AI-Driven Insights: Redefining Patent Review and IP Strategy
AI-Driven Insights: Redefining Patent Review and IP Strategy - Shifting daily tasks in patent review work
The practical realities of patent review work are undeniably changing as intelligent systems become more prevalent. As of May 2025, the integration of AI is less about outright replacement and more about fundamentally altering the granular tasks performed daily. IP professionals are spending less time on the exhaustive manual sorting and initial analysis of massive patent and literature databases, leveraging algorithmic capabilities for swift preliminary screening. This shift necessitates maintaining a sharp focus on human oversight for the nuanced interpretation and critical evaluation that remains beyond current AI capabilities. The acceleration isn't just in search; AI is also impacting how drafts are reviewed and claims are initially assessed, potentially flagging relevant prior art or inconsistencies with remarkable speed. While promising significant time savings and facilitating a more data-aware strategic approach, particularly in aligning legal views with R&D output, the dependency on these tools also raises questions about the potential for over-reliance and the need to continuously train human expertise to interact effectively with AI outputs, rather than passively accept them. This evolution demands a constant adaptation of workflows and skill sets.
Here are some observations on how daily patent review tasks appear to be evolving with the integration of computational tools leveraging artificial intelligence:
1. Given AI's demonstrated capacity to rapidly process and sift through vast technical document repositories, the prospect emerges that those involved in examining patent applications might have bandwidth freed up. This could potentially allow them to dedicate more cognitive effort to the trickier, more subjective evaluations inherent in assessing true novelty and the subtle nuances of inventive step (or obviousness), rather than getting bogged down in initial searching and categorization. Whether this directly translates to consistently higher quality examination outcomes remains a subject of ongoing evaluation.
2. Integrating these AI systems into the daily routine isn't just a matter of adopting new software; it seems to necessitate a change in the human skill set. There's an increasing requirement for patent professionals to develop familiarity with concepts from data analysis and understand how AI tools arrive at their conclusions (interpretability). This suggests a transition for some towards becoming practitioners with blended technical-legal expertise, rather than purely traditional legal navigators.
3. While automated systems are becoming remarkably efficient at identifying potentially relevant prior technical disclosures at speed, the crucial, layered process of interpreting this material within a legal framework and making the final, often subjective judgment on inventiveness continues to reside with human experts. The role of this "human-in-the-loop," providing the ultimate legal and technical interpretation and evaluation, appears to be taking on an even more decisive role as it represents the final validation stage after the initial AI filtering.
4. The enhanced operational efficiency promised by AI tools processing tasks faster could logically influence the pace of the patent prosecution lifecycle itself. A quicker turnaround on initial reviews and responses might compress the overall timeframe from filing to grant or abandonment, potentially influencing the speed at which new technologies enter the public domain or are commercialized, though the ultimate impact on the rate of technological progress is complex and multifactorial.
5. AI systems are showing capabilities in dynamically aggregating and presenting information, potentially enabling the creation of personalized operational views (sometimes referred to as dashboards). These interfaces could theoretically analyze incoming tasks, real-time data flows, and project deadlines to help guide the individual reviewer in prioritizing their daily activities based on immediate needs and evolving situational context, moving away from static task lists towards more data-informed workflow management.
AI-Driven Insights: Redefining Patent Review and IP Strategy - Connecting IP planning with technical development efforts
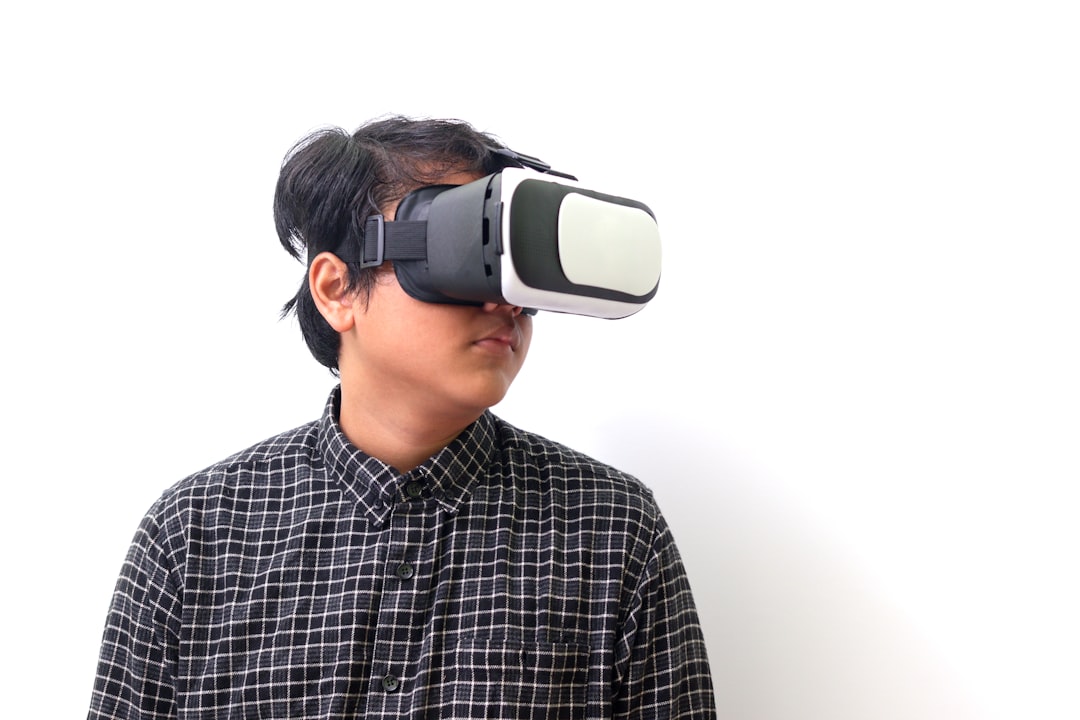
Achieving meaningful synergy between crafting intellectual property strategies and executing technical development projects has become an imperative for organizations navigating the innovation landscape. In the current environment, where intelligent systems are increasingly integrated into intellectual asset management, ensuring that R&D initiatives are guided by and, in turn, inform IP planning is essential. This closer alignment facilitates clearer decision-making early in the development cycle, potentially improving the assessment of whether novel technical outcomes are truly distinct and eligible for protection. It aims to empower technical teams with a better understanding of the existing IP environment as they invent. However, achieving this seamless connection is proving complex; simply deploying new tools doesn't automatically bridge departmental silos or guarantee that AI-generated insights are effectively translated into actionable R&D direction. Sustaining a genuinely collaborative loop requires continuous effort to ensure human experts in both IP and technical fields effectively communicate, interpret tool outputs critically, and collectively steer innovation towards strategically valuable outcomes, rather than passively accepting system suggestions. The success of future innovation pipelines hinges on how well these interdependencies are managed.
Observing the intersection where intellectual property considerations meet the messy reality of technical development efforts offers some interesting perspectives. It's not just about patenting what's built; it's increasingly about how IP insights might influence *what* gets built in the first place. Here are some thoughts from the engineering side looking into this dynamic:
1. There's a notion floating around that mapping the existing technological landscape using computational tools can effectively highlight unclaimed territory – often optimistically called "white spaces" – before significant development resources are committed. The hypothesis is that directing R&D towards these seemingly open areas, guided by this AI-assisted landscaping, might result in patent applications that face fewer obstacles from pre-existing prior art. While the goal of avoiding wasted effort is appealing, the critical question is whether these tools truly identify commercially viable or technically feasible white spaces, or simply areas where nobody has bothered to tread for legitimate technical or market reasons. Relying purely on IP data might paint an incomplete picture.
2. Integrating automated systems that scan for newly published patents with ongoing technical development seems intended to create a sort of early warning system. The idea is that if a competitor patents something relevant while you're in the middle of prototyping, the AI flags it swiftly, potentially allowing for design tweaks to avoid future conflicts. The potential speedup in reacting to external IP shifts is often touted, but how effectively technical teams can actually *incorporate* such changes mid-development is highly context-dependent and rarely as seamless as simply receiving an alert. Real engineering changes take time and resources, regardless of how fast the legal team finds the relevant prior art.
3. Promoting direct, earlier interaction between IP specialists and technical teams, perhaps facilitated by shared access to organized patent information via AI-powered platforms, is seen as a way to potentially craft stronger patent claims. The hope is that a better mutual understanding of both the technology's nuances and the legal claiming strategy can lead to claims that are more difficult for competitors to navigate around. However, attributing a specific percentage increase in "design-around difficulty" to this process seems overly precise; the strength and breadth of claims remain highly dependent on the inventive concept itself and the skill of the human drafter, not solely on the tools used or the frequency of interaction.
4. Attempts are being made to use AI algorithms to analyze historical data – like the number of times a patent is cited by later applications or correlations with reported commercial outcomes – to predict a patent's likely "strength" or potential impact. The notion is that these predictions could help inform decisions about which areas of technology to invest in or prioritize for development. While statistical patterns can sometimes offer interesting correlations, predicting complex future outcomes like commercial success or litigation resilience based primarily on document-based metrics feels speculative. Relying heavily on such predictions to guide fundamental technical strategy might overlook crucial, non-quantifiable factors.
5. There's also exploration into using AI to predict the likelihood that a given patent, either ours or a competitor's, might be involved in future assertion or litigation. By analyzing claim language, market data, and known competitor activities, these systems aim to identify high-risk IP areas. The premise is that R&D can then potentially steer clear of these predicted conflict zones, thereby reducing the likelihood of future legal disputes. While proactive risk assessment is valuable, the intricate, often unpredictable nature of litigation, driven by business strategy and legal interpretations that are difficult for algorithms to model accurately, makes the reliability of such predictions, and thus their utility for directly dictating development paths, quite uncertain.
AI-Driven Insights: Redefining Patent Review and IP Strategy - Examining the USPTO's approach to AI tools
By May 2025, the U.S. Patent and Trademark Office is visibly expanding its adoption of artificial intelligence capabilities, a move largely driven by the increasing volume of AI-related patent applications it receives. The agency has outlined a formal strategy acknowledging both the potential benefits and inherent difficulties in integrating these tools into the patent examination workflow. Part of this approach includes recent guidance clarifying how patent professionals should responsibly use AI, coupled with the assertion that current regulations are sufficient to manage risks. While the USPTO emphasizes leveraging AI to speed up and improve examination processes, questions persist regarding whether this push for efficiency might inadvertently affect the depth of analysis required for complex inventions or create an undue reliance on algorithmic outputs where human judgment is paramount. Effectively navigating this transformation demands more than just deploying technology; it necessitates a critical eye on ensuring quality remains uncompromised.
Okay, here are some observations on interesting ways the USPTO seems to be incorporating computational tools leveraging artificial intelligence into its operations, gleaned from public information and discussions as of May 2025. These points highlight specific aspects of their internal process rather than the broader strategic outcomes already discussed:
Based on various reports and agency statements, it appears the USPTO is actively deploying AI in some less obvious areas. For example, internal tools are reportedly being used to try and forecast the workload burden on individual patent examiners. The aim is to potentially allow for more dynamic allocation of applications, attempting to smooth out backlogs and perhaps match application complexity with examiner experience, although the practical effectiveness of this dynamic redistribution in truly optimizing expertise across diverse technologies is still being assessed.
There's early data suggesting that the AI systems assisting examiners in their prior art searches might be proving particularly adept at surfacing non-patent literature (NPL) – things like technical articles, standards documents, and conference papers – which are often crucial but historically harder to find systematically than patents. If this trend holds, it could subtly shift the emphasis of examination towards a more robust consideration of the technical state of the art beyond just patent documents, provided examiners have the time and training to properly evaluate the volume and diversity of NPL found.
A more experimental area involves the USPTO exploring AI-driven simulations of how claims in a patent application might be interpreted in various hypothetical legal scenarios, essentially trying to predict potential claim construction issues or vulnerabilities. The apparent idea is to use these insights, perhaps at the examination stage or even in guidance to applicants, to encourage clearer claim language from the outset, theoretically reducing downstream ambiguity or the likelihood of disputes. Whether an algorithm can reliably mimic the complexities of human legal interpretation remains a significant question mark.
Additionally, there are indications that the agency is utilizing AI algorithms to perform automated checks for internal consistency within patent applications – specifically looking for potential disconnects or lack of support between the detailed written description and the scope of the claims. Such checks could, in theory, flag issues early in the process, potentially reducing the number of office actions related to formalities or the written description requirement (35 U.S.C. § 112), thereby streamlining at least one source of delay, assuming the tools are accurate and don't generate excessive false positives.
Finally, on the procedural side, AI tools are reportedly being piloted to assist in the formal review of international patent applications as they enter the U.S. national phase. The goal here seems to be ensuring that these complex foreign-filed applications meet U.S. formal requirements more accurately before being passed on for substantive examination or transmission to other offices, potentially reducing administrative burdens and speeding up the initial processing steps for a significant portion of the USPTO's incoming applications, provided the systems can handle the variations in international filing practices.
AI-Driven Insights: Redefining Patent Review and IP Strategy - Moving past manual methods for assessing patent strength
As of May 2025, a significant evolution is underway in how the robustness and potential value of a patent are assessed. The process is increasingly moving beyond the limitations of purely manual methods, incorporating computational tools that attempt to undertake some of the initial, labor-intensive analytical steps. This marks a shift towards systems that aim not just to gather data faster, but to provide preliminary algorithmic evaluations concerning aspects of validity, scope, or comparative positioning. It signals a departure from solely human-driven review towards integrating automated methods for the initial screening and flagging of potential strengths or vulnerabilities inherent in a patent asset. A key challenge remains ensuring that these automated assists genuinely enhance the quality and depth of the final assessment, given the nuanced legal and technical interpretation required to determine a patent's true footing.
Here are some observations regarding how methods for evaluating the potential robustness or "strength" of a patent seem to be evolving, moving beyond solely manual approaches:
1. There's work being done to analyze the sheer structure and linguistic patterns within patent claims using computational methods. This isn't just checking for formality; it's attempting to identify subtle phrasing or structural choices that might correlate with how those claims are interpreted later, perhaps in a dispute, going deeper than simply looking for specific keywords or technical terms. It's almost treating legal language as data to find predictors of future interpretation.
2. Efforts are expanding in using network analysis to map the relationships *around* patents. Instead of just tallying direct citations, these tools explore connections between inventors, companies, technical areas, and even research publications that cite or are cited by patent families. The aim is to understand a patent's position within this broader ecosystem, hypothesizing that its "strength" or influence might derive from its nodal importance or bridging role between different parts of the network, not just its individual technical merit in isolation.
3. Models are being trained on historical data from patent validity challenges and litigation outcomes. Proponents suggest these machine learning approaches can predict the likelihood of a patent withstanding such scrutiny based on patterns identified in the data – potentially factors related to its content, prosecution history, or citation profile. While intriguing, the complexity of legal processes and the influence of non-quantifiable human elements make claiming definitively high accuracy in predicting real-world judicial outcomes a proposition that warrants significant, ongoing scrutiny.
4. While automated tools have helped generate technology landscape overviews, the current development appears to include capabilities for the tool itself to offer an assessment of the confidence level regarding its own findings – for instance, how certain it is about the boundaries of a technology cluster it identified or the genuine lack of prior art in a suggested "white space." This self-assessment feature, if reliable, could be valuable in tempering expectations about the AI's output, though understanding *how* this confidence score is derived is essential.
5. For these computational assessments of strength to be truly useful, the drive towards 'Explainable AI' (XAI) is critical. Simply receiving a "strength score" from an algorithm isn't particularly actionable for a human expert who needs to understand the underlying reasoning. XAI systems in this context aim to highlight *which* specific factors – perhaps certain claim terms, links in the network analysis, or patterns from the historical data – contributed most significantly to the AI's assessment, attempting to open the "black box" and provide transparent, verifiable grounds for its conclusions.
AI-Driven Insights: Redefining Patent Review and IP Strategy - Navigating questions of authorship with AI assisted inventions
The growing capacity of artificial intelligence to contribute meaningfully, and sometimes substantially, to the inventive process presents patent systems with a fundamental test: redefining who or what qualifies as an inventor. As of May 2025, the established legal consensus, rooted in crediting human ingenuity, faces pressure from scenarios where AI tools generate novel technical solutions with minimal human guidance on the specific outcome. This sparks intricate debates about whether recognition should extend beyond humans, potentially including the AI itself, the entity that trained or owns it, or the human interacting with it in some capacity. The absence of clear global uniformity on this issue necessitates careful consideration within legal frameworks to ensure they appropriately attribute inventorship, manage the rights associated with AI-generated subject matter, and critically, avoid stifling the very innovation these tools enable while upholding core IP principles.
Okay, here are five interesting points regarding the challenges of navigating questions of authorship when artificial intelligence is involved in the invention process, as observed around May 2025:
1. The established concept of legal inventorship, which traditionally requires human "conception" of the invention, is encountering significant friction. When an AI tool goes beyond simply processing data or executing pre-defined steps and appears to contribute conceptually to a novel technical solution, deciding if, how, and why that contribution should be recognized legally is a knotty problem. It highlights the difficulty in applying a human-centric legal framework to creations where the spark of innovation seems to emerge, at least partially, from an algorithmic black box.
2. Discussions are gaining ground about the possibility of formally acknowledging the role of AI in invention filings, perhaps through a designation like "AI Contributor," without necessarily granting it the full status (or legal rights) of a human inventor. This feels like a pragmatic attempt to bridge the gap between the realities of modern R&D workflows and the existing legal structure. However, from a practical standpoint, it raises questions about what information such an acknowledgment should contain to be useful – simply stating "AI was used" seems insufficient, but detailing the AI's precise role can be challenging and potentially reveal sensitive internal methodologies.
3. Determining inventorship becomes particularly complex when multiple AI systems, potentially developed by different teams or relying on different models, interact with each other and human users in the process of reaching an inventive solution. Pinpointing which algorithmic contribution, or combination of contributions, was the legally operative "driver" of the invention is proving to be a significant technical and legal puzzle. It underscores how tightly coupled the outputs of advanced AI can be, making the legal task of isolating individual "conceptions" seem increasingly artificial.
4. There's a growing emphasis, reflected in early legal interpretations, on the importance of being transparent about the use of AI tools in the invention process when filing for patent protection in certain jurisdictions. It appears that simply omitting this information, even if the AI's role isn't argued to rise to the level of inventorship, could potentially lead to questions of candor during patent prosecution. This pressure to disclose reflects an effort by the legal system to grapple with the novelty and potential opacity introduced by AI-assisted innovation methods.
5. A substantial debate is unfolding globally regarding the legal status of large datasets used to train generative or inventive AI systems. Specifically, whether these comprehensive collections of existing technical information should be considered prior art themselves when evaluating the novelty of an AI-assisted invention derived from them. If a model trained on publicly available technical literature generates a novel concept, can that concept truly be considered inventive over the aggregate knowledge contained within its training data? How this is resolved will significantly influence the patentability landscape for AI-driven outputs.
More Posts from patentreviewpro.com: