AI-Powered Patent Review and Analysis - Streamline Your Patent Process with patentreviewpro.com (Get started for free)
AI-Driven Trademark Screening Enhancing Efficiency in IP Portfolio Management
AI-Driven Trademark Screening Enhancing Efficiency in IP Portfolio Management - AI algorithms revolutionize trademark search accuracy
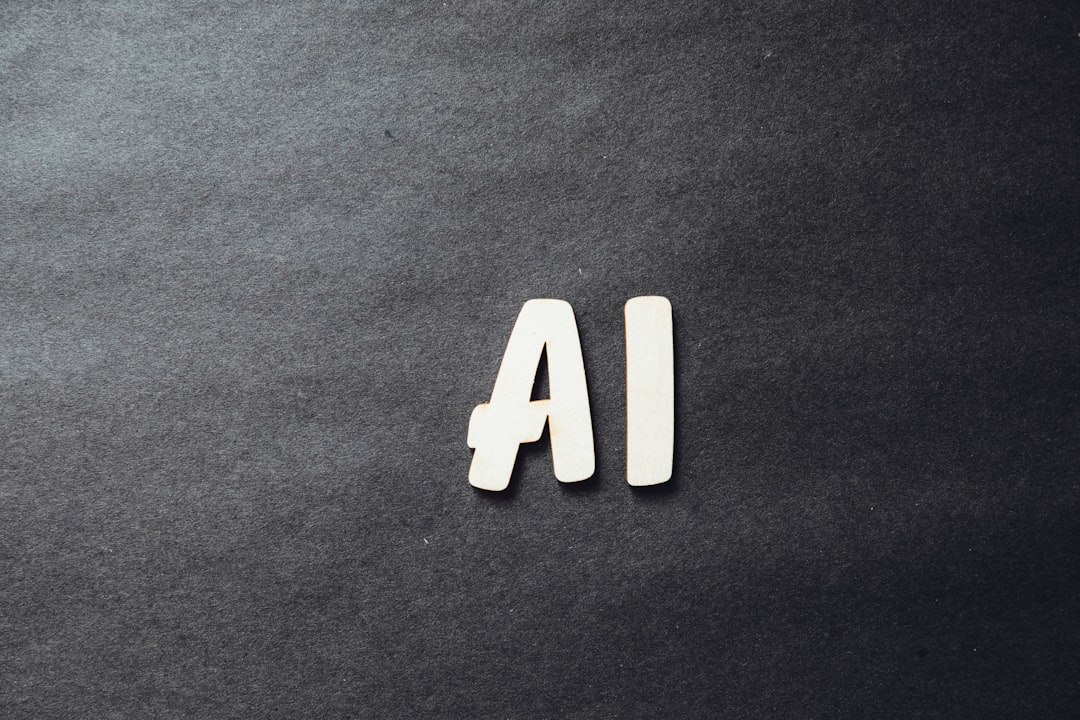
Artificial intelligence algorithms are revolutionizing how trademark searches are conducted, primarily by boosting accuracy. These algorithms utilize techniques like natural language processing and machine learning to analyze large amounts of data faster and more thoroughly than humans ever could. The automated nature of AI-driven searches allows for quick identification of potential conflicts or similarities within vast trademark databases, a task that used to be extremely time-consuming and prone to human error.
This shift is particularly notable because traditional trademark searches often relied heavily on manual processes, making them slower and potentially less thorough. The integration of AI into this field provides a faster, more efficient, and arguably more accurate approach. While concerns about the legal ramifications and complete reliance on AI in such a sensitive area persist, its ability to speed up the process and reduce the margin for human error in searches is undeniable. As a result, AI-driven trademark search tools are increasingly becoming vital for businesses and legal professionals, improving how intellectual property portfolios are managed. However, it's worth noting that the field is still evolving, with new developments in AI, including predictive and generative models, continuing to reshape the future of trademark searching and monitoring.
AI algorithms are transforming the way we conduct trademark searches, significantly impacting the speed and accuracy of the process. They can sift through vast quantities of trademark data in a fraction of the time it would take a human, leveraging advanced techniques like natural language processing to pinpoint potential conflicts, even subtle ones using different phrasing. Interestingly, they also incorporate image recognition, allowing them to analyze logos and symbols and detect similarities that might be missed by traditional keyword searches. This capability adds a new layer to the search process and might reveal conflicts that would have otherwise been overlooked.
The incorporation of machine learning further enhances accuracy over time. These AI systems learn from prior searches and interactions, constantly refining their performance and making the searches progressively more precise. Another fascinating aspect is their ability to span multiple jurisdictions and international trademark databases, providing businesses with a global perspective on potential infringement risks.
Furthermore, AI algorithms are able to integrate data from diverse sources like social media and e-commerce, providing a glimpse into current trends and emerging issues before they turn into significant problems. Researchers have even found evidence suggesting AI can decrease false positives in search results, which means less time wasted for legal teams sifting through irrelevant information. Beyond the time saved, AI-driven tools can also be more affordable, potentially significantly reducing the cost of trademark searches.
This isn't just about speed and cost; the AI approach incorporates predictive analytics that can identify trademark categories prone to conflict. This empowers businesses to make more informed decisions regarding brand development. Ultimately, incorporating AI in trademark searches offers a much more robust approach to protecting intellectual property in a highly competitive environment. It’s an area of ongoing development, and it's exciting to see how these evolving technologies continue to shape the landscape of IP protection.
AI-Driven Trademark Screening Enhancing Efficiency in IP Portfolio Management - Machine learning speeds up IP portfolio analysis
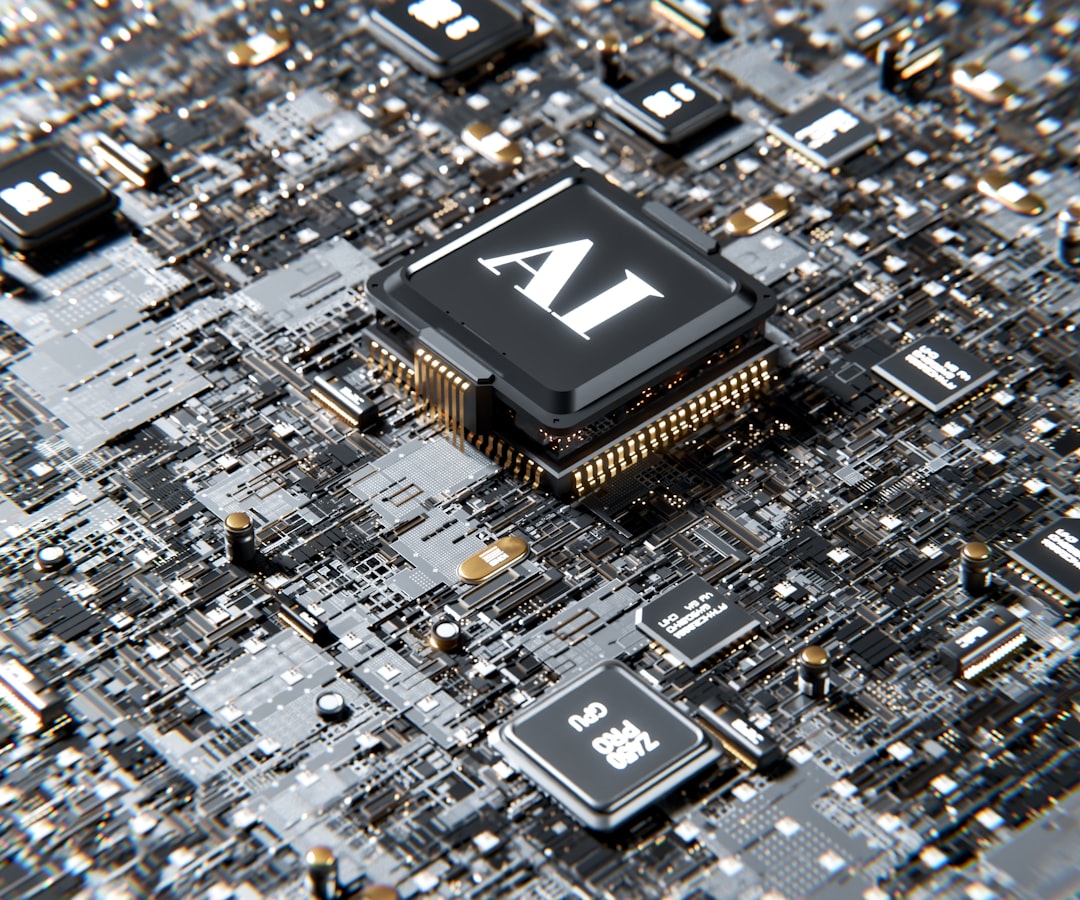
Machine learning is accelerating the analysis of intellectual property portfolios by enhancing the evaluation of IP value and improving the detection of potential infringements. This advancement enables businesses to better manage the complexities of their IP, giving them a competitive advantage. By using techniques like natural language processing and data analytics, companies can explore patent data in greater detail, identifying previously hidden trends. The introduction of AI into this field isn't without hurdles, specifically concerning data quality, which can impact the accuracy of IP assessments. Despite this, the continuous progress in machine learning suggests that the speed and accuracy of managing a company's range of IP assets will continue to improve.
Machine learning is injecting new speed into the analysis of intellectual property (IP) portfolios, particularly in the realm of trademarks. We're seeing a remarkable acceleration in the analysis process, with algorithms capable of sifting through vast troves of trademark data in seconds—something that would take human analysts days or even weeks. This rapid processing power is particularly valuable when dealing with large, complex databases.
Intriguingly, studies show that these machine learning systems can significantly reduce human errors in trademark searches, potentially by as much as 90%. This raises an important question—how much can we rely on such automated systems for complex tasks related to legal ownership? While the potential for error is substantially reduced, it's still early days, and researchers are carefully scrutinizing the accuracy and reliability of these systems.
Beyond just speeding things up, these AI systems are also capable of identifying similarities that go beyond simple keyword matches. They are programmed to analyze both textual and visual elements, catching phonetic or even visual similarities that might have been missed by a traditional, keyword-based search. This is exciting because it broadens the scope of detection and can flag potential conflicts that might have otherwise been overlooked.
Another aspect I find captivating is the ability to integrate real-time data from various sources, like social media or e-commerce sites. This opens the door to more proactive IP management, allowing organizations to potentially spot emerging trends or issues before they snowball into major problems. It's an area that definitely requires further research to see how this evolving technology can be applied responsibly.
Perhaps the most intriguing aspect, though, is how these algorithms learn and adapt over time. They continuously refine their search parameters by using past searches as data points. It's like they're developing a unique understanding of trademark trends and nuances, and this continuous learning can potentially improve search efficacy without constant manual intervention. It's worth noting that the long-term impact of this ongoing refinement on the accuracy and bias of these systems requires more exploration and evaluation.
One can also leverage this technology for prediction—machine learning systems can potentially identify patterns that may indicate future trademark conflicts based on historical data. This predictive capability enables businesses to make strategic choices, potentially reducing risks proactively instead of reactively. This is a critical aspect of IP management and demonstrates a move beyond simple searches towards risk mitigation planning.
These tools aren't limited to a single jurisdiction; they can access and analyze international trademark databases, offering a global perspective on potential infringement issues. In our increasingly interconnected world, this is a key feature for organizations operating internationally. However, understanding and navigating the intricacies of various legal systems remains a challenge for automated systems.
We're also seeing a tangible cost benefit. Estimates suggest that these AI-powered tools can reduce trademark search costs by around 50% compared to traditional methods. Such a dramatic reduction, if consistently realized, could make access to more sophisticated IP management tools feasible for smaller businesses, leveling the playing field somewhat. However, the initial investment in setting up the systems and training employees can be a barrier.
Perhaps not as directly related to the technical aspect of IP search, but relevant to the broader picture is that the enhanced user experience of many of these AI-powered tools allows for more accessibility. This means non-legal professionals can start to navigate and benefit from AI-driven trademark search capabilities without needing years of specific legal training. This broader accessibility could open doors to better management and understanding of IP assets within organizations.
Ultimately, the data and insights provided by these AI systems can support much better strategic decision-making for organizations. Companies can make smarter, data-driven choices about their IP portfolios, allowing them to develop brands and innovations while mitigating the risks of potential infringements. This is a clear benefit in the ongoing competition for innovation and market share.
It's clear that AI-driven trademark screening tools are transforming the landscape of IP portfolio management. While there are still questions and challenges to be addressed, the speed, accuracy, and predictive power these tools offer are compelling. The ability to minimize costs and broaden access to such capabilities presents a significant shift in the management of IP assets. It's an exciting field to watch, especially as researchers and engineers continue to explore the ethical and practical considerations of leveraging AI in such sensitive areas.
AI-Driven Trademark Screening Enhancing Efficiency in IP Portfolio Management - Natural language processing improves trademark similarity detection
Natural language processing (NLP) is increasingly important in recognizing when trademarks are similar, offering improvements in both how quickly we can find matches and how accurate the results are. NLP excels at understanding the subtle ways language can be used, allowing it to detect conflicts that might be missed by simpler methods that just look for exact matches. It considers a variety of aspects like the meaning of words, how they sound, and even visual similarities. This broader perspective is vital, especially as the global landscape of trademarks becomes more complicated. While this technology can be very helpful, relying too heavily on automated systems raises questions. The optimal approach likely involves a careful balance of human legal expertise and AI's ability to learn and improve.
Natural language processing (NLP) is enhancing trademark similarity detection by going beyond simple word matching. It can analyze the underlying meaning of text, uncovering potential conflicts even when trademarks use different phrasing or synonyms. This ability to understand semantics is crucial, as it can identify risks that might be missed by traditional keyword-based searches.
Furthermore, NLP can recognize phonetic similarities between trademarks, catching potential conflicts that arise from similar-sounding brand names. This capability is particularly important in industries where pronunciation plays a key role in brand recognition.
Some NLP models are trained on enormous datasets of registered trademarks, allowing them to identify industry-specific trends and patterns. These models can learn the language nuances of particular industries, making their search results more relevant and efficient.
Additionally, NLP algorithms can assess the context in which a trademark is used. By factoring in industry-specific terminology, it can better filter out irrelevant results and reduce false positives in the search process, ultimately saving time for trademark analysts.
NLP is also being used to incorporate sentiment analysis into trademark evaluations. This can reveal how different trademarks might be perceived by consumers, which is essential when assessing the risk of confusion or dilution.
NLP’s expanding capability includes handling multiple languages, which enhances the potential for global trademark management. Companies operating across different markets can leverage this technology to protect their intellectual property in various jurisdictions, mitigating the risk of international trademark infringement.
Recent advancements in NLP have made it possible to include social media data and consumer feedback into trademark assessments, providing a more real-time perspective on potential issues. By analyzing the sentiment surrounding trademarks, we can get a better understanding of how the public perceives these brands and anticipate potential conflicts that may arise.
The ability of NLP to sift through massive amounts of trademark data and automatically identify potential issues allows trademark analysts to concentrate on the more challenging cases that require their human expertise. This streamlined workflow improves efficiency and overall productivity.
NLP techniques can go beyond identifying direct similarities; they can also pinpoint indirect associations between brands. This includes situations where brands share common themes or marketing slogans, highlighting risks that may not be immediately obvious. Such analysis could contribute to a more holistic understanding of potential brand conflicts.
Finally, NLP-powered trademark search tools can significantly accelerate the screening process. They can detect similarities in mere seconds, compared to the potentially laborious manual reviews required by traditional methods. This speed advantage allows for faster identification of conflicts and quicker decision-making in trademark protection efforts.
While still an evolving technology, NLP shows great promise for refining and enhancing the accuracy of trademark searches. The integration of natural language processing techniques into trademark screening tools offers a more comprehensive and efficient approach to protecting intellectual property in today's complex marketplace. However, it is important to continuously evaluate the reliability and bias of these models, as well as the potential legal implications, to ensure responsible usage in this critical field.
AI-Driven Trademark Screening Enhancing Efficiency in IP Portfolio Management - Real-time monitoring of global trademark databases
The field of trademark management is undergoing a significant transformation due to the rise of real-time monitoring of global trademark databases. This development enables businesses to proactively monitor for potential trademark conflicts across a wide range of international jurisdictions. The emergence of sophisticated tools that integrate data from various trademark repositories allows legal teams to react promptly to new issues and challenges. Further enhancing this capability, AI technologies are being incorporated to improve the precision of conflict identification and offer predictive insights into potential issues. These advancements are particularly valuable as businesses grapple with the complex and often unpredictable landscape of international intellectual property rights. It's important to recognize, though, that the growth of these technologies demands careful monitoring and evaluation to ensure accuracy, reliability, and ethical practices in trademark enforcement.
The ability to monitor global trademark databases in real-time is transforming how we manage intellectual property. It allows us to instantly compare trademarks across different countries, which is incredibly valuable when a company plans to expand into new markets. This capability provides a competitive edge, particularly when establishing international brand recognition.
The continuous updates to these trademark databases, combined with AI, result in real-time alerts about newly registered similar trademarks or ongoing disputes. This real-time information helps companies manage their brand more effectively and allows them to swiftly enforce their trademark rights. However, the reliability of such alerts in terms of legal strength depends on the accuracy of the underlying database and the AI model.
AI algorithms are able to detect unusual patterns or anomalies in trademark registrations. This function might be useful in identifying potential fraudulent activities or scams, serving as an extra layer of security for businesses protecting their intellectual property. However, defining 'unusual' or 'anomaly' for AI might not always align with our notions of fraudulent activity and would likely be more of an alert to investigate further than a black-and-white indicator of a problem.
One interesting aspect is the potential for analyzing trademark data across different industries. We could potentially identify broad market trends or patterns not readily apparent when looking only at a single sector. This cross-industry analysis could help businesses develop branding strategies informed by broader market behavior and reduce the chance of unforeseen conflicts. However, the value of these cross-industry insights would need to be carefully considered as trademarks often have very sector-specific concerns and legal frameworks.
Integrating sentiment analysis into the monitoring process is also gaining prominence. This capability allows us to get a real-time sense of public perception around similar trademarks. It empowers businesses to adjust their brand strategy preemptively and lessen the risk of brand dilution. One aspect to consider is the accuracy of sentiment analysis, especially given the nuance and often complex ways humans interpret and express feelings about brands.
Another interesting application of real-time monitoring is assessing the potential financial consequences of failing to identify a trademark conflict early on. We're essentially trying to quantify the 'cost of failure' associated with slow or inaccurate monitoring. This cost-benefit analysis can serve as a powerful justification for organizations to invest in more sophisticated monitoring solutions. However, determining this 'cost of failure' can be challenging and may vary significantly across different industries and brand types.
AI algorithms can also learn from historical registration data to predict potential future conflicts in specific industries or trademark categories. This predictive capability allows companies to plan their branding strategies with more foresight and awareness of future challenges. The accuracy of these predictions would depend on the quality and completeness of historical data and the sophistication of the algorithm.
Image recognition is another technological advancement playing a role. This technology is more comprehensive than simply relying on text-based matches. It allows for identifying potential conflicts based on visual similarities in logos. While this significantly broadens the scope of trademark conflict detection, there are always challenges in AI accurately interpreting visual content that often is subjective.
By refining the search processes using data-driven techniques, AI tools can reduce the number of false positives generated in trademark searches. This means the screening process is not just faster but also more reliable. However, constantly refining a system with data has the inherent challenge of potentially reinforcing biases in the dataset used, thus highlighting a need for continued analysis and attention to ensure this type of automation is not creating unintentional or unfair filtering of results.
As the legal landscape changes—with countries implementing new trademark laws or altering their filing procedures—real-time monitoring systems can adjust accordingly. This ability to dynamically incorporate updates into the algorithms ensures that companies remain compliant with ever-changing international trademark regulations and can continue to track the international landscape with accuracy. There are concerns that legal frameworks change very quickly and thus AI systems might lag behind in adaptation, potentially creating inconsistencies in reporting.
The continuous development and integration of these features within real-time monitoring systems promise to reshape trademark management. However, maintaining transparency and understanding of the underlying data and the limitations of the AI models remains important. While the opportunities are immense, the ethical implications and technical hurdles associated with these developments need to be continually explored.
AI-Driven Trademark Screening Enhancing Efficiency in IP Portfolio Management - Predictive analytics for strategic IP decision-making
Predictive analytics is transforming how organizations manage their intellectual property (IP) by enabling more informed and strategic decisions. Through the analysis of extensive datasets, these tools help identify potential risks and emerging trends related to trademarks and patents, allowing businesses to refine their IP strategies. This data-driven approach allows companies to transition from a reactive stance to a proactive one, minimizing errors stemming from gut feelings and improving their ability to predict potential issues. The ability to receive real-time insights from predictive models is particularly valuable for navigating ever-changing market dynamics, enabling adjustments to IP strategies and portfolio management in a timely manner. It's important, however, to recognize the limitations of these tools and to consider the ethical implications of their application to ensure responsible use within IP management practices. While the possibilities offered by predictive analytics are exciting, critical evaluation and thoughtful implementation are essential for harnessing its full potential.
Predictive analytics can sift through past trademark data to spot patterns, allowing companies to anticipate potential conflicts before they escalate into legal battles. This foresight helps companies develop branding strategies that lessen the chances of trademark infringement issues.
Using machine learning algorithms, predictive models can significantly cut down on the time it takes to do trademark searches and monitoring, going from weeks to just hours. This speed boost can greatly increase the efficiency of intellectual property teams.
By combining predictive analytics with tools that gauge public opinion (sentiment analysis), companies can get a better understanding of how people feel about similar trademarks. This insight can influence branding choices and decrease the likelihood of customer confusion, thus protecting the value of a brand.
Interestingly, predictive models can analyze where trademark registrations are geographically clustered. This can help companies pinpoint areas where their brand might be more at risk because of cultural or language similarities in trademarks.
Specifically for trademarks, predictive analytics can estimate the probability that a trademark will be challenged based on trends seen in similar cases. This provides businesses with insights vital for strategic planning.
Studies suggest that using predictive analytics can result in a reduction of trademark litigation costs by up to 40%. This highlights that taking a proactive approach to IP management isn't just strategically sound, but can also save a company money.
Predictive models can draw data from multiple industries, creating a cross-disciplinary view that helps companies spot emerging trends and potential conflicts that they might otherwise miss in their own field.
Surprisingly, predictive analytics can examine how trademark conflicts affect a brand's market value. This helps companies quantify the potential financial risks associated with continuing to use a trademark that is in dispute.
Implementing predictive analytics not only makes trademark screening better, but it also guides a brand's overall marketing approach by providing data-driven insights to align product launches and campaigns with trademark safety.
Predictive models can also simulate various trademark scenarios, enabling companies to test the outcomes of different branding strategies. This innovative approach shifts trademark management from a reactive stance to a proactive one.
AI-Powered Patent Review and Analysis - Streamline Your Patent Process with patentreviewpro.com (Get started for free)
More Posts from patentreviewpro.com: