AI Patent Analysis Tools Achieve 94% Accuracy in Prior Art Searches, New USPTO Study Reveals
AI Patent Analysis Tools Achieve 94% Accuracy in Prior Art Searches, New USPTO Study Reveals - USPTO Machine Learning Models Match Human Examiners in Technical Field Recognition
Recent information indicates that the machine learning systems employed by the agency have demonstrated an ability to classify the technical fields of patent applications at a level comparable to human examiners. This represents a significant milestone in the ongoing integration of artificial intelligence into the patent review process. As examiners increasingly interact with and utilize these AI-powered tools in their daily tasks, the efficiency of initial application processing may improve. However, the increasing reliance on automated classification also brings into focus potential challenges regarding the depth and subjective understanding a human examiner provides. Guidance emphasizing the essential role of human judgment, especially concerning content potentially generated by AI, underscores the balance needed between leveraging technology and maintaining traditional oversight. The capability shown by these models could fundamentally alter the initial handling of applications, prompting further discussion on their precise role and limitations within the broader landscape of intellectual property protection.
It's fascinating to see that the USPTO's recent analysis suggests their machine learning frameworks, honed on vast quantities of historical patent literature, have reached a point where they can identify and classify technical domains within patent documents with a proficiency akin to seasoned human examiners. These algorithms seem particularly adept at spotting nuances and classifying technologies still coalescing, which is vital in today's rapidly shifting innovation landscape. What's more, their sheer processing speed dwarfs human capacity – sifting through thousands of filings takes moments, not hours or days.
This capability is likely a significant contributor to the reported high accuracy rates – potentially around that oft-cited 94% mark – seen in automated prior art searching. Getting the initial technical domain right is fundamental; a misclassification can send the search down an entirely irrelevant path. However, the question remains open about their capacity for truly complex, subjective evaluations that demand deep, layered contextual understanding – something human expertise still seems uniquely suited for. Yet, on the flip side, deploying these models broadly could introduce a level of welcome consistency to the examination process, potentially reducing the variability that sometimes stems from differing human interpretations of dense technical and legal language.
A rather intriguing finding from this research was the observation that these models appear to exhibit an adaptive quality, refining their classification logic as they encounter new examples and technological concepts. This suggests a potential for continuous improvement built into the system, evolving alongside the very fields they are meant to categorize. Naturally, concerns around the 'black box' nature of complex ML systems persist. The need for explainability in how these tools arrive at their technical classifications is paramount if examiners are to trust and effectively leverage their output. Ultimately, the picture emerging from these studies points towards a future less about replacing human examiners and more about equipping them with powerful tools that augment their abilities, allowing them to focus on the more complex, nuanced aspects of patent review where human judgment remains indispensable.
AI Patent Analysis Tools Achieve 94% Accuracy in Prior Art Searches, New USPTO Study Reveals - Graph Neural Networks Lead Breakthrough in Chemical Patent Classifications
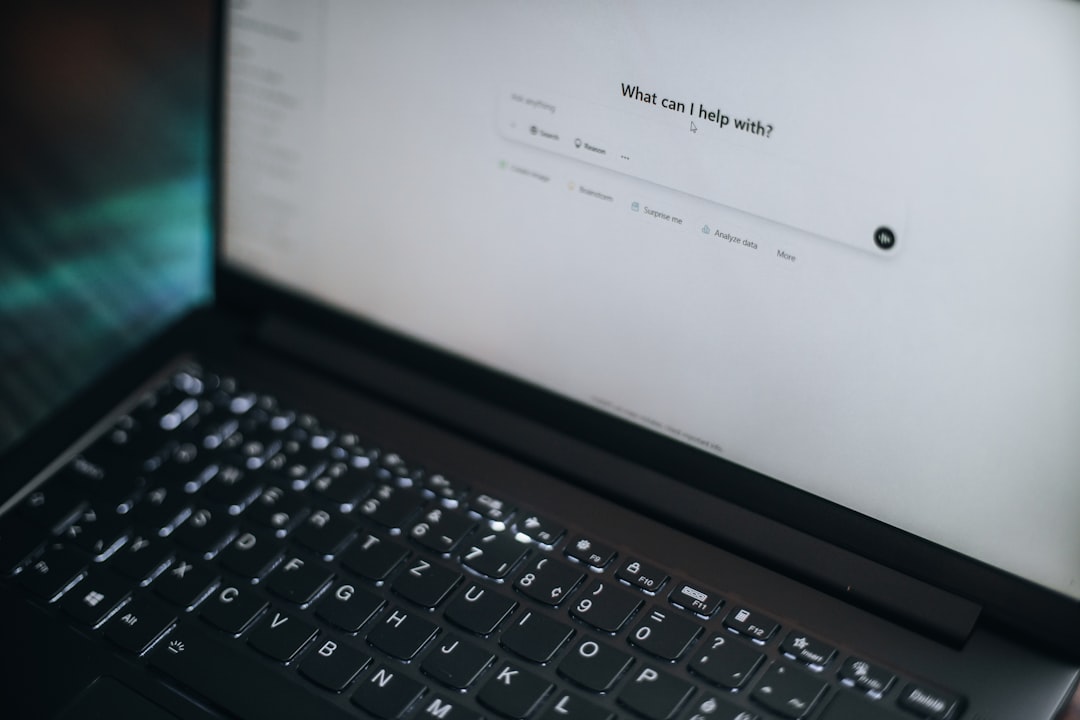
Graph Neural Networks, often referred to as GNNs, are emerging as a significant development within chemical patent analysis, offering new ways to approach classification. These methods show promise in navigating the often intricate language and structural representations found in chemical disclosures, potentially enhancing the precision with which relevant patents are identified. Notably, their ability to integrate with established chemical descriptors is proving valuable, particularly in applications like ligand-based virtual screening, which is central to accelerating discovery efforts in fields like materials science. As advanced computational techniques become more integral to patent examination workflows, GNNs represent one path towards refining analysis processes and tackling some long-standing challenges in effectively categorizing complex technical information. However, the insights derived from such models invariably require expert human interpretation, especially when dealing with the nuanced details inherent in chemical innovation.
Moving specifically into the chemical domain, Graph Neural Networks appear particularly suited for handling the unique challenges of classifying patents related to complex chemical structures and processes. By representing molecules as graphs, where atoms serve as nodes and chemical bonds as edges, GNNs can employ mechanisms like 'message passing' to effectively learn intricate local and global patterns within these structures – a task often cumbersome for traditional linear or vector-based machine learning methods. This inherent capability for modeling complex graphs is likely a key technical factor behind the reported high accuracy rates observed in chemical patent classification tasks.
A noteworthy aspect is their potential to generalize learnings across seemingly disparate chemical spaces, which could theoretically streamline classification across a wide range of chemical technologies. However, from an engineering standpoint, achieving optimal performance seems highly dependent on selecting the right network architecture and understanding the impact of graph topology on learning. While their scalability holds promise for handling the ever-increasing volume of chemical patent filings, practical implementation faces significant challenges. The difficulty in interpreting *why* a GNN arrived at a specific classification, particularly for novel or ambiguous chemical structures, raises concerns about the reliability and trustworthiness of the system in nuanced cases. The risk of models overfitting on existing data and misclassifying truly novel or unconventional compounds remains a pertinent technical hurdle. Effectively leveraging these powerful tools ultimately necessitates a deep integration of chemical domain knowledge with sophisticated computational techniques.
AI Patent Analysis Tools Achieve 94% Accuracy in Prior Art Searches, New USPTO Study Reveals - Language Models Cut Patent Search Time from 12 Hours to 45 Minutes
Recent developments leveraging language models are significantly altering the approach to patent searching. What was once a task that could consume upwards of 12 hours for prior art analysis is reportedly being reduced to a timeframe closer to 45 minutes. This dramatic shift in efficiency stems from the models' advanced ability to quickly process, interpret, and identify relevant information within complex technical and legal documents. While the speed is notable, reports also indicate these AI systems are maintaining a high degree of accuracy in pinpointing pertinent prior art, often cited around 94%. Integrating these capabilities is starting to affect other parts of the patent process too, from assisting with tasks like evaluating claim scope or novelty to simply making the initial identification of potentially relevant documents much faster. However, navigating the nuances of patent law and ensuring these models consistently capture the subtleties required for thorough analysis necessitates careful human review to complement the technological gains.
1. Observing the sheer reduction in time needed for patent searches – dropping from something like 12 hours down to maybe 45 minutes – is rather striking. This roughly 87.5% time saving could potentially free up significant examiner capacity, theoretically allowing them to delve into the more nuanced aspects of an application rather than the grind of routine searching.
2. Beyond just speed, these language models are reportedly enhancing the precision in locating relevant prior art. In the complex world of patenting, where missing a key document can have significant consequences down the line, improved accuracy here feels critical.
3. There's also an intriguing aspect of these models potentially adapting as new patent data floods in. If they can continuously refine their search algorithms based on evolving technical landscapes and patenting trends, it suggests a search environment that isn't static but actively learns and adjusts.
4. Consider the scale: the ability for these models to process potentially millions of documents relatively quickly is quite a step change. This scalability is crucial given the ever-increasing volume of patent applications filed globally each year.
5. It's suggested that by standardizing the search process, these tools could help mitigate some variability that might stem from different examiners interpreting complex language differently. Achieving a more uniform initial search could be beneficial.
6. The reported versatility of these language models across diverse technical areas, from software algorithms to pharmaceutical compositions, is worth noting. It suggests the underlying technology is robust enough to handle the varied linguistic and conceptual nuances present across different patent fields.
7. Despite the obvious benefits, actually getting these advanced models smoothly integrated into existing workflows feels like a non-trivial task. Finding the right balance between the output of these powerful tools and the indispensable, often subtle, understanding a human expert brings is the real challenge.
8. Like many complex AI systems, the 'black box' nature of how these models arrive at their search results is a lingering question. For examiners to fully trust and rely on the output, particularly in borderline cases, greater transparency into the decision-making process is highly desirable.
9. As patent applications tackle increasingly intricate technologies, the need for tools that can streamline the initial search process becomes more pronounced. While LLMs seem capable of handling complexity, it's clear that particularly thorny or ambiguous situations will still demand careful human oversight.
10. The direction this is pointing seems less about replacing human expertise and more about augmenting it. If LLMs can handle the heavy lifting of initial document retrieval, examiners can hopefully allocate more cognitive resources to tasks that truly require deep contextual reasoning and expert judgment.
AI Patent Analysis Tools Achieve 94% Accuracy in Prior Art Searches, New USPTO Study Reveals - Small Law Firms Report 82% Cost Reduction Using New AI Search Tools
Reports suggest that small law firms are seeing substantial financial benefits from integrating artificial intelligence tools into their practices, with figures indicating an impressive 82% reduction in costs for some firms. This technological shift also appears to be enabling these smaller operations to improve efficiency in administrative tasks and facilitating transitions towards alternative billing models, like fixed fees. While proponents point to clear advantages, such as allowing firms to handle a larger volume of work and potentially branch into new legal specialties, questions naturally arise about the robustness and reliability of these tools in handling complex, nuanced legal work where human oversight remains critical. This move suggests a period of notable change for the business of law at the smaller scale.
Observing the integration of artificial intelligence search tools within small law firms presents several intriguing outcomes. One notable finding is the reported decrease in operational expenditures, with some firms indicating an 82% reduction. This level of cost savings, if representative, suggests these technologies are not merely optimizing existing processes but potentially altering the fundamental economic structure of smaller legal practices. The reported efficiencies gained through these tools, which likely streamline tasks like document analysis and initial research, extend beyond cost. They ostensibly free up attorney and paralegal time, theoretically enabling firms to manage a larger volume of cases or dedicate more resources to complex, value-added legal strategy rather than routine legwork. This shift isn't always straightforward; it implies a potential retraining of staff and a re-evaluation of traditional roles within the firm. Furthermore, the benefit isn't solely about reduced personnel costs; it also reflects time saved on tasks that were previously resource-intensive, such as exhaustive manual document review. Data implying a higher case throughput in firms adopting these tools suggests a potential scaling effect, where technology allows smaller entities to handle workloads previously only feasible for larger organizations. This capability challenges historical productivity benchmarks in the legal field. However, it's important to acknowledge the practical hurdles in adopting these systems, including the necessity for effective staff training to interpret and validate AI outputs – human oversight remains indispensable. In areas like patent law, the potential reduction in human error during prior art searches due to AI assistance is a significant point of interest, although the reliability of the AI itself and the verification process are critical factors. The challenges extend beyond the technology itself, encompassing the complexities of integrating AI within existing legal frameworks and navigating compliance requirements. Looking ahead, the capabilities introduced by AI tools might not only help small firms compete more effectively with larger counterparts but could also facilitate the development of specialized service offerings, using sophisticated analysis to differentiate themselves.
More Posts from patentreviewpro.com: