanding at that time.
Third, the inclusion of Claude Shannon was significant. His contributions reinforced the theoretical underpinnings of information theory and helped promote statistical methods, which are essential in today's machine learning. His work added a certain level of credibility to the nascent field of AI, bringing the prestige of established fields like mathematics into the mix.
Fourth, Nathaniel Rochester’s work underlined the importance of contextual learning. He argued that machine intelligence must navigate and adapt to complex, real-world scenarios, not just solve abstract puzzles in a controlled environment. In retrospect, this viewpoint helped pave the way for a shift in AI research that sought to create systems that could interact with the 'real world.'
Fifth, John McCarthy's contributions during the workshop brought formal logic into the foreground of AI. This approach laid the groundwork for the design of future AI systems that utilized logical reasoning and heavily influenced the development of AI programming languages. The early exploration of logic's role in AI research was, in hindsight, an important decision.
Sixth, despite the breakthroughs in thinking, skepticism remained among attendees. Many questioned the feasibility of building truly intelligent machines, echoing the overall view of many academics at the time. The doubts raised by several researchers at the workshop reveal that AI did not have universal appeal even at its genesis.
Seventh, the Dartmouth workshop's discussions acted as a catalyst for cognitive science. Researchers started exploring the links between human cognition and computation, leading to future psychological research and inspiring studies on how the brain might or might not operate like computers. While the connection is debated even today, it was a fertile area of research spawned by the workshop.
Eighth, the researchers went beyond simply crafting problem-solving algorithms. They started to examine real-world decision-making, a critical step that foreshadowed the development of AI systems that could mimic human reasoning in a more nuanced way. This interest in how machines could replicate the complex process of decision-making reflected an ambition that continues to drive the field today.
Ninth, discussions at the workshop inevitably touched on ethical aspects of AI. Researchers contemplated the future ramifications of creating machines that could operate autonomously and make decisions on their own. While still a core area of AI research, the concern over 'AI ethics' emerged early in the discussions surrounding AI development.
Finally, the legacy of the Dartmouth workshop endures. The theories and practices considered at the eight-week conference continue to shape subsequent AI research. It remains a core touchstone in ongoing debates regarding the nature of human and artificial intelligence. The lasting influence of the Dartmouth workshop shows that the initial focus on understanding machine intelligence remains relevant today.
John McCarthy's 1956 Dartmouth Proposal The Birth Certificate of Modern AI Patents - From Learning Machines to Natural Language Processing The Workshop Agenda
The "From Learning Machines to Natural Language Processing" workshop exemplifies the ongoing evolution of the field of artificial intelligence, a journey that began with the 1956 Dartmouth Conference. It underscores the progress made in specific areas of AI, moving from the basic ideas behind machine learning to the more intricate aspects of natural language processing. Natural language processing requires a detailed understanding of human language and how we think, which means AI research has become increasingly intertwined with insights from psychology. The focus has shifted to understanding how machines can interpret and interact with human language, leading to the creation of advanced algorithms capable of adapting to different communication styles. However, while these developments are promising, they also bring to light complex ethical questions, concerns about how well we can understand how AI systems arrive at their results, and whether they can truly communicate in ways that mirror human communication. The topics on the workshop's agenda reflect the need for continued, careful consideration of these crucial issues, echoing the original, forward-looking discussions at Dartmouth. It's a testament to the continued relevance of the original Dartmouth workshop's goals and concerns as AI continues to develop.
The "From Learning Machines to Natural Language Processing" workshop serves as a fascinating retrospective on the foundational ideas that emerged from the Dartmouth Project. It showcases how early explorations of machine learning have branched out into specialized areas like natural language processing (NLP).
The workshop's agenda highlights a key shift in perspective. Early researchers were primarily focused on algorithmic approaches. Now, understanding the context and subtleties of human language takes center stage. There's a greater emphasis on creating models capable of grasping the nuanced aspects of human communication, rather than just focusing on syntax.
Interestingly, many of the mathematical concepts established at the Dartmouth conference, such as formal logic and probability theory, remain integral to contemporary NLP techniques. Modern NLP algorithms rely heavily on statistical methods for tasks like language generation and understanding.
The interdisciplinary spirit of the Dartmouth workshop resonates with current research. It’s evident that progress in AI, especially NLP, depends on combining knowledge from diverse fields like linguistics, psychology, and computer science. It's a clear demonstration of how insights from various areas can strengthen the pursuit of more advanced AI.
Some of the early participants in the Dartmouth AI endeavor were quite skeptical about the possibility of machines achieving human-like intelligence. That sentiment is still relevant today. NLP researchers continue to face hurdles in understanding context, meaning, and the emotional undertones present in language. It underscores that building true natural language understanding is far more complex than initially anticipated.
The transition from "learning machines" to NLP reflects a wider trend within AI. Researchers have moved away from solely computational objectives towards addressing more sophisticated tasks that involve human interaction. This fundamental goal remains a challenging pursuit.
The discussions at the Dartmouth workshop laid the groundwork for what we now recognize as "conversational AI." The idea of creating user-friendly environments that incorporated human-like conversational aspects was foreshadowed during those early days. This foresight has manifested in the development of chatbots and virtual assistants.
Ethical considerations regarding AI were already present in discussions at Dartmouth. The researchers contemplated the societal impacts of machines capable of comprehending natural language. With the widespread adoption of NLP applications, these conversations have taken on renewed importance.
One unexpected consequence of those early conversations is the realization that effective NLP necessitates an understanding of cultural context. This introduces a significant layer of complexity to algorithm design, requiring researchers to account for diverse language use and social norms.
The workshop's exploration of the intersection of machine learning and NLP provides a contemporary lens through which to view some of the challenges initially presented at Dartmouth. It serves as a reminder that potential biases in training data remain a key concern, echoing earlier debates about the ethical applications of AI.
John McCarthy's 1956 Dartmouth Proposal The Birth Certificate of Modern AI Patents - Rochester Shannon Minsky and McCarthy Four Authors One Revolutionary Document
The 1956 Dartmouth Summer Research Project, spearheaded by John McCarthy, Claude Shannon, Marvin Minsky, and Nathaniel Rochester, marked a turning point for artificial intelligence and highlighted the power of interdisciplinary collaboration. This seminal event, frequently dubbed the "Constitutional Convention of AI," solidified the field's identity by formally introducing the term "Artificial Intelligence" and setting out fundamental principles for the research that would follow. The workshops not only focused on solving practical AI problems but also initiated conversations about the potential ethical and societal impacts of advanced machines, topics that remain at the forefront of modern AI discussions. The initial vision of these four researchers fostered the growth of a robust research community, balancing both theoretical investigations and practical implementations of AI technologies. Their pioneering efforts illustrate how the fusion of diverse fields can deepen our comprehension of intelligence, whether it's human or artificial. This collaborative approach remains crucial as AI continues to evolve and its influence expands.
The Dartmouth Summer Research Project on Artificial Intelligence, conceived in 1955 and held in the summer of 1956, represents a crucial moment in the development of this field. The proposal, authored by John McCarthy, Marvin Minsky, Nathaniel Rochester, and Claude Shannon, is often viewed as AI's "birth certificate." It outlined a two-month study with a small team of ten researchers focused on advancing the then-fledgling discipline.
Interestingly, this initiative brought together a diverse range of minds from various disciplines. The collaboration of computer scientists, psychologists, and engineers was a defining characteristic of the workshop, and it underscored the inherent complexity of the field. It highlighted a realization that achieving breakthroughs in AI would require perspectives from several areas of expertise. The early emphasis on interdisciplinary collaboration, particularly with psychology, proved remarkably prescient, setting the tone for subsequent research.
While the notion of "neural networks" wasn't explicitly stated in the proposal, the discussions regarding learning machines implicitly touched upon foundational concepts later formalized within neural network theory. The project's early explorations hinted at the potential for machines to adapt and learn through experience, an idea that formed a cornerstone for much of AI's development.
John McCarthy's emphasis on logic and formal reasoning proved instrumental in shaping the field. His insights not only helped to foster the development of programming languages like Lisp, specifically crafted for AI, but also contributed to the field of formal verification which is now used to assess the reliability and security of sophisticated AI systems.
Although the researchers were enthusiastic, a degree of skepticism regarding the potential for attaining human-level intelligence within a short timeframe was present among the participants. This tension between optimistic goals and the limitations of available technologies remains a central theme in the ongoing development of AI.
The Dartmouth workshop had a significant influence on the emergence of cognitive science as a distinct field of study. The discussions at Dartmouth encouraged researchers to explore the connection between human cognitive processes and computational models, stimulating a line of inquiry that continues to be relevant within both AI and psychology.
Interestingly, discussions at Dartmouth foreshadowed contemporary concerns about the ethics of AI. Researchers pondered the potential societal impact of machines capable of making autonomous decisions. This early acknowledgment of ethical issues relating to AI set the stage for the widespread debate that surrounds this topic today.
Many currently-used techniques in Natural Language Processing can be traced back to the discussions at Dartmouth. The early exploration of concepts relating to language understanding laid the foundation for the sophisticated algorithms that power modern language models.
Nathaniel Rochester's argument that machines needed to navigate the complexities of real-world scenarios signaled a crucial shift in focus from overly abstract problems. This viewpoint helped pave the way for AI systems that can interact with and adapt to the messiness of the real world, rather than only abstract or idealized problems.
Surprisingly, even early on, researchers acknowledged that understanding cultural contexts was vital for machine learning. The proposal hinted at the idea that language and communication are influenced by social norms and cultural traditions, a concept that has become increasingly important in AI research as algorithms are developed and deployed in a variety of social contexts.
The Dartmouth workshop's emphasis on human-like decision-making helped launch discussions on human cognitive biases in algorithmic design. Researchers began to think about how cognitive biases could affect the development and use of AI systems, setting the stage for research into the fairness and transparency of AI algorithms.
The Dartmouth Proposal, though written nearly 75 years ago, remains a crucial artifact in the history of AI. Its influence on the field’s development continues to be felt in diverse areas of contemporary research. The careful consideration of the past can help guide the future development of AI and ensure that the field continues to address the challenges and opportunities that its evolution presents.
More Posts from patentreviewpro.com: