7 Ways AI is Revolutionizing Modular Construction Management in 2025
7 Ways AI is Revolutionizing Modular Construction Management in 2025 - Automated Quality Control Through Computer Vision Reduces Inspection Time By 40%
Automated quality control using computer vision is significantly changing how inspections are done, cutting down inspection times by up to 40%. This speed-up allows for quicker identification of problems during manufacturing, potentially improving overall product quality. In situations where finding enough skilled workers is an issue, AI-powered inspections offer a way to maintain standards and scale up production. Computer vision combines the benefits of automated processes with the flexibility of human inspection. This helps to address the intricate requirements of today's manufacturing and construction environments. AI technology in inspections is seeing use in different sectors, aiding quality assurance across the board.
Analysis suggests that using computer vision for quality checks in modular construction cuts inspection time by roughly 40%. It’s argued these systems process images at high speeds, swiftly flagging defects, far surpassing human capability. Where a person might take about five seconds to examine a part, such automated systems are described as completing the same job in fractions of a second - reportedly under 200 milliseconds. Furthermore, these machine learning-driven setups are said to refine their accuracy with each inspection, lowering the likelihood of false defect alerts. Advocates are asserting these systems achieve upwards of 90% accuracy, rivaling and sometimes outperforming human inspectors. These technologies supposedly handle varying light and material types without error – conditions claimed to be problematic for humans. It's stated that cost savings of up to 30% are possible when deploying these systems making them economical. Real-time data from high speed processing means faster decisions during production. Consistency is another perceived benefit, reducing variation due to inspector fatigue and lapses in attention. These systems are also capable of checking for correct dimensions, not just visual flaws, supposedly guaranteeing standards are met. Thus, this incorporation could mean improvements in product quality as a whole and better long term performance of finished builds.
7 Ways AI is Revolutionizing Modular Construction Management in 2025 - Digital Twin Integration Powers Real Time Supply Chain Management
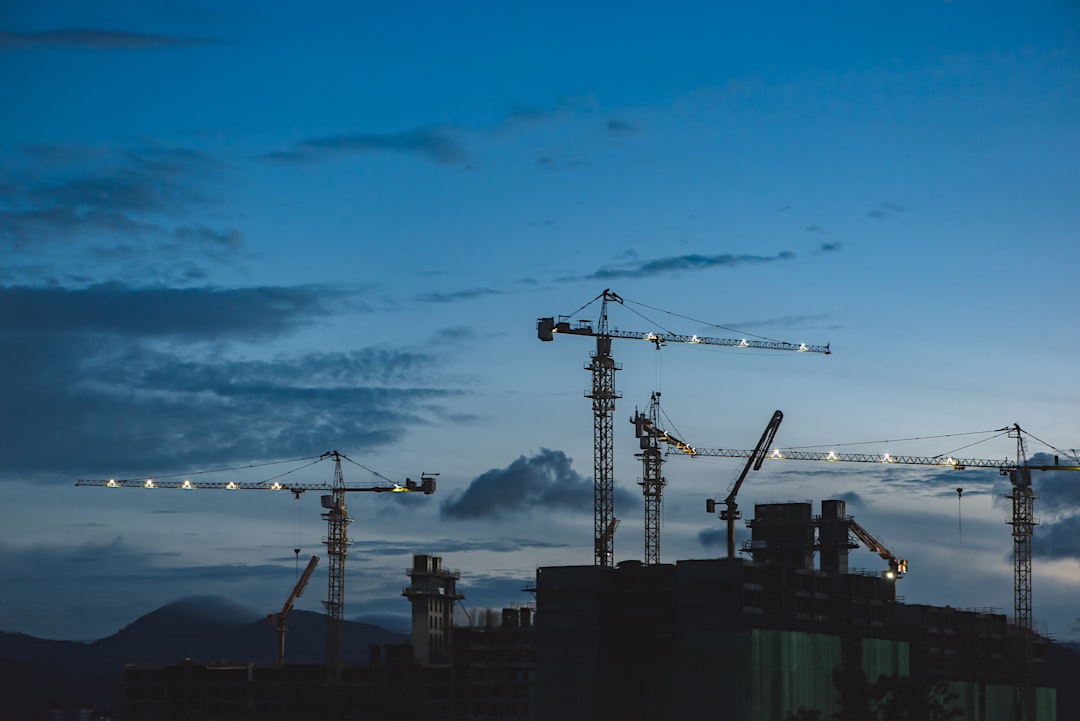
Digital twin integration in supply chain management is transforming how companies operate by providing real-time insights that enhance operational efficiency and risk mitigation. By creating virtual replicas of supply chain processes, organizations can monitor their systems dynamically, allowing for precise scenario testing and decision-making based on live data. This shift enables not just better management but also prediction of future trends, supporting a more proactive strategy. Though the capabilities are vast, the challenges in data integration and management still slow the process. The move towards AI-driven digital twins allows for better disruption response. Supply chain management transitions from older methods towards a more in-depth understanding of performance. Businesses are adopting it to remain competitive. But, until the integration challenges are addressed, this technology won't be as useful as it could be.
Digital twins provide real-time data feeds from various points, enabling supply chain managers to create dynamic simulations that reflect current operational statuses. Updates can occur as rapidly as once per second, leading to immediate adjustments and decision-making—a step beyond static modeling. These tools have also shown the potential to reduce downtime by up to 30%, by forecasting disruptions and suggesting actions by blending historical data with current analytics. This predictive ability aims to reduce delays, not eliminate them completely. Inventory optimization is another reported benefit in manufacturing contexts. Digital twins allow better matching of stock with demand, potentially reducing inventory carrying costs by about 20% and maintain production schedules. Process efficiency may also see a potential 25% improvement, it's claimed. This occurs due to higher visibility of the whole supply chain, which leads to swifter anomaly detection. The technology also appears to facilitate collaboration by allowing access and interaction with the same virtual model across various stakeholders, potentially increasing communication efficiency by roughly 40%.
Real-time information provided by digital twins is altering the speed of decision-making in supply chains; some using the technology have claimed reduced lead times by 50%, due to better forecasting. AI integration with digital twins could even lead to fully autonomous decision-making systems where parameters adjust themselves. These claims, however are only theoretical. In situations of supply chain disruption, it is stated that companies using digital twins may recover quicker. Simulation capabilities allow for quick testing of back-up plans. Since data is continuously fed into digital twins, their algorithms can auto-adjust. This is touted to be essential in volatile conditions where other models may fail. It is also suggested that digital twin integration enables the creation of a digital supply chain with complete organizational visibility. This claimed holistic view could encourage transparency and accountability, changing decision-making processes to rely more on data than prior methods.
7 Ways AI is Revolutionizing Modular Construction Management in 2025 - Machine Learning Algorithms Cut Material Waste By 35% In Prefab Assembly
Machine learning algorithms are showing strong results in cutting material waste in prefab assembly, with reported reductions reaching 35%. Given the construction industry's heavy consumption of raw materials and its contribution to significant waste, these advancements address an essential problem. Projections indicate a substantial rise in global waste generation by 2050, from 201 billion to 340 billion tonnes, underscoring the urgency to develop more efficient and environmentally conscious processes. By refining designs and improving inventory accuracy, machine learning can not only minimize waste but also potentially enhance the handling of materials. This development could be important in promoting more sustainable practices and reducing the industry's overall environmental impact, assuming claims prove true.
Within prefab assembly, it's being explored how machine learning algorithms can sift through past waste data to spot causes of material inefficiencies, potentially leading to a 35% waste reduction - showing an intriguing intersection of data work and real-world use.
By employing methods like reinforcement learning, these systems can test various design and build options to discover the configurations that result in the least material usage, demonstrating a novel approach to refining modular construction production.
Predictive analytics algorithms can forecast which materials might end up as surplus, based on project specifications. This enables teams to make better decisions on inventory, potentially reducing excess waste.
Machine learning models, using methods of clustering, can categorize materials and parts to pinpoint the items that most often lead to waste. This allows for a more targeted approach to trimming excess material usage in later builds.
Using machine learning in prefab assembly may reduce waste but also cut down on labor hours spent handling excess materials. This could mean better operations and speedier project delivery.
These machine learning models can adapt to changes in design or materials, constantly fine-tuning their advice on resource use, basically making ongoing project management into an iterative process.
Some manufacturers say that machine learning algorithms can accurately predict expected waste for each phase of a project, with nearly 90% accuracy. This could dramatically improve planning and budget accuracy.
These algorithms can analyze real-time data from the production lines to fine-tune material usage as it occurs. This highlights an innovative ability of machine learning to rapidly respond to on-site issues.
Incorporating machine learning into assembly allows for advanced math to model and solve complex problems related to stress and load distribution within materials. This can ensure materials are being used optimally and are the appropriate quantity needed.
It's worth noting, reducing waste by 35% may have ripple effects across the entire supply chain, leading to cost savings not just in raw material purchasing, but also in shipping logistics; which highlights potential widespread financial advantages of better waste management strategies.
7 Ways AI is Revolutionizing Modular Construction Management in 2025 - Robotic Process Automation Takes Over 80% Of Documentation Tasks
Robotic Process Automation (RPA) is now substantially changing the management of paperwork in construction. It's being said that up to 80% of routine documentation can be handled by RPA systems, freeing up human workers to focus on more critical tasks. This shift aims to improve both efficiency and accuracy by reducing errors made by people. When used with AI, RPA can also process unstructured information and handle complex tasks that would traditionally require human input. While many see these changes as positive, they're not yet fully taken advantage of, mainly due to insufficient understanding of the tech and its benefits.
Robotic Process Automation Takes Over 80% Of Documentation Tasks
It appears Robotic Process Automation (RPA) is now capable of performing basic documentation tasks at speeds beyond what humans can do, some claim processing tasks in mere milliseconds compared to the minutes a human worker might need. This increased pace seems useful, but more real-world data is needed to fully measure its impact.
RPA is reported to operate with strong reliability, with some documentation tasks allegedly achieving over 99% accuracy. While this might suggest fewer errors, it’s necessary to investigate how such accuracy affects the real-world issues with complex document trails in construction.
Furthermore, it is claimed that these systems can function non-stop, allowing documentation processes to continue around the clock, without interruption, potentially speeding up work flow; it is unclear if this nonstop operation will present new problems in how projects are organized.
The incorporation of RPA into construction documentation has been stated to produce measurable efficiency gains, with reports indicating a reduction of record management times by a claimed 80%. It might be crucial to see these reports are transparent to verify the real value provided by such systems.
RPA also seems to have the ability to easily adjust to varied workloads, potentially giving companies flexibility to handle unexpected jumps in documentation without the standard problems of hiring more staff, this appears promising, but should be tested in various construction contexts to see how well it holds up.
Human documentation errors can often come from problems such as fatigue or lack of focus; it's clear that RPA eliminates these issues, but it would be beneficial to verify whether such systems might introduce new types of documentation issues that aren’t seen in human work.
RPA seems to be useful in enhancing compliance and audit preparation, as these systems can track changes, create logs, and keep records better than manual practices. Such capabilities could be essential given the compliance demands in large construction projects.
RPA allows easier connections with current tech setups, allegedly automatically pulling data from documents, forms, and emails. It will be beneficial to see if these integrations will simplify the processing of multiple data streams commonly seen in modular construction.
Cost savings from RPA implementation appear to move beyond simple labor costs, since faster processing times and fewer mistakes could lower operating costs. These assertions should be explored carefully to see the long-term effects.
With natural language processing, RPA could potentially learn from prior documentation patterns, and adapt workflows. These claims should be tested to see how effectively RPA handles the varying language and terminologies used across construction documentation, which has many exceptions.
7 Ways AI is Revolutionizing Modular Construction Management in 2025 - Predictive Analytics Prevent Construction Delays Through Weather Pattern Analysis
Predictive analytics is emerging as a key strategy for preventing construction delays, focusing on detailed analysis of weather patterns. This approach changes how project managers plan and assign resources. AI systems, processing both past and current weather data, aim to foresee likely disruptions and enable preventative actions to maintain project timelines. The goal is improved decision-making, potentially leading to better cost accuracy and greater efficiency. Despite these advances, issues like the complexity of construction projects and the unpredictable nature of the environment still pose challenges. For the construction sector, adopting these tools means carefully balancing technology with the real-world conditions of each site.
Predictive analytics offers a way to use past weather data to foresee disruptions, which may improve scheduling accuracy by up to 25%. This lets project managers change timelines early based on forecasts, lessening the risk of expensive delays. Certain predictive models can analyze things like the El Niño and La Niña patterns, which have been shown to be a factor in delays. This is important when trying to reduce risks connected to changing environments. More advanced models can also look at microclimates by considering local land shapes and city heat islands, which affect weather conditions on building sites. This detailed level of analysis helps when deciding when to move workers and equipment.
By employing predictive analytics, building projects might be able to reduce idle time caused by bad weather by around 30%. This benefit seems to come from smarter work schedules that are in line with expected weather patterns and not just averages. These models can also show how weather impacts materials, recommending changes in material choices and handling to maintain quality under varying conditions. This could cut down on rework that happens because of storage or installation issues. Such analytical systems can provide real-time weather updates, alerting teams about incoming storms or temperature drops. This speed can help lessen on-site accidents and promote overall safety. Some predictive solutions also integrate map data, considering geographical risks, like flood zones or high wind areas. This type of understanding may be useful when selecting a construction site and its overall planning by removing difficult areas.
Additionally, predictive weather analytics can aid in optimizing energy usage for temporary site buildings. By tracking weather trends, it might be possible to design for minimized energy usage during bad weather, like excessive heat or heavy rain. Machine learning can improve the accuracy of weather predictions over time. By always learning from new information, these systems could refine their forecasting, possibly making them more reliable. Predictive weather analytics can also help with communicating with stakeholders. By providing reliable forecasts and risk assessments, project managers could make communication better with clients, making sure that everyone is informed.
7 Ways AI is Revolutionizing Modular Construction Management in 2025 - Smart Sensors Track Module Transportation With 99% Location Accuracy
Smart sensors are proving very useful for tracking module transportation, with location accuracy reported to be over 99%. This level of precision is intended to make logistics run more smoothly and improve overall efficiency in modular construction. These sensors can help ensure that components get where they need to be, without undue delays. When combined with artificial intelligence, these smart sensor systems may give project managers tools to optimize routes and schedules using real-time information, supposedly allowing for better resource management. While these technologies have many possible benefits, there are concerns about how secure the data is, and how these systems would operate if they broke down. It remains important to fully test their reliability and be prepared with backup plans when the industry adds these technologies into project management.
Smart sensors are now used in module transportation, reportedly with location accuracy exceeding 99%. These devices offer a constant stream of data; things like module speed, temperature, and humidity, reportedly capturing hundreds of data points per second. This wealth of real-time information seems beneficial for managing the moving process; the sensors may even help predict future conditions that could negatively impact operations, but this needs further testing. These sensor systems use a mix of location technologies like GPS, RFID, and inertial units. It's been suggested that even in dense areas, like forests or cities, pinpoint accuracy is maintained, but this needs validation via independent research.
These smart systems also construct detailed maps of all transit routes. Stored historical data could allow for improved route selection in the future and might cut down overall time spent in transit. Claims are being made of up to 20% reduction in travel time, but more information is needed to verify these figures, especially in unpredictable conditions. It is claimed that the data collected can even be used by machine learning, which could improve efficiency over time; the tech can change routes based on past data and could reduce transportation costs by improving fuel efficiency, with some projections suggesting up to 15% fuel savings. It's being suggested that improved location tracking may reduce inventory discrepancies by up to 30%, thus making sure everything arrives when it is supposed to. It seems the tech may be most useful in situations with very complex transportation issues.
With on-board sensors to monitor conditions, warnings can be sent if the environment gets too hot or humid for delicate materials. If such claims prove true, this would reduce material quality problems, possibly by a full 25%. Yet, when deploying sensors across many vehicles the resulting data can easily become unmanageable, especially without solid data management software, so care needs to be taken. Also, the cost of deploying these smart sensors is a barrier for some companies. However, it is argued that the savings resulting from reduced delays and reduced material loss outweigh these expenses, and could potentially save as much as 20% on transportation, if such data claims are to be believed.
Moreover, these smart sensors appear to enable direct communication between logistics managers and on-site teams. If these claims hold up it could lead to improved coordination, as well as quicker decision making, with time savings of up to 50% in theory. The information gathered by the sensors may also predict when a vehicle will require servicing based on usage, and prevent breakdowns. If accurate, this might cut downtime and increase vehicle life by about 40%, though, without independent analysis of these claims it's hard to fully accept them.
7 Ways AI is Revolutionizing Modular Construction Management in 2025 - Natural Language Processing Streamlines Building Code Compliance Reviews
Natural Language Processing (NLP) is making significant changes to how building code compliance is handled in modular construction. This technology works by changing complex legal jargon into plain, understandable language. It is supposed to speed up the contract review process and pinpoint important details and potential issues which might help to better stick to building regulations. Furthermore, NLP has the potential to automate building code checks, which could make land development and permit processes faster. Although these improvements seem to offer better efficiency and safety in construction, there is a valid concern about how well AI can actually grasp all the subtle parts of regulatory language. Nevertheless, NLP shows that there is potential for smarter, more efficient management in construction projects.
Natural Language Processing (NLP) doesn't just grasp words; it analyzes context. This means NLP can streamline building code reviews by picking out relevant rules from huge piles of documents. This could potentially cut review times by up to half. More advanced NLP systems try to understand what the code actually means, going beyond just the surface-level words. This type of analysis can spot things like vagueness or contradictions in paperwork. By doing this, such systems can lead to less confusion and fewer project delays that cost money. When dealing with various building code docs, NLP can organize things by location, building type, or materials. This enables builders to get the right regulations quickly, which matters given that building codes are often tricky and very local. Using NLP also automates taking crucial compliance data from scanned pages, eliminating the need to enter everything by hand, speeding up review and lowering the chances of human mistakes.
NLP could help project managers communicate with regulators by creating clear questions and answers, which could lead to better back and forth and speed up how compliance issues are solved. NLP models can adapt and learn from how compliance reviews went in the past. This helps them better understand which code interpretations lead to approvals, helping improve predictions on future work. Construction companies can use NLP to check real-time updates on building code changes; as NLP analyzes large datasets. This might reduce compliance risks, making sure that projects follow the newest standards. Some researchers propose that NLP use in compliance could spot patterns of non-compliance, which would help shape construction practices and team training, creating a feedback loop of progress.
Instead of simply pointing out potential issues, newer NLP systems could give actual solutions or modifications, based on previous approvals. This might be valuable by offering real guidance as situations come up. Introducing NLP tools may alter regulatory inspections as quick verification of compliance could lessen the need for long manual checks. This might change how regulators do their work and encourage more cooperation between them and construction firms.
More Posts from patentreviewpro.com: